RtSNN: Building a Real-time Siamese Neural Networks Model for Few-shot Action Recognition.
BigCom(2022)
摘要
The technology of real-time action recognition based on wearable sensors has been widely used by organizations and individuals to provide high-quality healthcare services. For instance, real-time action recognition using sports sensors can be used to predict irregular actions of athletes to avoid serious sports injuries. However, the collection and annotation of action data are always expensive, and only few high-quality labeled data are available. Moreover, existing studies on few-shot action recognition mainly focus on videos analysis, rather than targeting real-time action recognition. Therefore, how to enable real-time action recognition with few-shot data is still challenging and remains to be fully explored. In this paper, we propose the first siamese neural networks for real-time few-shot action recognition. Our main idea is to use augmented data from the original minority labeled action data to recognize real-time actions. To address the challenge of overfitting in few-shot learning tasks, we devise a scheme to effectively augment the original data. The proposed model, named Real-time Siamese Neural Networks (RtSNN), can learn a feature space in which all real-time action data are transformed into feature vectors. By leveraging the design philosophy of metric learning, RtSNN can classify different actions by the distance learned in the feature space. We perform extensive experiments on real-world action datasets to evaluate the RtSNN model. The evaluation results confirm that RtSNN can effectively recognize real-time actions. It can maintain the highest classification accuracy compared with baseline models.
更多查看译文
关键词
real-time action recognition,siamese neural networks,few-shot learning
AI 理解论文
溯源树
样例
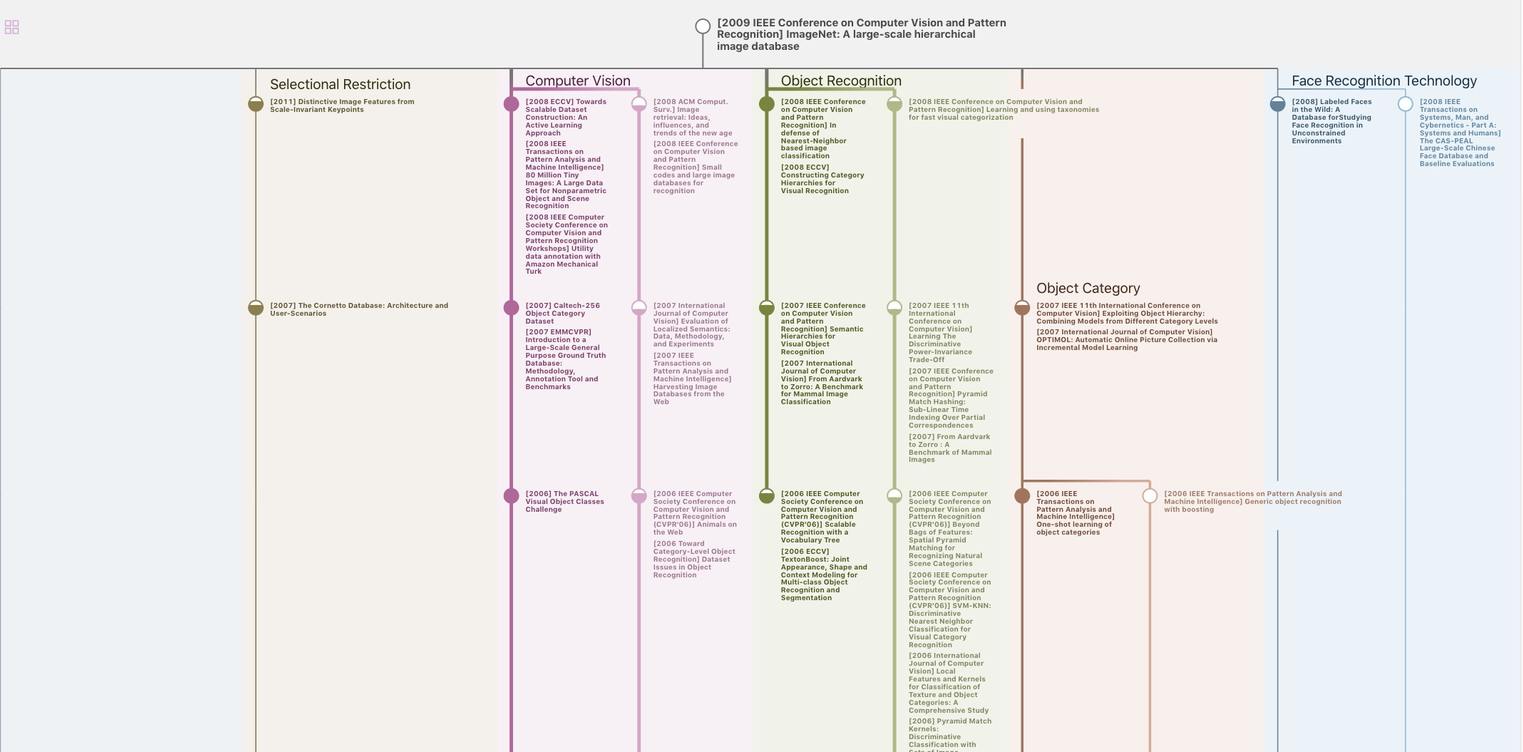
生成溯源树,研究论文发展脉络
Chat Paper
正在生成论文摘要