Unsupervised crack detection on complex stone masonry surfaces
CoRR(2023)
摘要
Computer vision for detecting building pathologies has interested researchers for quite some time. Vision-based crack detection is a non-destructive assessment technique, which can be useful especially for Cultural Heritage (CH) where strict regulations apply and, even simple, interventions are not permitted. Recently, shallow and deep machine learning architectures applied on various types of imagery are gaining ground. In this article a crack detection methodology for stone masonry walls is presented. In the proposed approach, crack detection is approached as an unsupervised anomaly detection problem on RGB (Red Green Blue) image patches. Towards this direction, some of the most popular state of the art CNN (Convolutional Neural Network) architectures are deployed and modified to binary classify the images or image patches by predicting a specific class for the tested imagery; 'Crack' or 'No crack', and detect and localize those cracks on the RGB imagery with high accuracy. Testing of the model was performed on various test sites and random images retrieved from the internet and collected by the authors and results suggested the high performance of specific networks compared to the rest, considering also the small numbers of epochs required for training. Those results met the accuracy delivered by more complex and computationally heavy approaches, requiring a large amount of data for training. Source code is available on GitHub https://github.com/pagraf/Crack-detection while datasets are available on Zenodo https://doi.org/10.5281/zenodo.6516913 .
更多查看译文
关键词
unsupervised crack detection,complex stone masonry surfaces
AI 理解论文
溯源树
样例
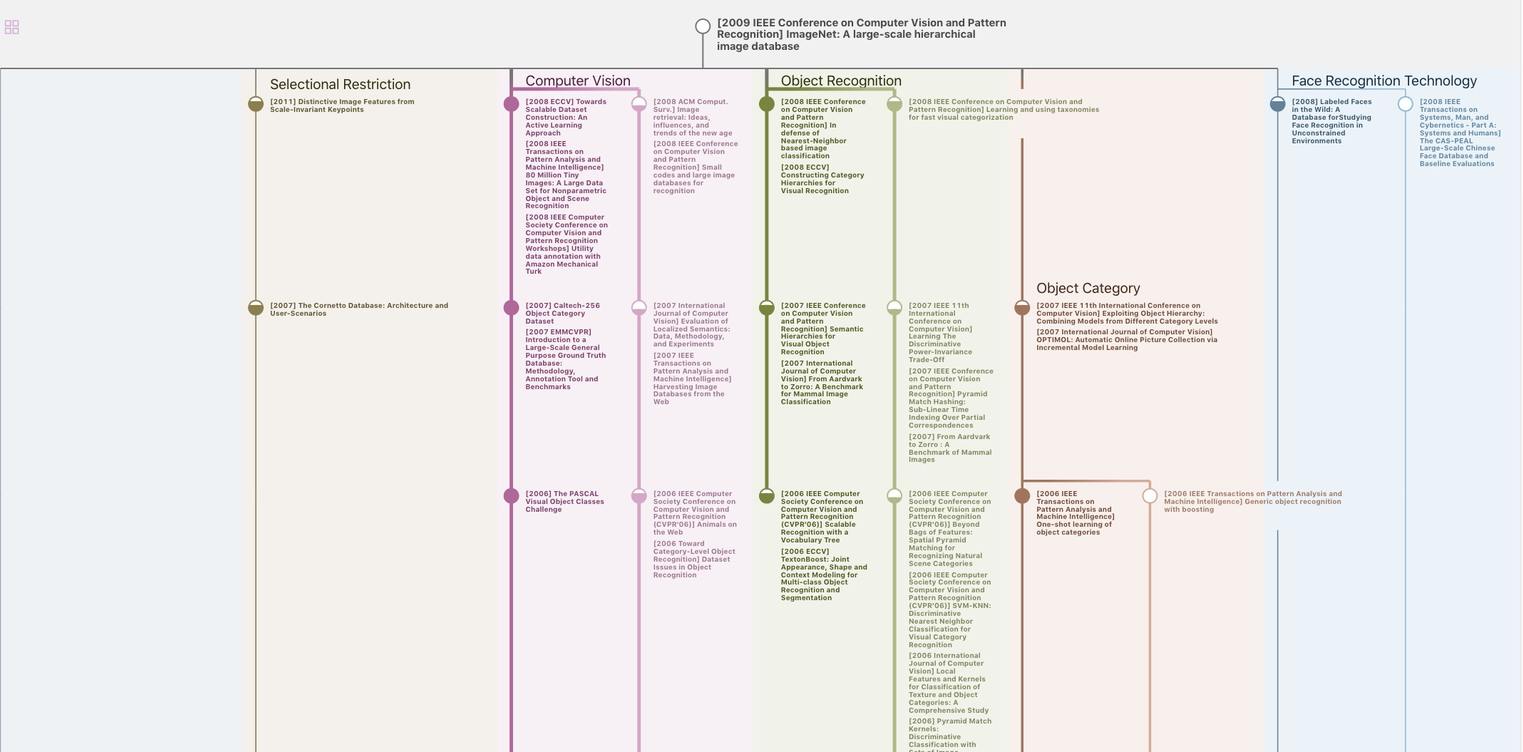
生成溯源树,研究论文发展脉络
Chat Paper
正在生成论文摘要