Data-driven surrogate modeling: Introducing spatial lag to consider spatial autocorrelation of flooding within urban drainage systems.
Environ. Model. Softw.(2023)
摘要
Data-driven surrogate modeling has been increasingly employed for flooding simulation of urban drainage systems (UDSs) due to its high computational efficiency and accuracy. However, spatial autocorrelation is prevalent in many typical scenarios, including the UDS. This omission of spatial information is very likely to cause the machine learning model to capture the wrong UDS overflow mechanism from the data. To capture the spatial autocorrelation, an artificial neural network (ANN)-based surrogate modeling method that introduces spatial lag to account for the spatial autocorrelation of flooding within the UDS is proposed and coupled with a genetic algorithm (GA) to reduce the uncertainty caused by random initialization of ANN. In this study, a sur -rogate modeling experiment was carried out for the Storm Water Management Model (SWMM). The experi-mental results show that the ANN can successfully capture the spatial autocorrelation induced by flooding within the UDS and accurately replicate the output simulated by SWMM.
更多查看译文
关键词
Machine learning-based surrogate modeling,(MLSM),Urban flooding simulation,Spatial autocorrelation,Spatial lag,Storm water management model(SWMM)
AI 理解论文
溯源树
样例
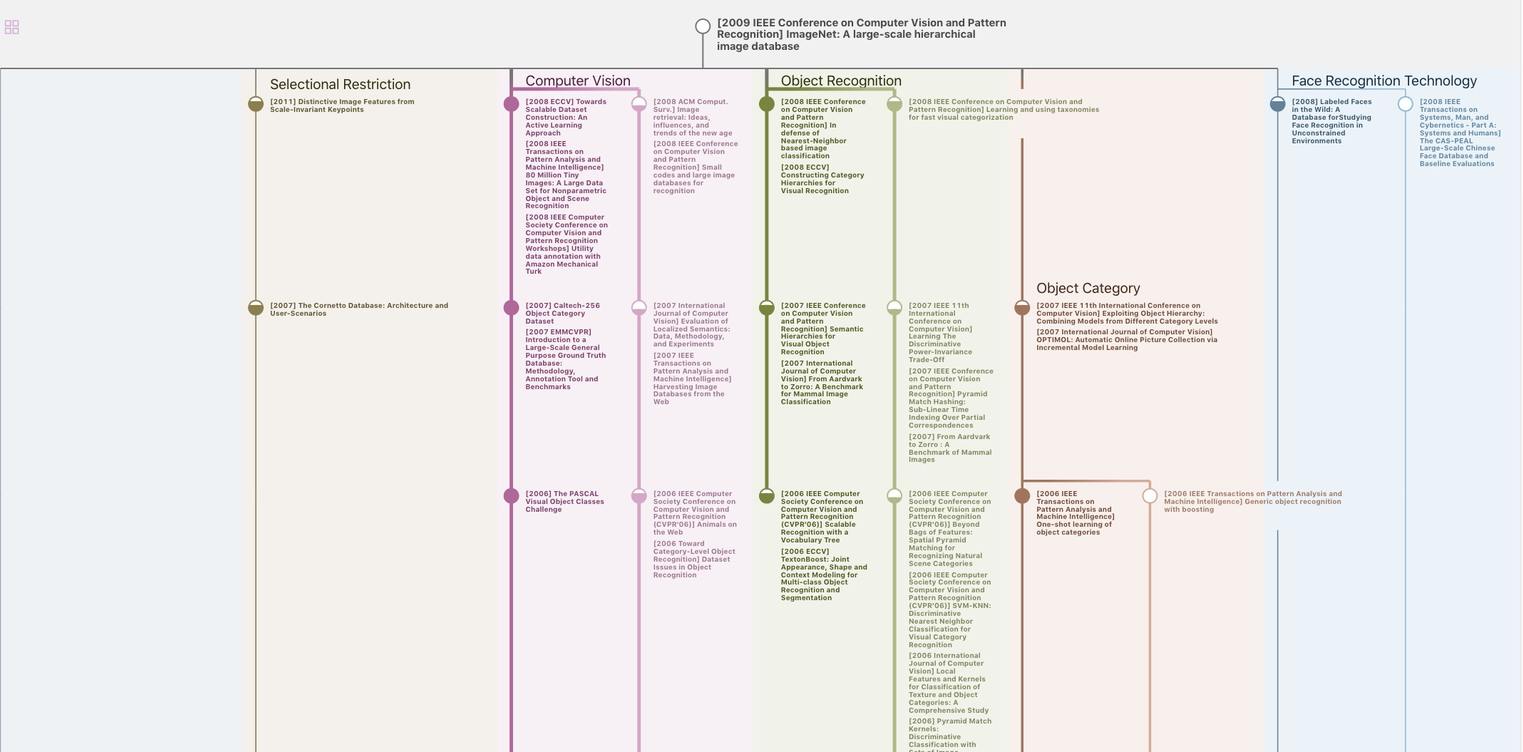
生成溯源树,研究论文发展脉络
Chat Paper
正在生成论文摘要