Connecting the Semantic Dots: Zero-shot Learning with Self-Aligning Autoencoders and a New Contrastive-Loss for Negative Sampling.
ICMLA(2022)
摘要
We introduce a novel zero-shot learning (ZSL) method, known as `self-alignment training', and use it to train a vanilla autoencoder which is then evaluated on four prominent ZSL Tasks CUB, SUN, AWA1&2. Despite being a far simpler model than the competition, our method achieved results on par with SOTA. In addition, we also present a novel `contrastive-loss' objective to allow autoencoders to learn from negative samples. In particular, we achieve new SOTA of 64.5 on AWA2 for Generalised ZSL and a new SOTA for standard ZSL of 47.7 on SUN. The code is publicly accessible on https://github.com/ Wluper/satae.
更多查看译文
关键词
Zero Shot Learning,Autoencoders,Open Source
AI 理解论文
溯源树
样例
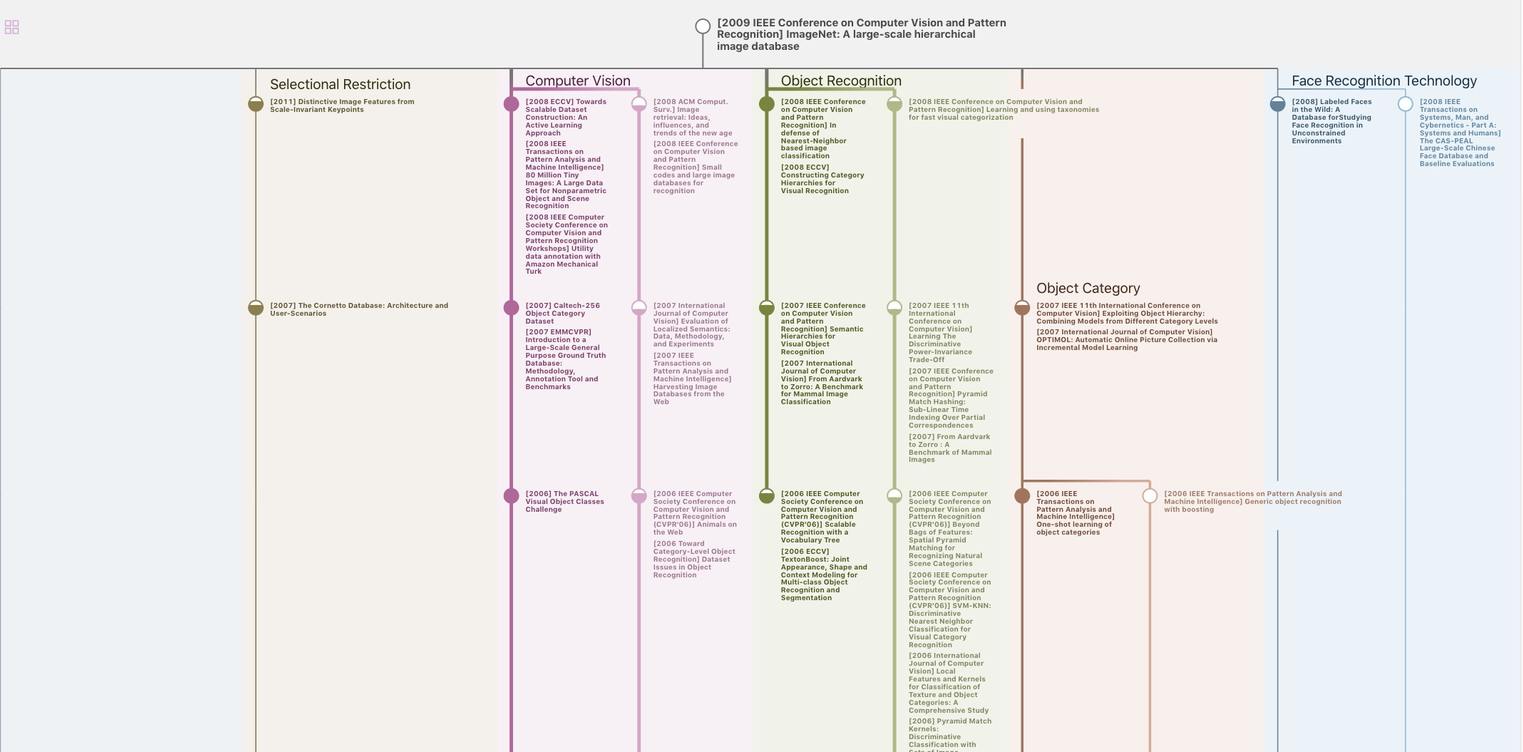
生成溯源树,研究论文发展脉络
Chat Paper
正在生成论文摘要