Deep Graph Unfolding for Beamforming in MU-MIMO Interference Networks
CoRR(2023)
摘要
We develop an efficient and near-optimal solution for beamforming in multi-user multiple-input-multiple-output single-hop wireless ad-hoc interference networks. Inspired by the weighted minimum mean squared error (WMMSE) method, a classical approach to solving this problem, and the principle of algorithm unfolding, we present unfolded WMMSE (UWMMSE) for MU-MIMO. This method learns a parameterized functional transformation of key WMMSE parameters using graph neural networks (GNNs), where the channel and interference components of a wireless network constitute the underlying graph. These GNNs are trained through gradient descent on a network utility metric using multiple instances of the beamforming problem. Comprehensive experimental analyses illustrate the superiority of UWMMSE over the classical WMMSE and state-of-the-art learning-based methods in terms of performance, generalizability, and robustness.
更多查看译文
关键词
Beamforming,WMMSE,algorithm unfolding,complex-valued graph neural networks
AI 理解论文
溯源树
样例
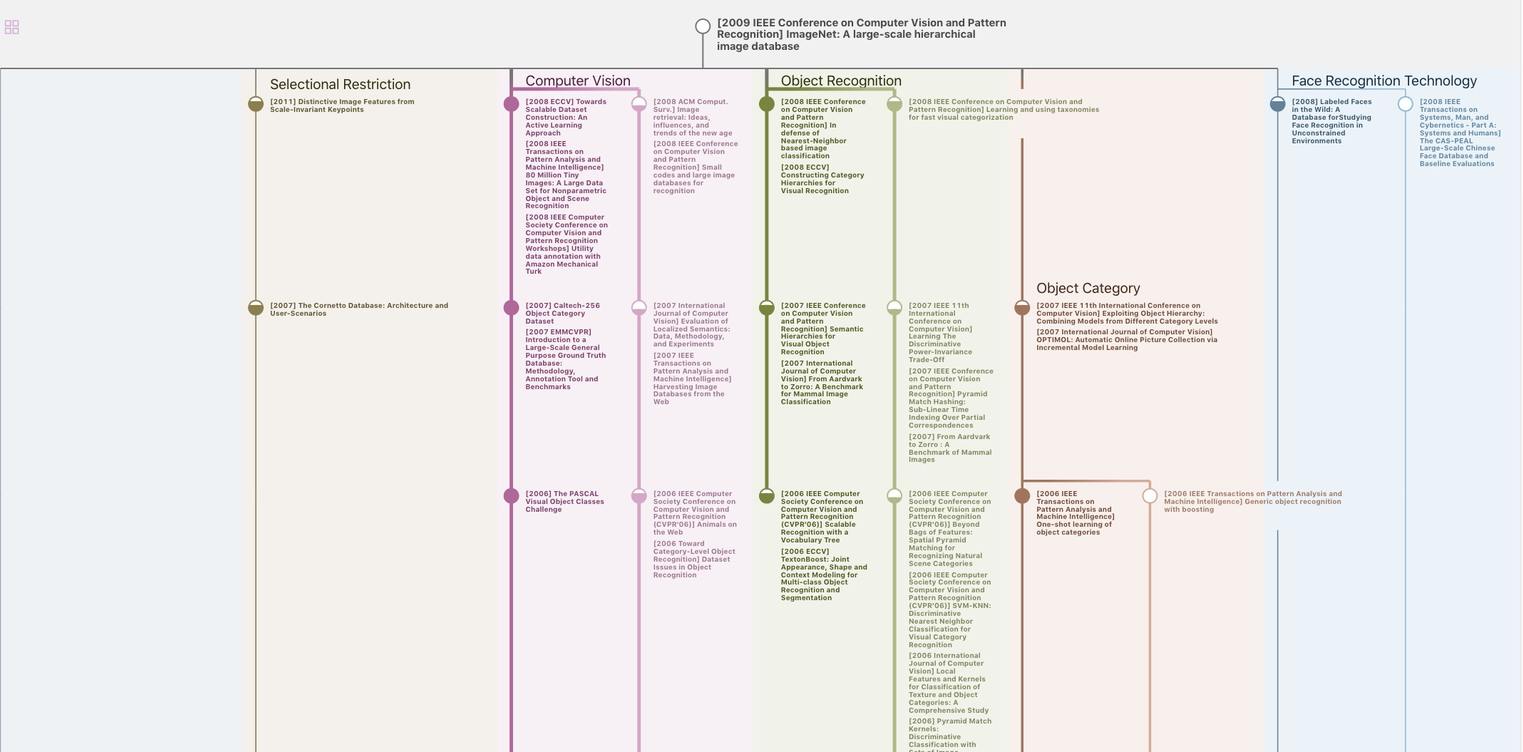
生成溯源树,研究论文发展脉络
Chat Paper
正在生成论文摘要