E($3$) Equivariant Graph Neural Networks for Particle-Based Fluid Mechanics
CoRR(2023)
摘要
We contribute to the vastly growing field of machine learning for engineering systems by demonstrating that equivariant graph neural networks have the potential to learn more accurate dynamic-interaction models than their non-equivariant counterparts. We benchmark two well-studied fluid flow systems, namely the 3D decaying Taylor-Green vortex and the 3D reverse Poiseuille flow, and compare equivariant graph neural networks to their non-equivariant counterparts on different performance measures, such as kinetic energy or Sinkhorn distance. Such measures are typically used in engineering to validate numerical solvers. Our main findings are that while being rather slow to train and evaluate, equivariant models learn more physically accurate interactions. This indicates opportunities for future work towards coarse-grained models for turbulent flows, and generalization across system dynamics and parameters.
更多查看译文
关键词
equivariant graph neural networks,fluid
mechanics,neural networks,particle-based
AI 理解论文
溯源树
样例
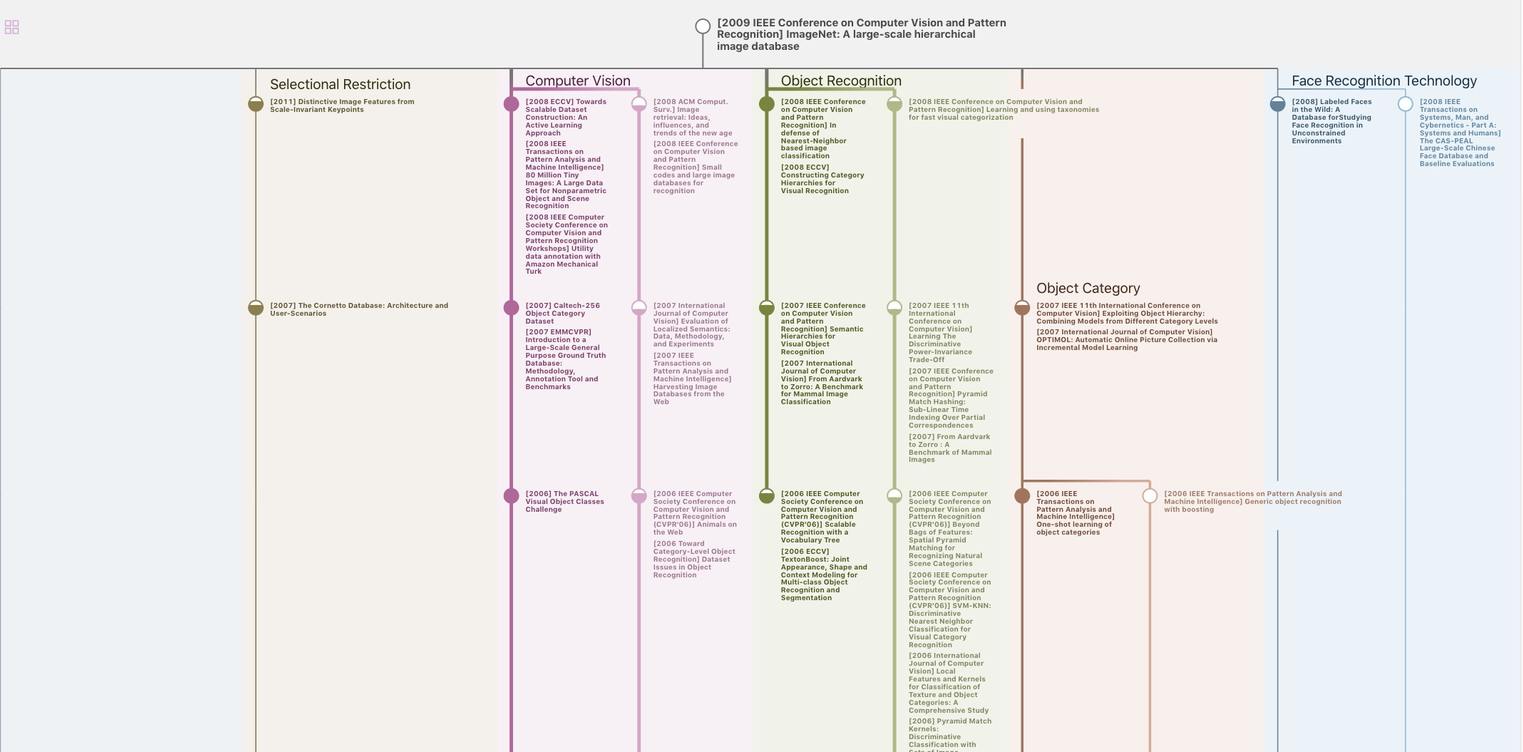
生成溯源树,研究论文发展脉络
Chat Paper
正在生成论文摘要