Deep Contrastive Anomaly Detection for Airline Ancillaries Prediction.
ICMLA(2022)
摘要
The increasing range of ancillary products offered by airlines is making existing, static frameworks obsolete. The changing expectations of customers have created a need for more dynamic and reactive offers. In order to tailor an offer to an individual journey, it is possible to leverage similar journeys and the observed outcomes using a semi-supervised approach. In this paper, a multi-stage deep learning framework, namely Deep Ancillaries Prediction (DAP), is developed to understand personalised demand for airline ancillaries and enhance pricing strategies. DAP solves the overlapping distribution problem and class imbalances observed in real-world airline datasets. The framework incorporates a contrastive learning module to learn richer feature embeddings and an autoencoder for semi-supervised learning into one framework, and outperforms current ancillary prediction systems. The modules can be trained separately and hence, are suitable for an online learning setting. This framework is designed to be transferable to different prediction tasks. Significant performance enhancements are attained compared to the current state-of-the-art algorithms.
更多查看译文
关键词
Airline ancillaries prediction, anomaly detection, contrastive learning, deep learning
AI 理解论文
溯源树
样例
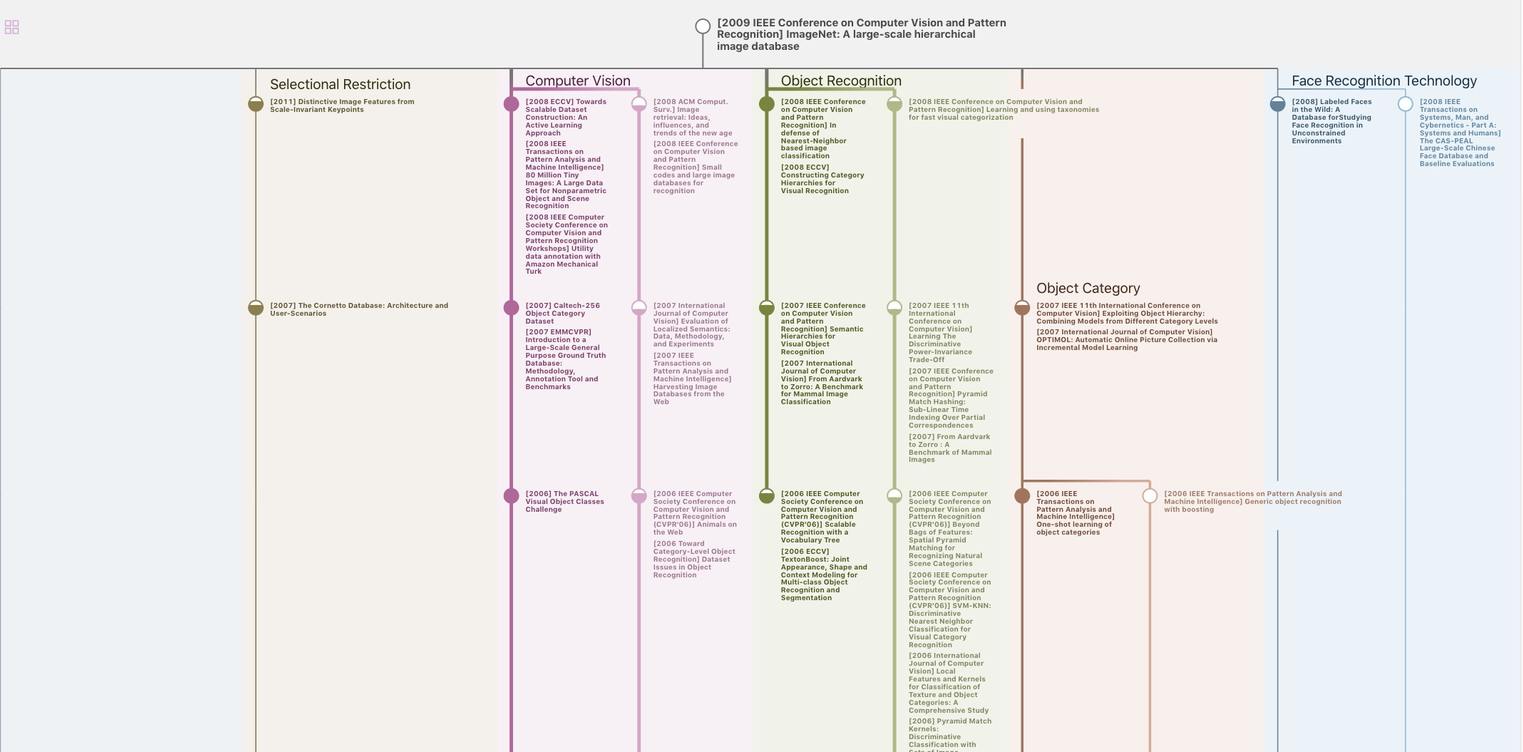
生成溯源树,研究论文发展脉络
Chat Paper
正在生成论文摘要