A 28nm Horizontal-Weight-Shift and Vertical-feature-Shift-Based Separate-WL 6T-SRAM Computation-in-Memory Unit-Macro for Edge Depthwise Neural-Networks.
ISSCC(2023)
摘要
SRAM-based computation-in-memory (CIM) has shown great potential in improving the energy efficiency of edge-AI devices. Most CIM work [3–4] is targeted at MAC operations with a higher input (IN), weight (W) and output (OUT) precision, which is suitable for standard-convolution layers and fully-connected layers. Edge-AI neural networks tradeoff inference accuracy for network parameters. Depthwise (DW) convolution support is essential for many light-CNN models, such as MobileNet-V2. However, when applying depthwise convolution, recent SRAM CIMs that only support keeping weights inside macro (weight-stationary) face three challenges: (1) decreased energy efficiency due to the short accumulation length (
$3\times 3$
kernel size) and large DW channel numbers [2-5]; (2) poor array utilization and large buffer-to-macro (B2M) power dissipation due to redundant data transmission, and; (3) the computation of a sparse, light network needs a high precision with a shorter access time and a smaller area for the readout circuit, as shown in Figure 7.5.1.
更多查看译文
AI 理解论文
溯源树
样例
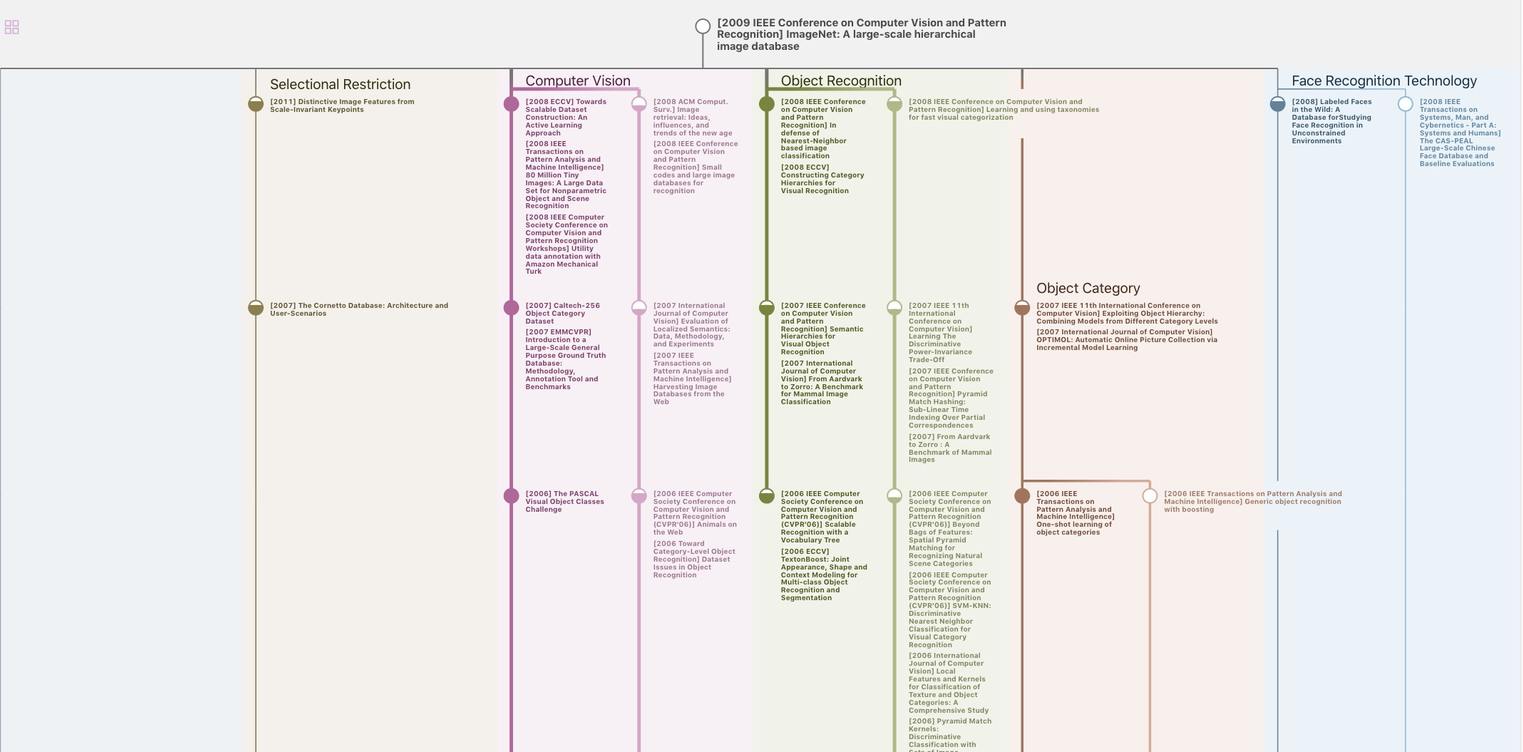
生成溯源树,研究论文发展脉络
Chat Paper
正在生成论文摘要