A Bayesian approach for two-stage multivariate Mendelian randomization with mixed outcomes.
Statistics in medicine(2023)
摘要
Many research studies have investigated the relationship between baseline factors or exposures, such as patient demographic and disease characteristics, and study outcomes such as toxicities or quality of life, but results from most of these studies may be problematic because of potential confounding effects (eg, the imbalance in baseline factors or exposures). It is important to study whether the baseline factors or exposures have causal effects on the clinical outcomes, so that clinicians can have better understanding of the diseases and develop personalized medicine. Mendelian randomization (MR) provides an efficient way to estimate the causal effects using genetic instrumental variables to handle confounders, but most of the existing studies focus on a single outcome at a time and ignores the correlation structure of multiple outcomes. Given that clinical outcomes like toxicities and quality of life are usually a mixture of different types of variables, and multiple datasets may be available for such outcomes, it may be much more beneficial to analyze them jointly instead of separately. Some well-established methods are available for building multivariate models on mixed outcomes, but they do not incorporate MR mechanism to deal with the confounders. To overcome these challenges, we propose a Bayesian-based two-stage multivariate MR method for mixed outcomes on multiple datasets, called BMRMO. Using simulation studies and clinical applications on the CO.17 and CO.20 studies, we demonstrate better performance of our approach compared to the commonly used univariate two-stage method.
更多查看译文
关键词
Bayesian analysis,Mendelian randomization,Metropolis-Hastings algorithm,genetic instrumental variable,mixed outcome,multivariate analysis
AI 理解论文
溯源树
样例
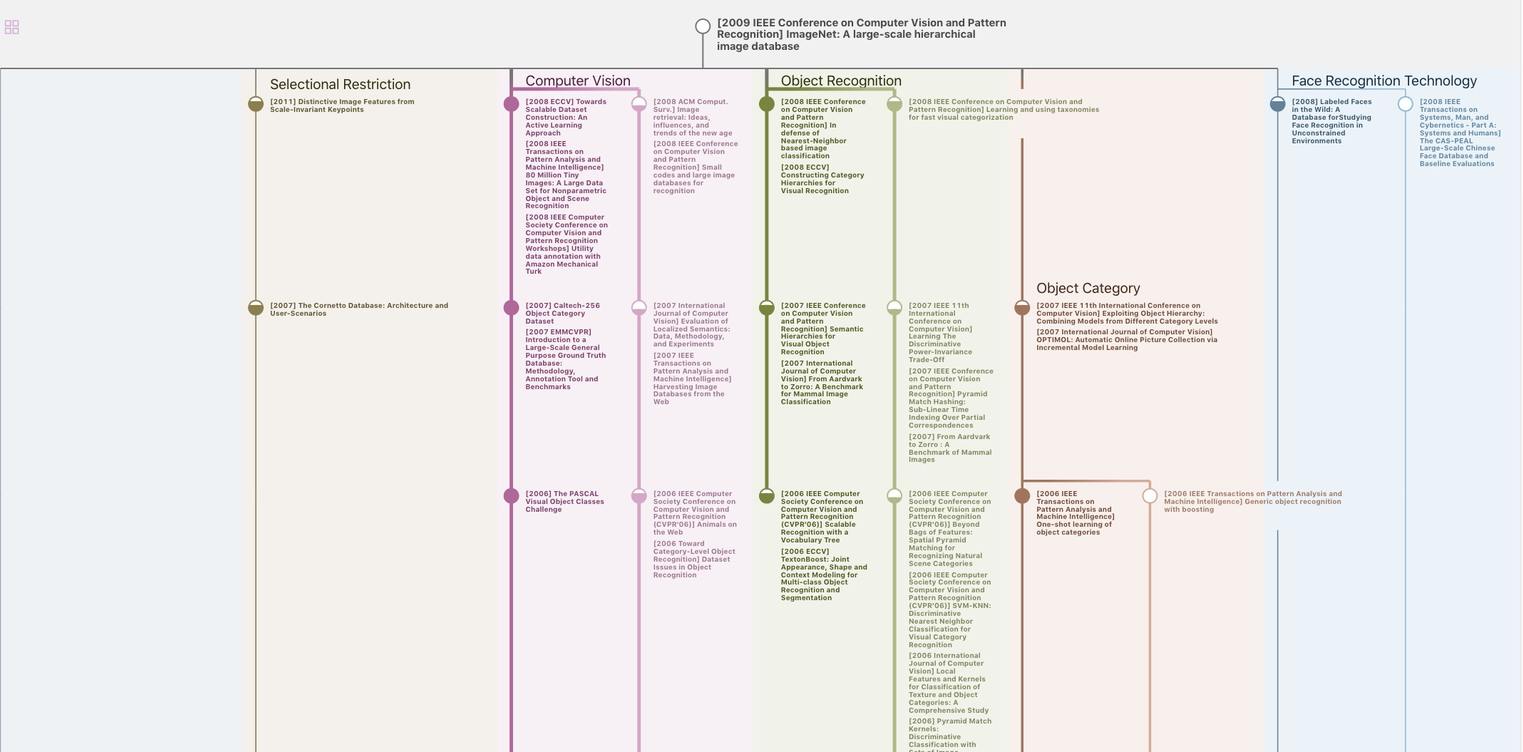
生成溯源树,研究论文发展脉络
Chat Paper
正在生成论文摘要