Assessing Inter-Annotator Agreement for Medical Image Segmentation.
IEEE Access(2023)
摘要
Artificial Intelligence (AI)-based medical computer vision algorithm training and evaluations depend on annotations and labeling. However, variability between expert annotators introduces noise in training data that can adversely impact the performance of AI algorithms. This study aims to assess, illustrate and interpret the inter-annotator agreement among multiple expert annotators when segmenting the same lesion(s)/abnormalities on medical images. We propose the use of three metrics for the qualitative and quantitative assessment of inter-annotator agreement: 1) use of a common agreement heatmap and a ranking agreement heatmap; 2) use of the extended Cohen's kappa and Fleiss' kappa coefficients for a quantitative evaluation and interpretation of inter-annotator reliability; and 3) use of the Simultaneous Truth and Performance Level Estimation (STAPLE) algorithm, as a parallel step, to generate ground truth for training AI models and compute Intersection over Union (IoU), sensitivity, and specificity to assess the inter-annotator reliability and variability. Experiments are performed on two datasets, namely cervical colposcopy images from 30 patients and chest X-ray images from 336 tuberculosis (TB) patients, to demonstrate the consistency of inter-annotator reliability assessment and the importance of combining different metrics to avoid bias assessment.
更多查看译文
关键词
Heating systems,Image segmentation,Annotations,Reliability,Lesions,Biomedical imaging,Artificial intelligence,agreement,inter-annotator,heatmap,STAPLE,Cohen's kappa,Fleiss' kappa
AI 理解论文
溯源树
样例
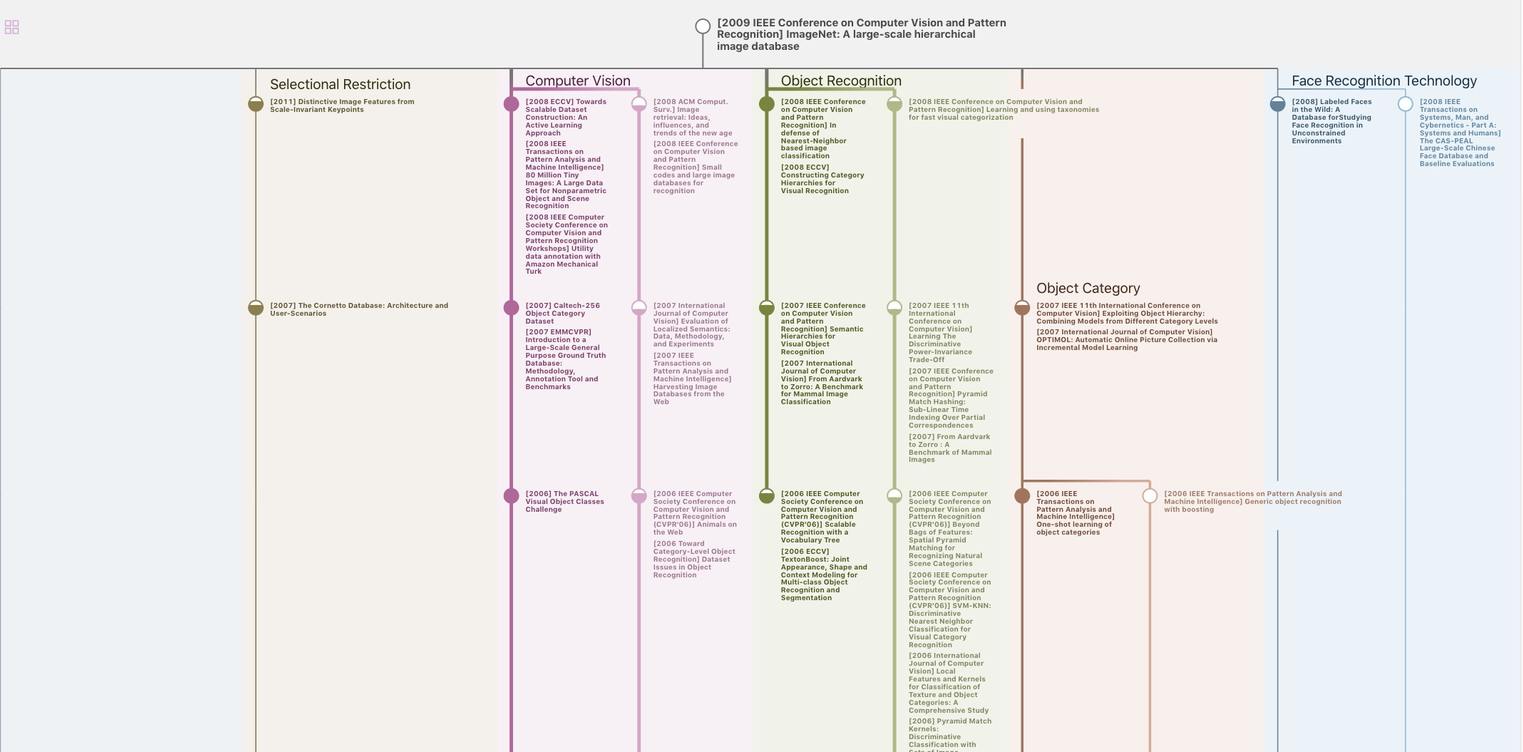
生成溯源树,研究论文发展脉络
Chat Paper
正在生成论文摘要