Learning from real world data about combinatorial treatment selection for COVID-19.
Frontiers in artificial intelligence(2023)
摘要
COVID-19 is an unprecedented global pandemic with a serious negative impact on virtually every part of the world. Although much progress has been made in preventing and treating the disease, much remains to be learned about how best to treat the disease while considering patient and disease characteristics. This paper reports a case study of combinatorial treatment selection for COVID-19 based on real-world data from a large hospital in Southern China. In this observational study, 417 confirmed COVID-19 patients were treated with various combinations of drugs and followed for four weeks after discharge (or until death). Treatment failure is defined as death during hospitalization or recurrence of COVID-19 within four weeks of discharge. Using a virtual multiple matching method to adjust for confounding, we estimate and compare the failure rates of different combinatorial treatments, both in the whole study population and in subpopulations defined by baseline characteristics. Our analysis reveals that treatment effects are substantial and heterogeneous, and that the optimal combinatorial treatment may depend on baseline age, systolic blood pressure, and c-reactive protein level. Using these three variables to stratify the study population leads to a stratified treatment strategy that involves several different combinations of drugs (for patients in different strata). Our findings are exploratory and require further validation.
更多查看译文
关键词
COVID-19,G-computation,multiple comparisons with the best,subgroup analysis,virtual multiple matching
AI 理解论文
溯源树
样例
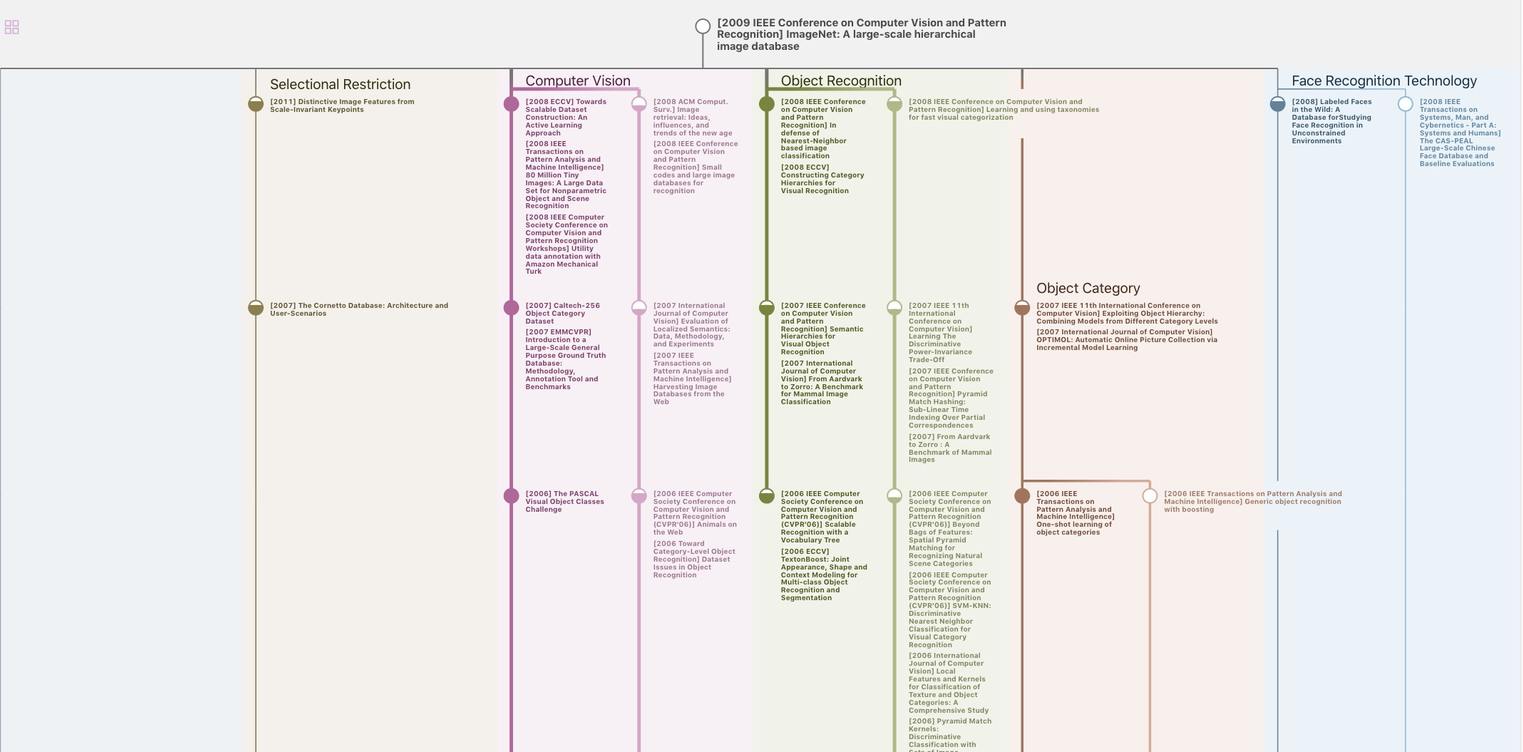
生成溯源树,研究论文发展脉络
Chat Paper
正在生成论文摘要