Multi-view Feature-based SSD Failure Prediction: What, When, and Why.
FAST(2023)
摘要
Solid state drives (SSDs) play an important role in large-scale data centers. SSD failures affect the stability of storage systems and cause additional maintenance overhead. To predict and handle SSD failures in advance, this paper proposes a multi-view and multi-task random forest (MVTRF) scheme. MVTRF predicts SSD failures based on multi-view features extracted from both long-term and short-term monitoring data of SSDs. Particularly, multi-task learning is adopted to simultaneously predict what type of failure it is and when it will occur through the same model. We also extract the key decisions of MVTRF to analyze why the failure will occur. These details of failure would be useful for verifying and handling SSD failures. The proposed MVTRF is evaluated on the large-scale real data from data centers. The experimental results show that MVTRF has higher failure prediction accuracy and improves precision by 46.1% and recall by 57.4% on average compared with the existing schemes. The results also demonstrate the effectiveness of MVTRF on failure type and time prediction and failure cause identification, which helps to improve the efficiency of failure handling.
更多查看译文
AI 理解论文
溯源树
样例
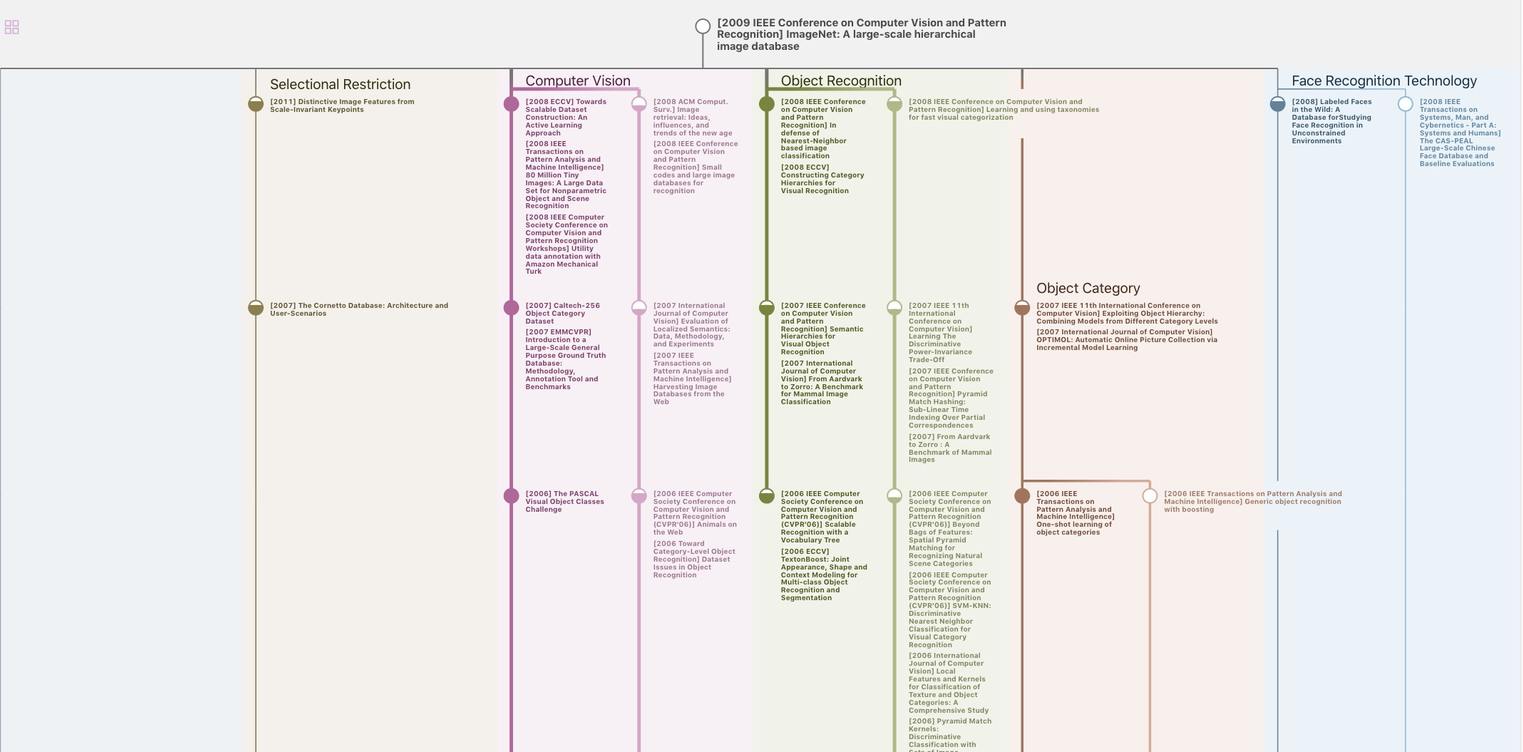
生成溯源树,研究论文发展脉络
Chat Paper
正在生成论文摘要