Impact of Labeling Noise on Machine Learning: A Cost-aware Empirical Study
ICMLA(2022)
摘要
Since the emergence of large datasets, machine learning models have demonstrated excellent performance in a wide range of applications. This accomplishment was made possible by the availability of large amounts of labeled datasets. Finding high-quality labeled datasets, on the other hand, is difficult to obtain. Acquiring high-quality datasets with limited class label noise becomes an important task since noisy datasets can affect the performance and structure of machine learning models. However, it is extremely difficult to reduce label noise significantly in real-world datasets unless using expensive expert annotators. This work studies the influence of varying degrees of label noise on the complexity and accuracy of machine learning models, based on considerable testing and research. It also explores how to reduce labeling costs while maintaining the desired accuracy.
更多查看译文
关键词
machine learning, deep learning, label noise, class label noise, Labeling Cost Optimization, mislabeled data
AI 理解论文
溯源树
样例
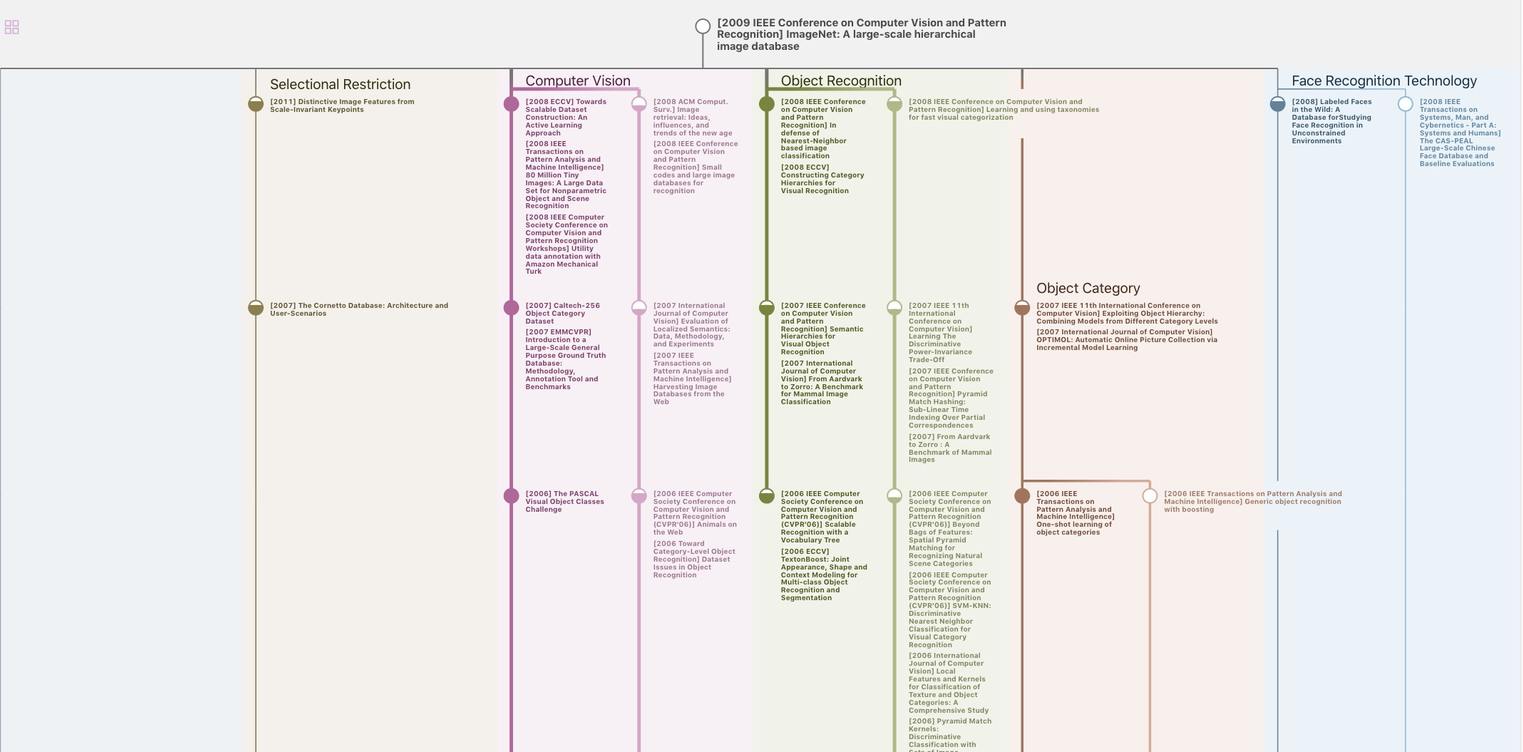
生成溯源树,研究论文发展脉络
Chat Paper
正在生成论文摘要