Knowledge Guided Two-player Reinforcement Learning for Cyber Attacks and Defenses.
ICMLA(2022)
摘要
Cyber defense exercises are an important avenue to understand the technical capacity of organizations when faced with cyber-threats. Information derived from these exercises often leads to finding unseen methods to exploit vulnerabilities in an organization. These often lead to better defense mechanisms that can counter previously unknown exploits. With recent developments in cyber battle simulation platforms, we can generate a defense exercise environment and train reinforcement learning (RL) based autonomous agents to attack the system described by the simulated environment. In this paper, we describe a two-player game-based RL environment that simultaneously improves the performance of both the attacker and defender agents. We further accelerate the convergence of the RL agents by guiding them with expert knowledge from Cybersecurity Knowledge Graphs on attack and mitigation steps. We have implemented and integrated our proposed approaches into the CyberBattleSim system.
更多查看译文
关键词
Reinforcement Learning,Knowledge Graphs,Cybersecurity
AI 理解论文
溯源树
样例
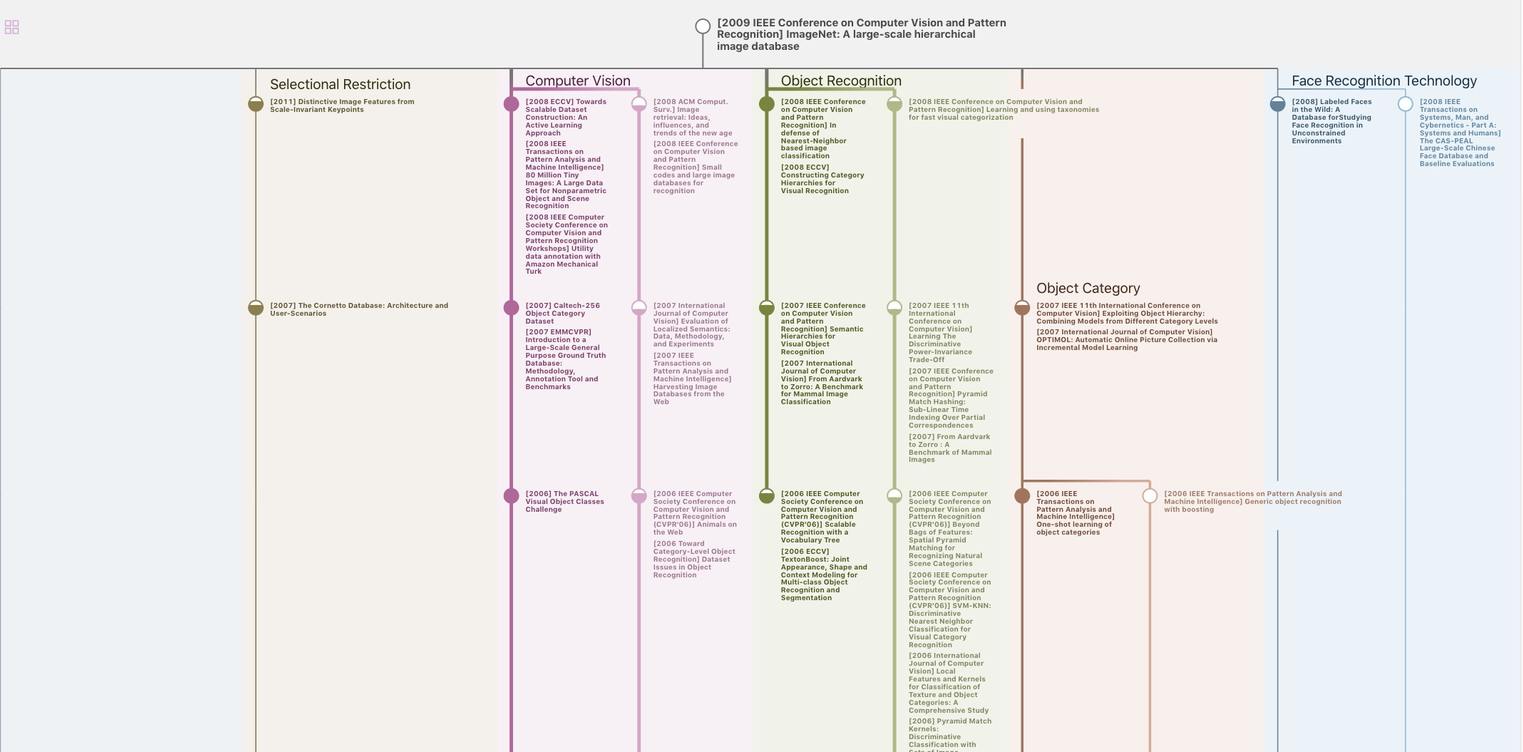
生成溯源树,研究论文发展脉络
Chat Paper
正在生成论文摘要