A Novel Approach for Synthetic Reduced Nearest-Neighbor Leveraging Neural Networks.
ICMLA(2022)
摘要
Synthetic Reduced Nearest Neighbor is a nearest neighbor model that is constrained on synthetic samples (i.e., prototypes). The body of work on such models includes proposals for improving the interpretability and optimization of SRNN models using expectation maximization. Motivated by the promise of this paradigm, we propose a novel Expectation Maximization approach for Synthetic Reduced Nearest Neighbors leveraging neural networks. Furthermore, we compare the performance of our proposed technique to classical state-of-the-art machine learning methods such as random forest and ensemble models. The empirical results demonstrate the advantages of using neural networks in lieu of an expectation maximization algorithm.
更多查看译文
关键词
Synthetic Reduced Nearest Neighbor,Machine learning,Regression,Expectation-Maximization Algorithm
AI 理解论文
溯源树
样例
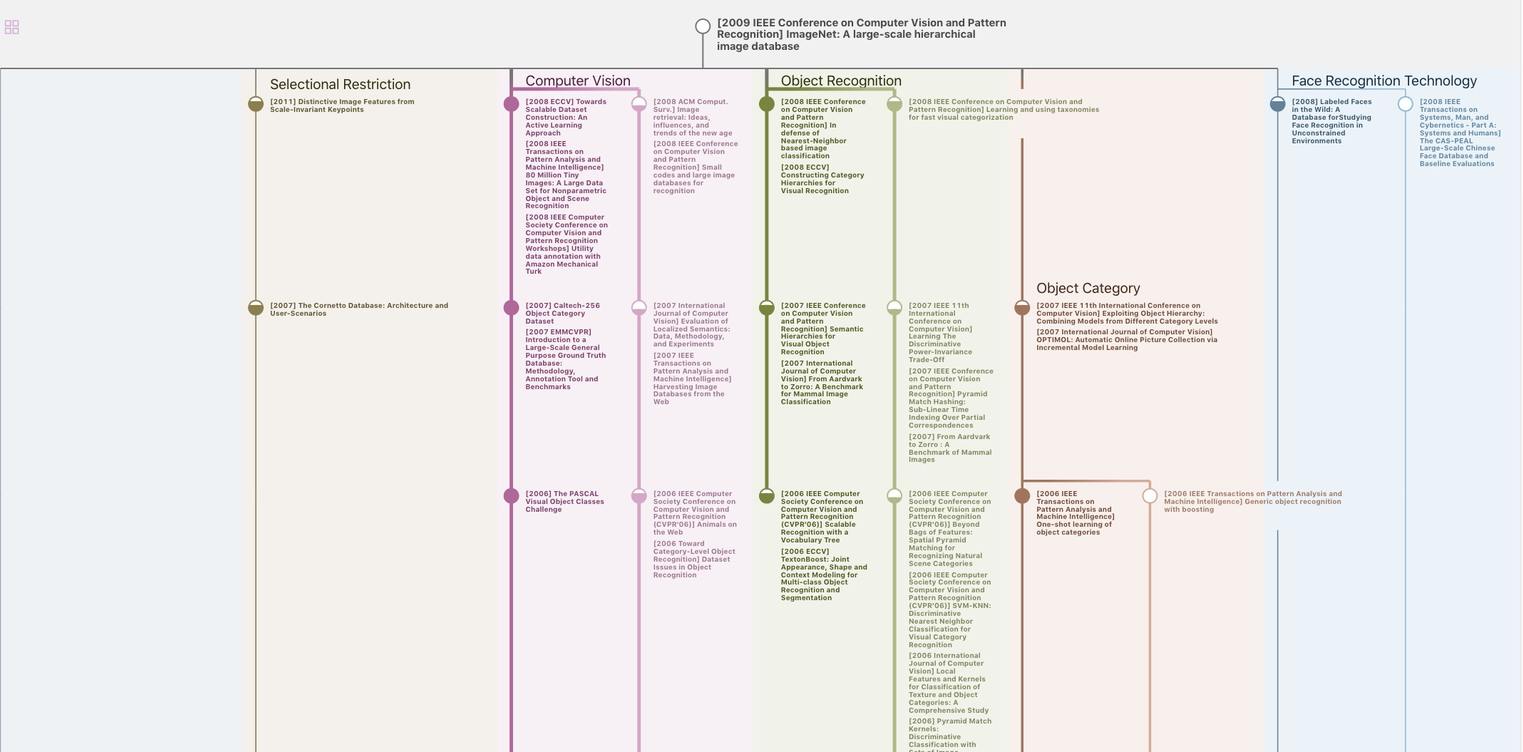
生成溯源树,研究论文发展脉络
Chat Paper
正在生成论文摘要