An efficient unfolding network with disentangled spatial-spectral representation for hyperspectral image super-resolution
Inf. Fusion(2023)
摘要
Hyperspectral image super-resolution (HSI SR) is dramatically impacted by high spectral dimensionality, insufficient spatial resolution, and limited availability of training samples. Current approaches mainly rely on complex data-driven models to address some of these challenges, and the characteristics of HSI are not fully considered in the model design. In this paper, we propose an efficient unfolding network with disentangled spatial-spectral representation (EUNet) for HSI SR by combining domain knowledge (i.e., spectral correlation, degradation model, and structure prior) with deep learning. Specifically, the optimization process of the superresolution prior-driven Maximum A Posterior (MAP) framework is unfolded into an interpretable multi-stage network, which inherits the advantages of deep learning-based image super-resolution (e.g., feature extraction in low-resolution space) and explicitly imposes the degradation model constraint. To well incorporate the structure prior of HSI, spatial and spectral feature extraction is disentangled by a variant of depthwise separable convolution, and spectral correlation is embedded by a lightweight spectral attention mechanism, so that the difficulty and computational complexity of feature learning are greatly reduced. Experiments on benchmark datasets with different degradation models demonstrate the feasibility and superiority of the proposed EUNet over other state-of-the-art methods in terms of evaluation metrics and computational complexity. The source code is available at https://github.com/denghong-liu/EUNet.
更多查看译文
关键词
Hyperspectral image,Super -resolution,Unfolding network,Disentangled spatial -spectral representation
AI 理解论文
溯源树
样例
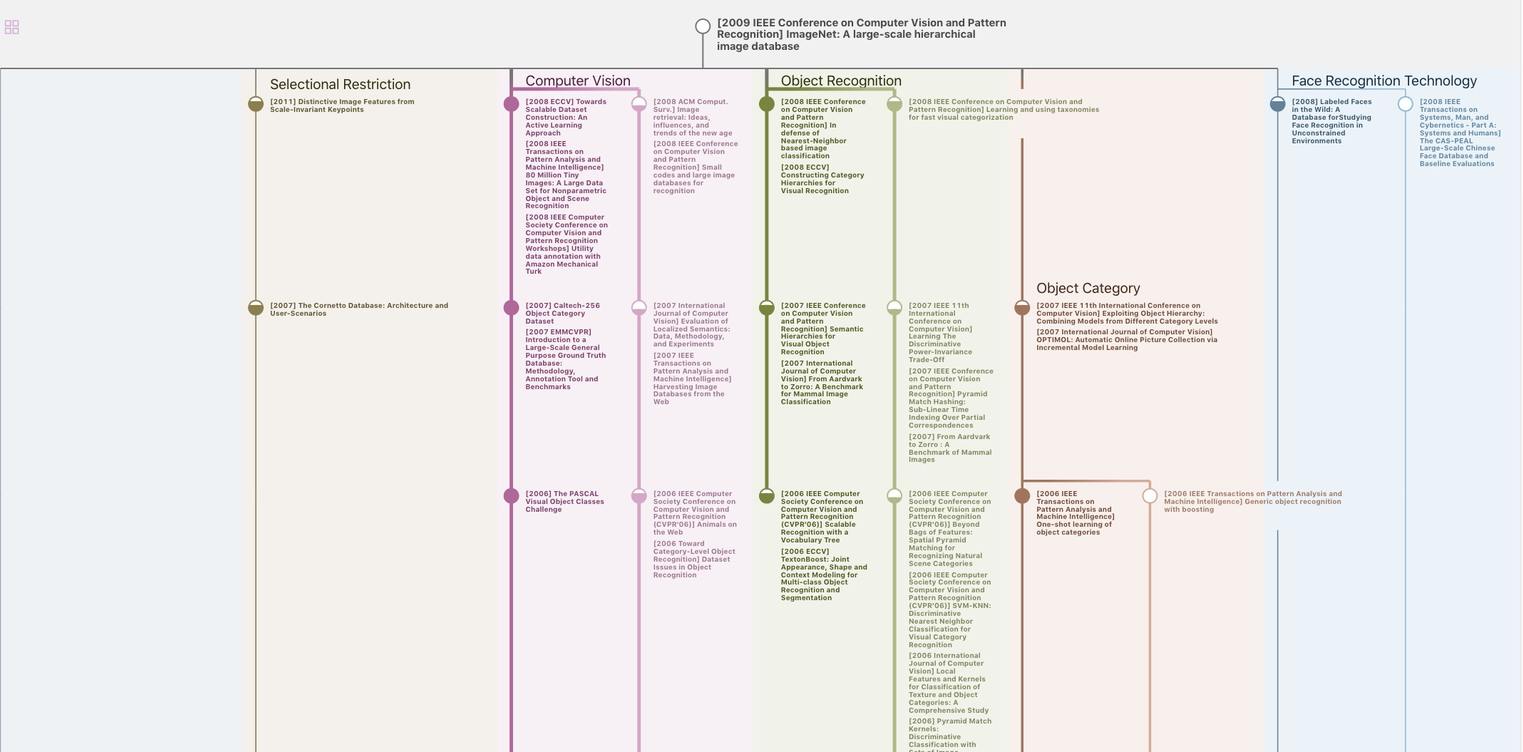
生成溯源树,研究论文发展脉络
Chat Paper
正在生成论文摘要