U-Shaped Low-Complexity Type-2 Fuzzy LSTM Neural Network for Speech Enhancement.
IEEE Access(2023)
摘要
Speech enhancement (SE) aims to improve the intelligibility and perceptual quality of speech contaminated by noise signals through spectral or temporal changes. Deep learning models achieve speech enhancement and estimate the magnitude spectrum. This paper proposes a novel and computationally efficient deep learning model to enhance noisy speech. The model pre-processes the noisy speech magnitude by redistributing energy from high-energy voiced segments to low-energy unvoiced segments using an adaptive power law transformation while maintaining the total energy of the speech signals constant. A U-shaped fuzzy long short-term memory (UFLSTM) estimates the magnitude of a time-frequency (T-F) mask by using the pre-processed data. Residual connections to the similar-shaped layers are added to avoid gradient decay. Attention process is adopted by modifying the forget gate of UFLSTM. To make a causal speech enhancement system, the processing does not include any future audio frames. We compare the proposed speech enhancement to other deep learning models in different noisy environments with signal-to-noise ratios of 0 dB, 5 dB, and 10 dB. The experiments show that the proposed SE system outscores the competing deep learning models and considerably improves speech intelligibility and quality. In terms of STOI and PESQ, the LibriSpeech database improves results by (0.211) 21.1% and (0.95) 36.39%, respectively, over noisy speech in seen noisy conditions, and by (0.199) 19.9% and (0.94) 35.69% over noisy speech in unseen noisy conditions. Further, the cross-corpus analysis shows that proposed SE system performs better when trained with the DNS dataset as compared to the LibriSpeech, VoiceBank, and TIMIT datasets.
更多查看译文
关键词
Speech enhancement,Noise measurement,Logic gates,Deep learning,Computer architecture,Computational modeling,Microprocessors,Energy consumption,Energy redistribution,LSTM,residual connections,speech enhancement,and time-frequency masking
AI 理解论文
溯源树
样例
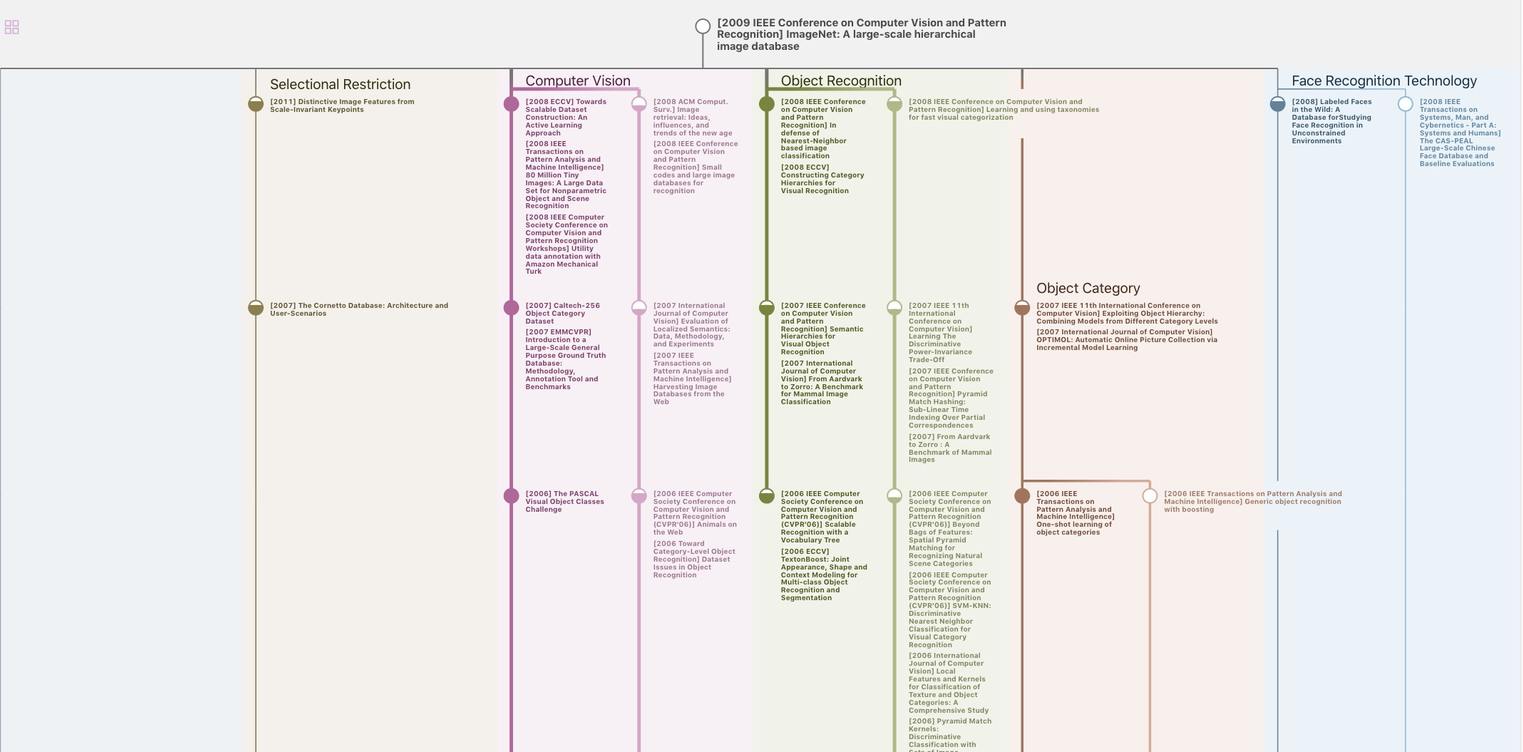
生成溯源树,研究论文发展脉络
Chat Paper
正在生成论文摘要