Secure Federated Learning against Model Poisoning Attacks via Client Filtering
CoRR(2023)
摘要
Given the distributed nature, detecting and defending against the backdoor attack under federated learning (FL) systems is challenging. In this paper, we observe that the cosine similarity of the last layer's weight between the global model and each local update could be used effectively as an indicator of malicious model updates. Therefore, we propose CosDefense, a cosine-similarity-based attacker detection algorithm. Specifically, under CosDefense, the server calculates the cosine similarity score of the last layer's weight between the global model and each client update, labels malicious clients whose score is much higher than the average, and filters them out of the model aggregation in each round. Compared to existing defense schemes, CosDefense does not require any extra information besides the received model updates to operate and is compatible with client sampling. Experiment results on three real-world datasets demonstrate that CosDefense could provide robust performance under the state-of-the-art FL poisoning attack.
更多查看译文
关键词
federated learning,model poisoning attacks,client
AI 理解论文
溯源树
样例
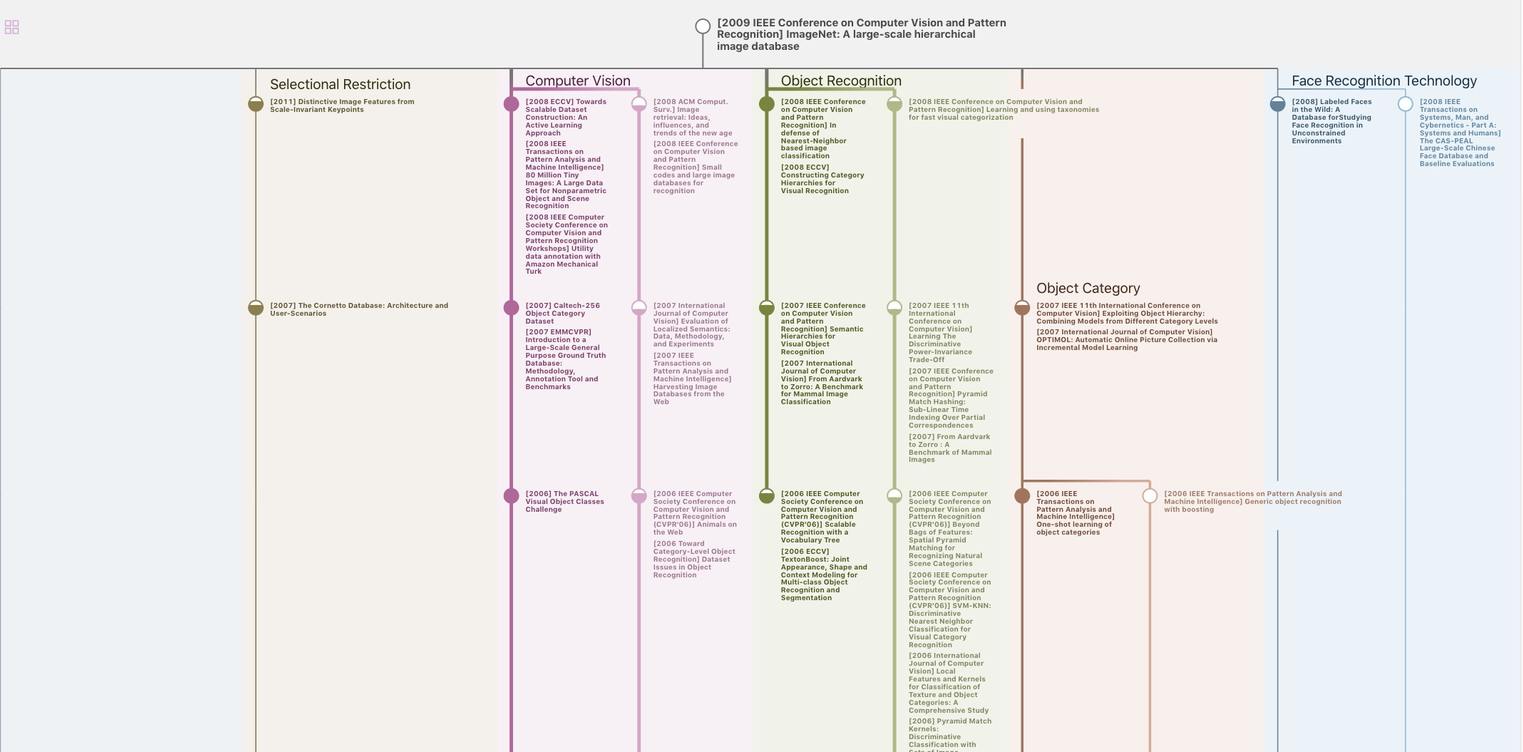
生成溯源树,研究论文发展脉络
Chat Paper
正在生成论文摘要