Data augmentation-based statistical inference of diffusion processes
CHAOS(2023)
摘要
The identification of diffusion processes is challenging for many real-world systems with sparsely sampled observation data. In this work, we propose a data augmentation-based sparse Bayesian learning method to identify a class of diffusion processes from sparsely sampled data. We impute latent unsampled diffusion paths between adjacent observations and construct a candidate model for the diffusion processes with the sparsity-inducing prior on model parameters. Given the augmented data and candidate model, we investigate the full joint posterior distribution of all the parameters and latent diffusion paths under a Bayesian learning framework. We then design a Markov chain Monte Carlo sampler with non-degenerate acceptance probability on system dimension to draw samples from the posterior distribution to estimate the parameters and latent diffusion paths. Particularly, the proposed method can handle sparse data that are regularly or irregularly sampled in time. Simulations on the well-known Langevin equation, homogeneous diffusion in a symmetric double-well potential, and stochastic Lotka-Volterra equation demonstrate the effectiveness and considerable accuracy of the proposed method.
更多查看译文
关键词
diffusion,statistical inference,data,augmentation-based
AI 理解论文
溯源树
样例
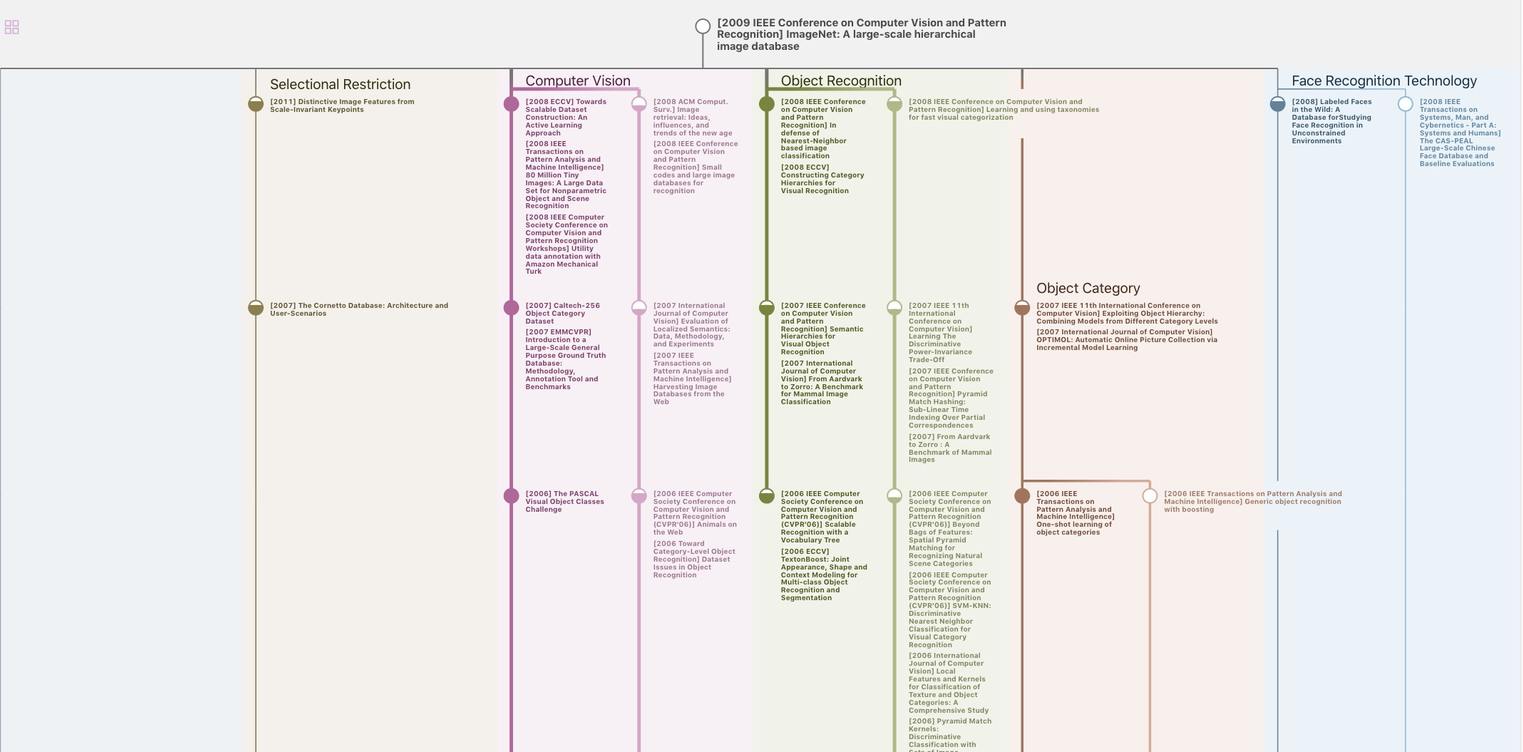
生成溯源树,研究论文发展脉络
Chat Paper
正在生成论文摘要