To be Robust and to be Fair: Aligning Fairness with Robustness
CoRR(2023)
摘要
Adversarial training has been shown to be reliable in improving robustness against adversarial samples. However, the problem of adversarial training in terms of fairness has not yet been properly studied, and the relationship between fairness and accuracy attack still remains unclear. Can we simultaneously improve robustness w.r.t. both fairness and accuracy? To tackle this topic, in this paper, we study the problem of adversarial training and adversarial attack w.r.t. both metrics. We propose a unified structure for fairness attack which brings together common notions in group fairness, and we theoretically prove the equivalence of fairness attack against different notions. Moreover, we show the alignment of fairness and accuracy attack, and theoretically demonstrate that robustness w.r.t. one metric benefits from robustness w.r.t. the other metric. Our study suggests a novel way to unify adversarial training and attack w.r.t. fairness and accuracy, and experimental results show that our proposed method achieves better performance in terms of robustness w.r.t. both metrics.
更多查看译文
关键词
fairness,robustness
AI 理解论文
溯源树
样例
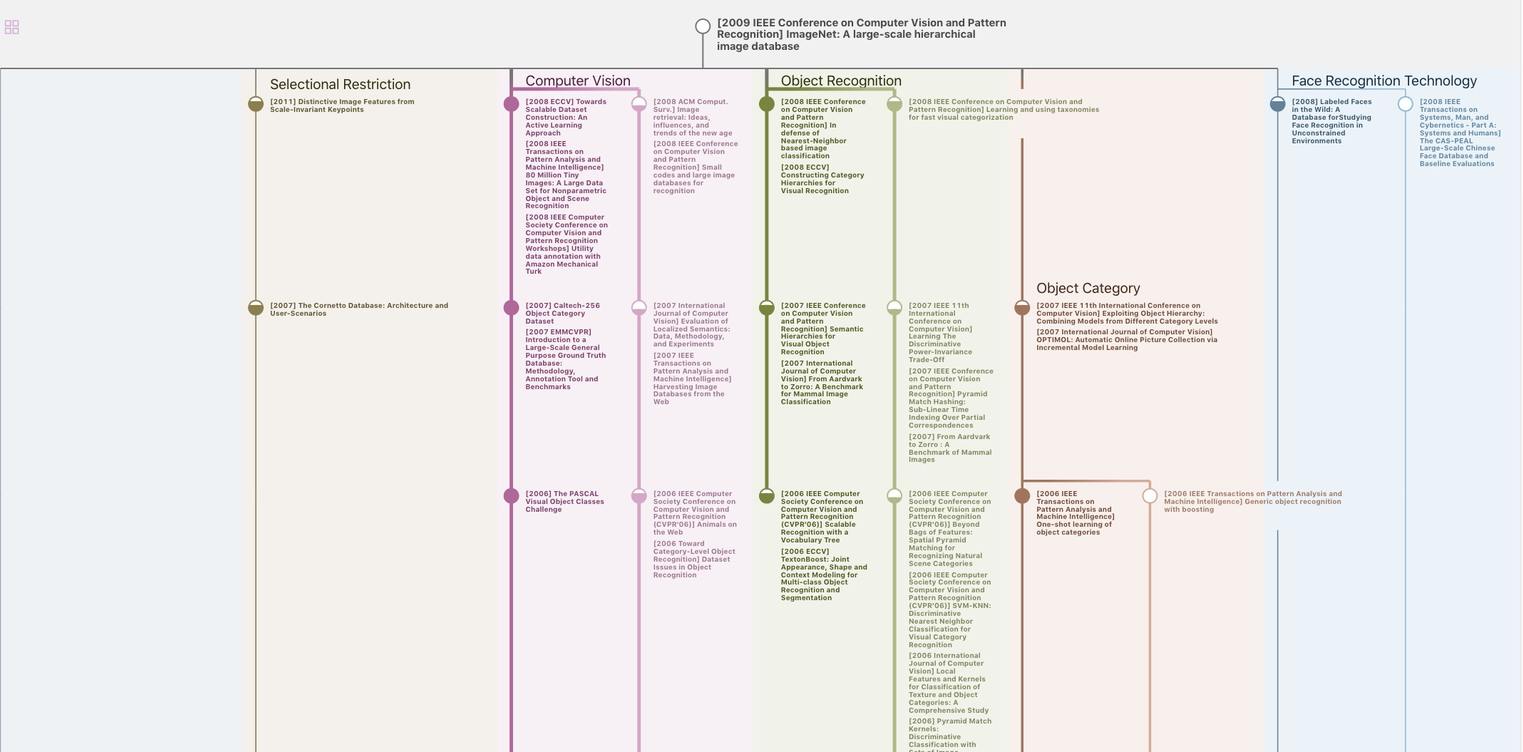
生成溯源树,研究论文发展脉络
Chat Paper
正在生成论文摘要