On the Sample Complexity of the Linear Quadratic Gaussian Regulator
2023 62ND IEEE CONFERENCE ON DECISION AND CONTROL, CDC(2023)
摘要
In this paper we provide direct data-driven expressions for the Linear Quadratic Regulator (LQR), the Kalman filter, and the Linear Quadratic Gaussian (LQG) controller using a finite dataset of noisy input, state, and output trajectories. We show that our data-driven expressions are consistent, since they converge as the number of experimental trajectories increases, we characterize their convergence rate, and we quantify their error as a function of the system and data properties. These results complement the body of literature on data-driven control and finite-sample analysis, and they provide new ways to solve canonical control and estimation problems that do not assume, nor require the estimation of, a model of the system and noise and do not rely on solving implicit equations.
更多查看译文
AI 理解论文
溯源树
样例
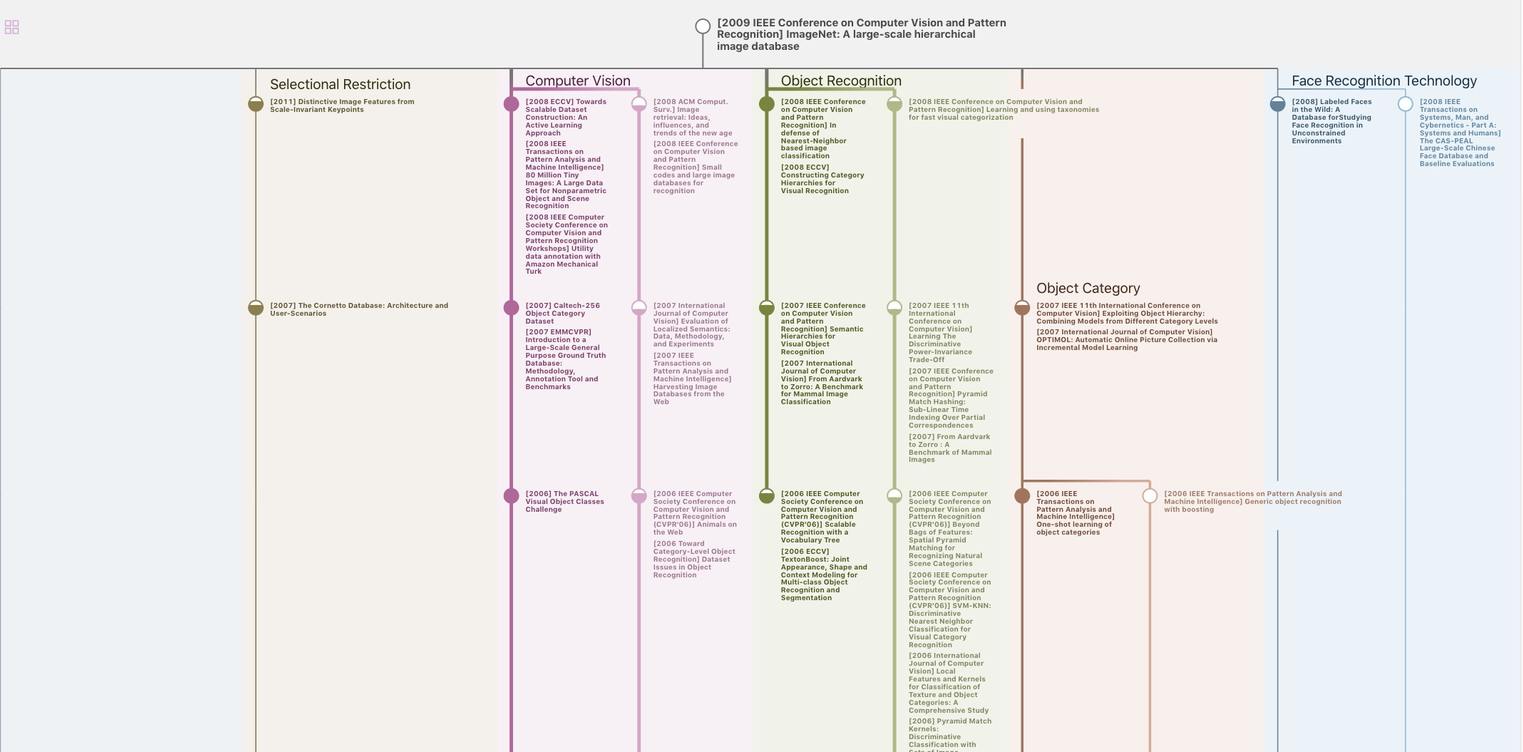
生成溯源树,研究论文发展脉络
Chat Paper
正在生成论文摘要