Fast estimation of multiple group generalized linear latent variable models for categorical observed variables.
Comput. Stat. Data Anal.(2023)
摘要
A computationally efficient method for marginal maximum likelihood estimation of multiple group generalized linear latent variable models for categorical data is introduced. The approach utilizes second-order Laplace approximations of the integrals in the likelihood function. It is demonstrated how second-order Laplace approximations can be utilized highly efficiently for generalized linear latent variable models by considering symmetries that exist for many types of model structures. In a simulation with binary observed variables and four correlated latent variables in four groups, the method has similar bias and mean squared error compared to adaptive Gauss-Hermite quadrature with five quadrature points while substantially improving computational efficiency. An empirical example from a large-scale educational assessment illustrates the accuracy and computational efficiency of the method when compared against adaptive Gauss-Hermite quadrature with three, five, and 13 quadrature points.
更多查看译文
关键词
Latent variable models,Item response theory,Integral approximation,Gauss-Hermite quadrature,Laplace approximation
AI 理解论文
溯源树
样例
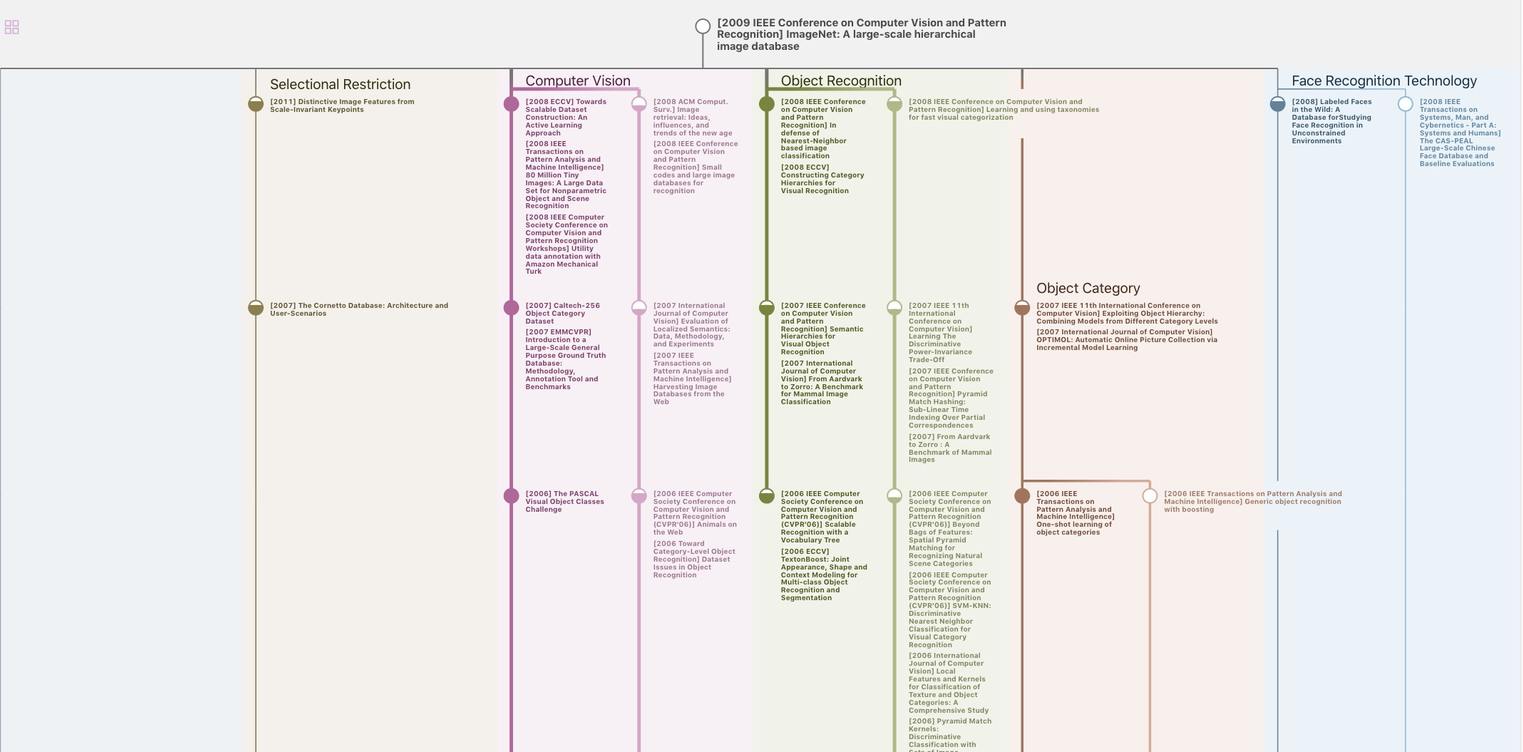
生成溯源树,研究论文发展脉络
Chat Paper
正在生成论文摘要