Cost-sensitive learning with modified Stein loss function.
Neurocomputing(2023)
摘要
Cost-sensitive learning (CSL), which has gained widespread attention in class imbalance learning (CIL), can be implemented either by tuning penalty parameters or by designing new loss functions. In this paper, we propose a cost-sensitive learning method with a modified Stein loss function (CSMS) and a robust CSMS (RCSMS). Specifically, CSMS is flexible, as it realizes CSL from above two aspects simultane-ously. In contrast, RCSMS merely achieves CSL by tuning penalty parameters, but the adopted loss func-tion makes it insensitive to noise. To our best knowledge, it is the first time for Stein loss function derived from statistics to be applied in machine learning, which not only offers two alternative class imbalance solutions but also provides a novel idea for the design of loss functions in CIL. The mini-batch stochastic sub-gradient descent (MBGD) approach is employed to optimize CSMS and RCSMS. Meanwhile, the Rademacher complexity is used to analyze their generalization error bounds. Extensive experiments pro-foundly confirm the superiority of both models over benchmarks.(c) 2023 Elsevier B.V. All rights reserved.
更多查看译文
关键词
Class imbalance learning,Cost-sensitive learning,Stein loss function,Penalty parameter,Support vector machine
AI 理解论文
溯源树
样例
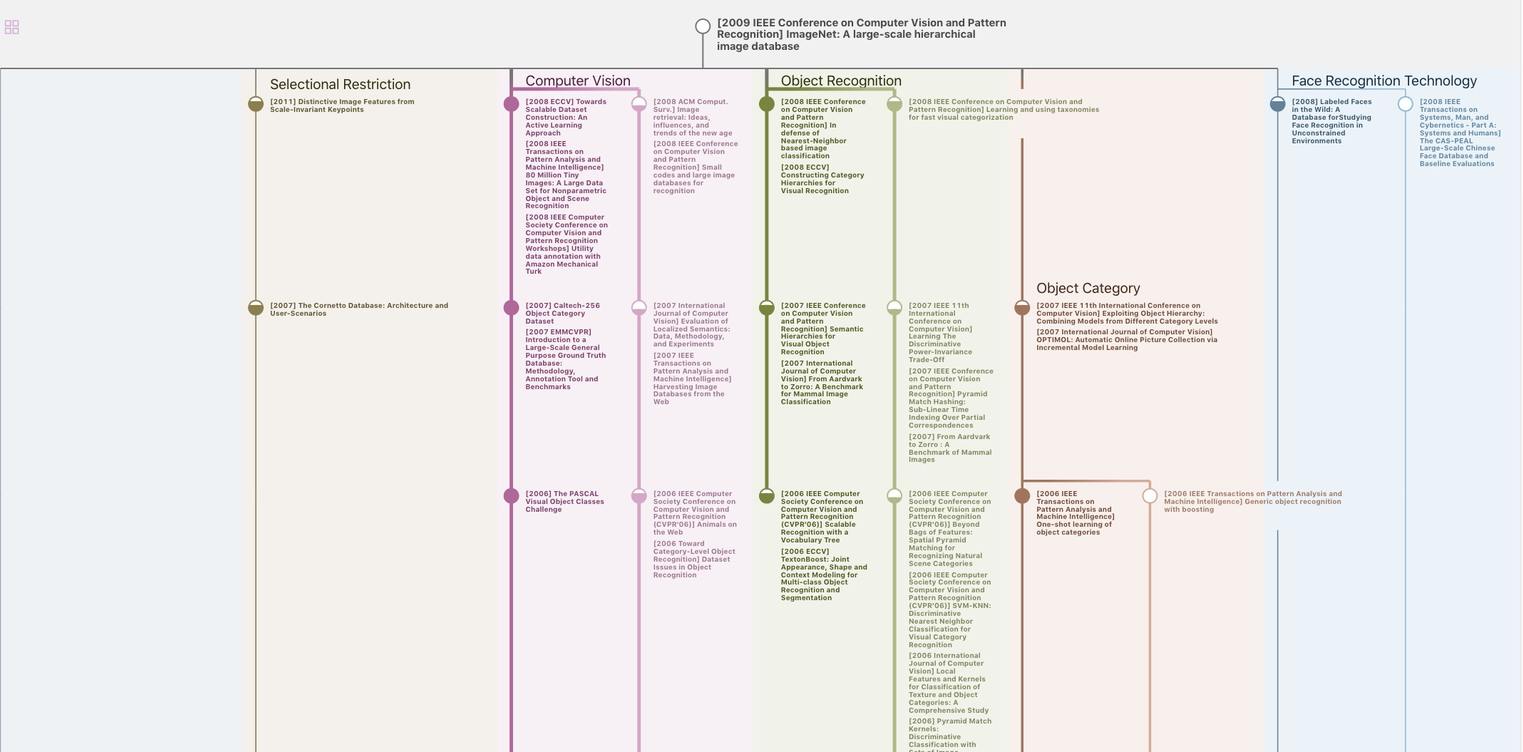
生成溯源树,研究论文发展脉络
Chat Paper
正在生成论文摘要