oBERTa: Improving Sparse Transfer Learning via improved initialization, distillation, and pruning regimes
CoRR(2023)
摘要
In this paper, we introduce the range of oBERTa language models, an easy-to-use set of language models, which allows Natural Language Processing (NLP) practitioners to obtain between 3.8 and 24.3 times faster models without expertise in model compression. Specifically, oBERTa extends existing work on pruning, knowledge distillation, and quantization and leverages frozen embeddings to improve knowledge distillation, and improved model initialization to deliver higher accuracy on a a broad range of transfer tasks. In generating oBERTa, we explore how the highly optimized RoBERTa differs from the BERT with respect to pruning during pre-training and fine-tuning and find it less amenable to compression during fine-tuning. We explore the use of oBERTa on a broad seven representative NLP tasks and find that the improved compression techniques allow a pruned oBERTa model to match the performance of BERTBASE and exceed the performance of Prune OFA Large on the SQUAD V1.1 Question Answering dataset, despite being 8x and 2x, respectively, faster in inference. We release our code, training regimes, and associated model for broad usage to encourage usage and experimentation.
更多查看译文
关键词
sparse transfer learning,distillation,improved initialization
AI 理解论文
溯源树
样例
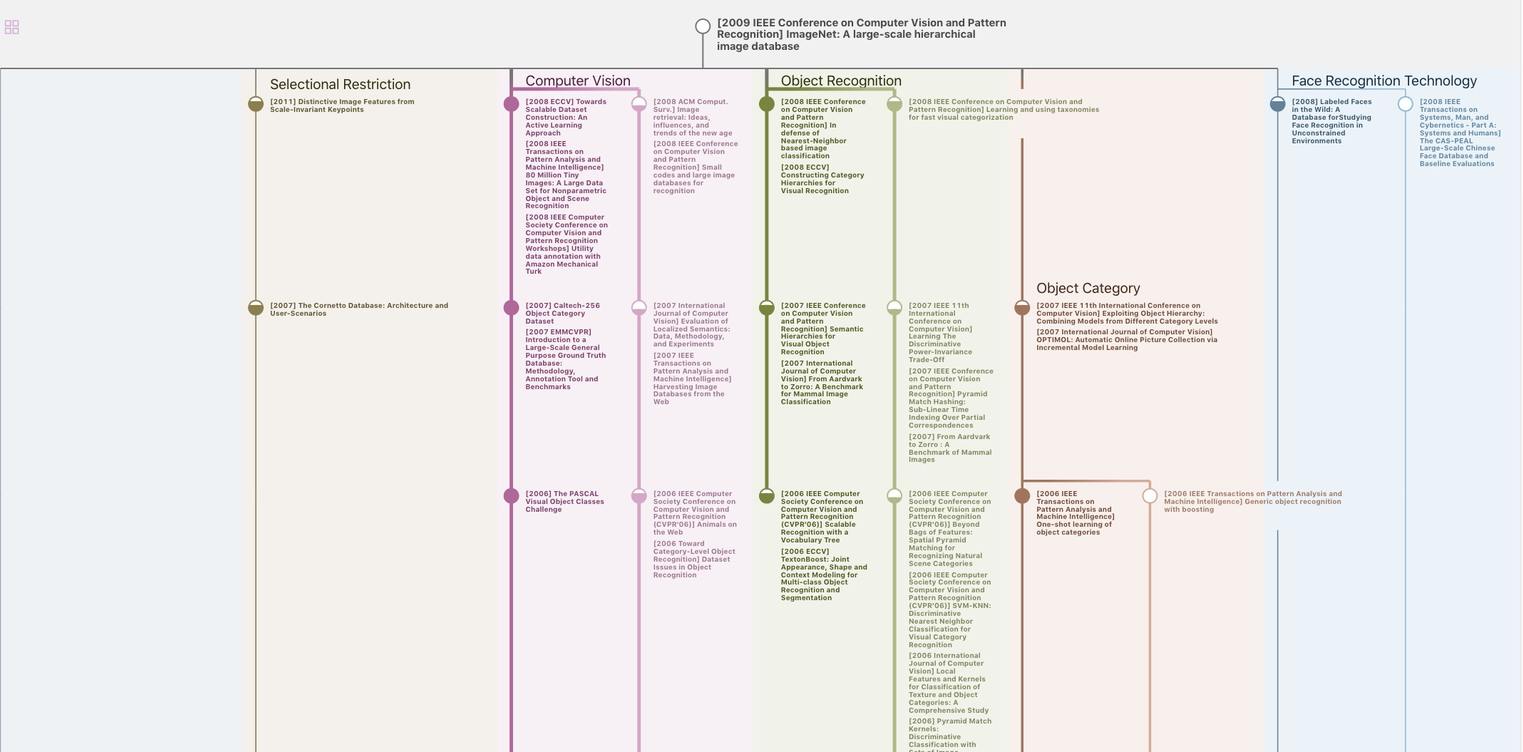
生成溯源树,研究论文发展脉络
Chat Paper
正在生成论文摘要