CAT: Using Cross Attention Transformation to Build Correlation of RGB-D for Road Detection.
BigCom(2022)
摘要
Road detection plays a vital role in advanced driver assistance systems (ADAS). In recent years, the mainstream methods use the data fusion to combine the advantages of RGB images and depth images. However, the fusion operation utilizes a simple pixel-wise summation and concatenation strategy, leading to lose the correlation between the two types of data. To this end, inspired by the core idea of Transformer, we propose a cross attention transformation module, which effectively fuses the RGB features and depth features using the attention mechanism. Specifically, the correlation attention features of RGB-D are generated by the cross-attention mechanism. Then the attention features are fused to enhance the attention response and capture global context information. Experiments are conducted in the two popular datasets including the KITTI road dataset and Cityscapes dataset. Empirical results show that the accuracy of road detection is improved by our fusion method. In the KITTI road Benchmark, Our method gets the better MaxF in UM_Road(95.02 %) and UMM_Road(97.03%).
更多查看译文
关键词
road detection,data fusion,attention mecha-nism,Transformer
AI 理解论文
溯源树
样例
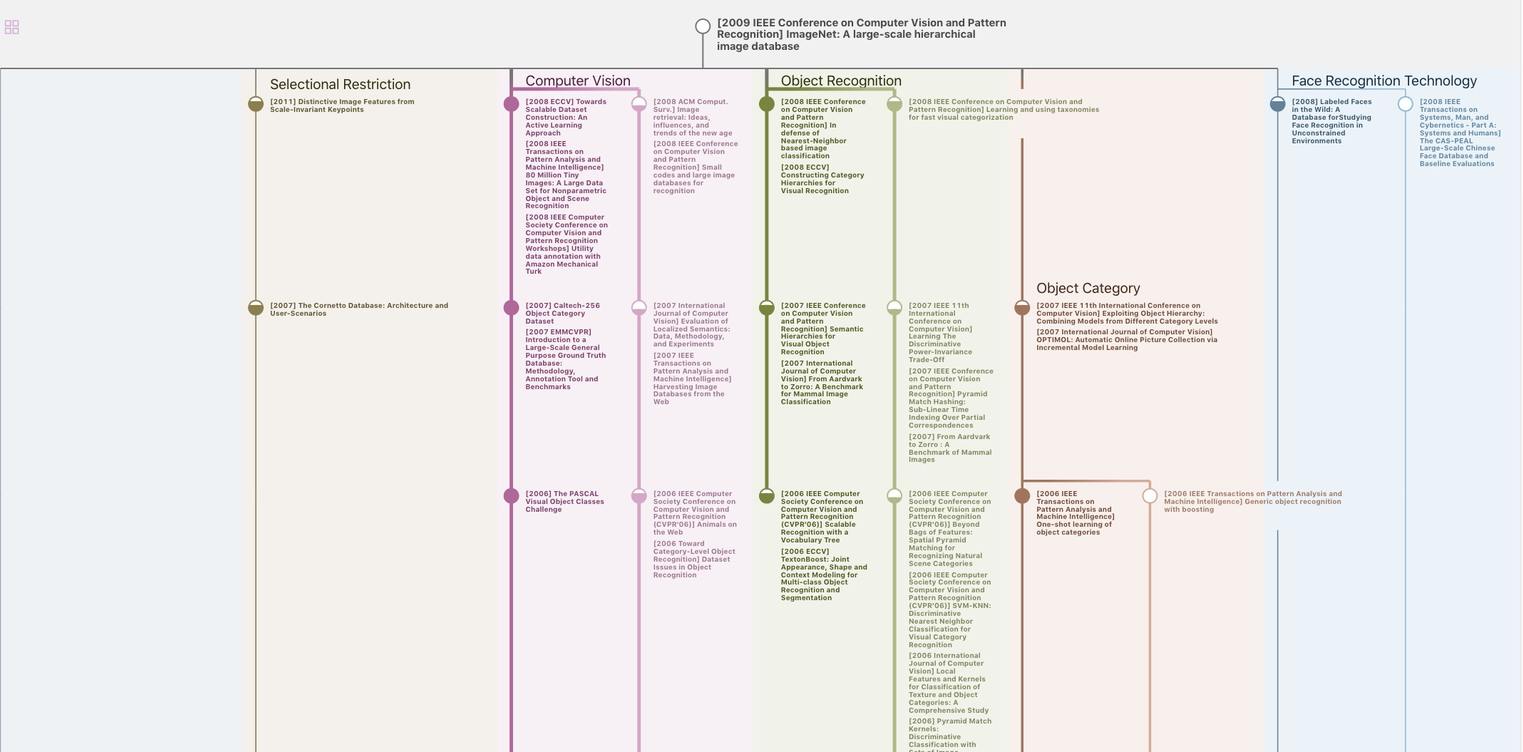
生成溯源树,研究论文发展脉络
Chat Paper
正在生成论文摘要