Impact of different classification schemes on discrimination of proteins with noise-contaminated spectra using laboratory-measured fluorescence data
Spectrochimica acta. Part A, Molecular and biomolecular spectroscopy(2023)
摘要
Biological agents are important to detect and identify with respect to environmental contamination and public health. Noise contamination in fluorescent spectra is one of the contributors to the uncertainties of identification. In order to investigate the noise-tolerant capability provided by laboratory-measured excitation -emission matrix (EEM) fluorescence spectra that are used as a database, fluorescence properties of four proteinaceous biotoxin samples and ten harmless protein samples were characterized by EEM fluorescence spectra, and the predicting performance of models trained by laboratory-measured fluorescence data was tested and verified from validation data with noise-contaminated spectra. By means of peak signal of noise (PSNR) as an indicator of noise levels, the potential impact of noise contaminations on the characterization and discrimination of these samples was evaluated quantitatively. Different classification schemes utilizing multivariate analysis techniques of Principal Component Analysis (PCA), Random Forest (RF), and Multi -layer Perceptron (MPL) coupled with feature descriptors of differential transform (DT), Fourier transform (FT) and wavelet transform (WT) were conducted under different PSNR values. We systematically analyzed the performance of classification schemes by the case study at 20 PSNR and by statistical analysis from 1-100 PSNR. The results show that the spectral features with EEM-WT decreased the demanding number of input variables while retaining high performances in sample classification. The spectral features with EEM-FT presented the worst performance although having the largest number of features. The distributions of feature importance and contribution were found sensitive to noise contaminations. The classification scheme of PCA prior to MPL with EEM-WT as input presented an improvement in lower PSNR. These results indicate that robust features extracted by corresponding techniques are critical to enhancing the spectral differentiation capabilities among these samples and play an important role in eliminating the noise effect. The study of classification schemes for discriminating protein samples with noise-contaminated spectra presents tremendous potential for future developments in the rapid detection and identification of proteinaceous biotoxins based on three-dimensional fluorescence spectrometry.
更多查看译文
关键词
Three-dimensional fluorescence spectrum,Protein,Feature transformation,Multivariate analysis,Classification scheme
AI 理解论文
溯源树
样例
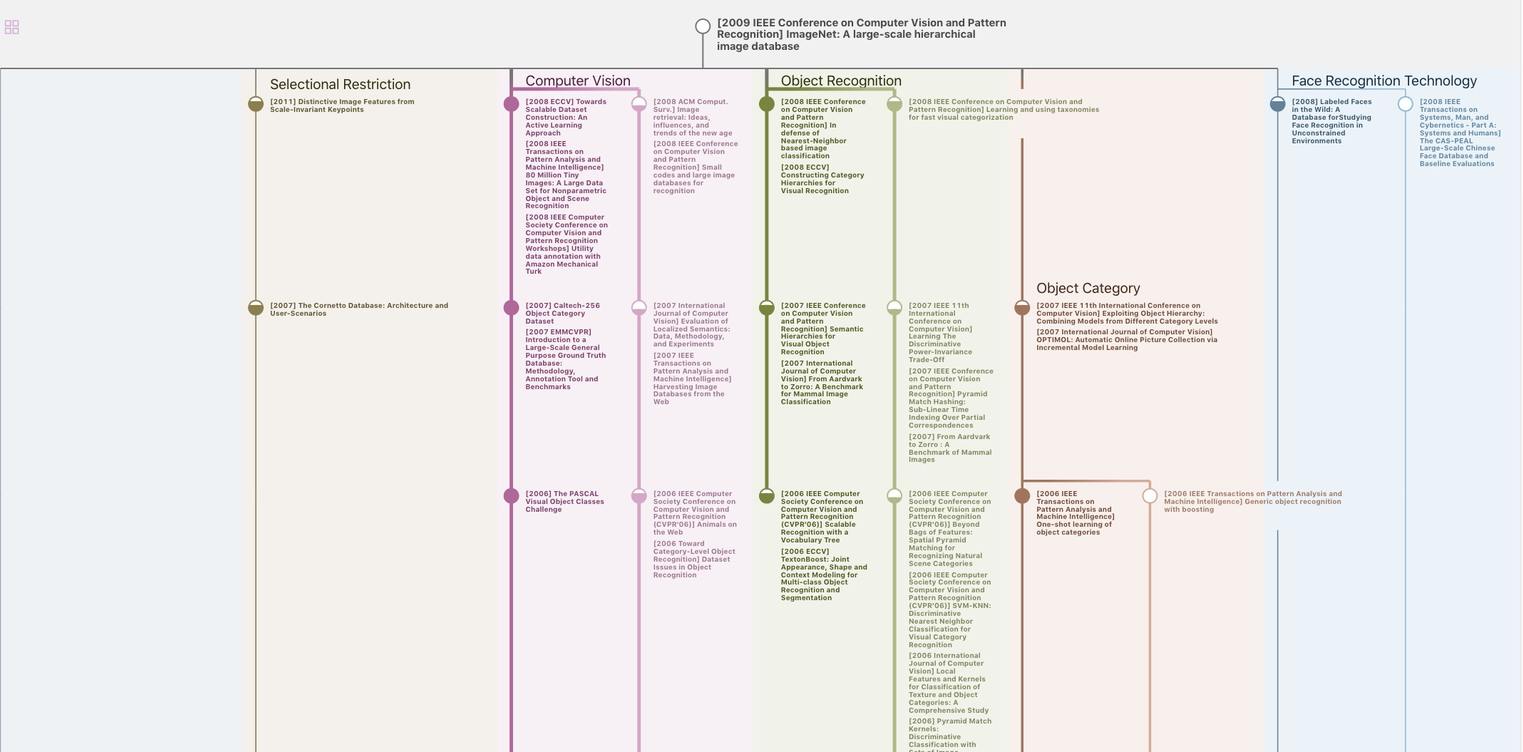
生成溯源树,研究论文发展脉络
Chat Paper
正在生成论文摘要