Multi-User mmWave Massive-MIMO Hybrid Beamforming: A Quantize Deep Learning Approach.
NCC(2023)
摘要
Hybrid precoding is an integral function in multi-user millimeter wave (mmWave) massive multiple-input multiple-output (MIMO) systems to reduce complexity and cost while obtaining an acceptable sum rate. Recent research on hybrid beamforming is frequently driven by convolution neural network (CNN) based deep learning (DL) techniques. These DL-based techniques use huge CNN sizes and floating point weights and activations that make the hardware implementation of such systems very complex on resource-constrained FPGA devices. In this work, we provide a quantized deep learning framework to boost performance while requiring less computation complexity than traditional DL-based beamforming methods. In actuality, we create a CNN-based DL model for mmWave massive MIMO hybrid beamforming design (BFNet) that accepts the noisy channel state information as input and outputs the precoders and combiners. To lower the complexity of the proposed scheme, quantized CNN with low precision weights and activations has been used. There are two main steps to the process. Initially, we use an exhaustive search algorithm to select the beamformers from a feasible set while maximizing the system sum capacity. The chosen beamformers are then employed as target labels for quantization-aware training (QAT) of the CNN. Through multiple, in-depth simulations, we assess the effectiveness of the proposed approach and demonstrate that the proposed BFNet performs better than traditional DL-based beamforming methods and also offers low complexity. Additionally, the proposed approach shows robustness to the increasing number of users.
更多查看译文
关键词
Quantized Deep Learning,mmWave-MIMO,hybrid beamforming,multi user MIMO,spectral efficiency
AI 理解论文
溯源树
样例
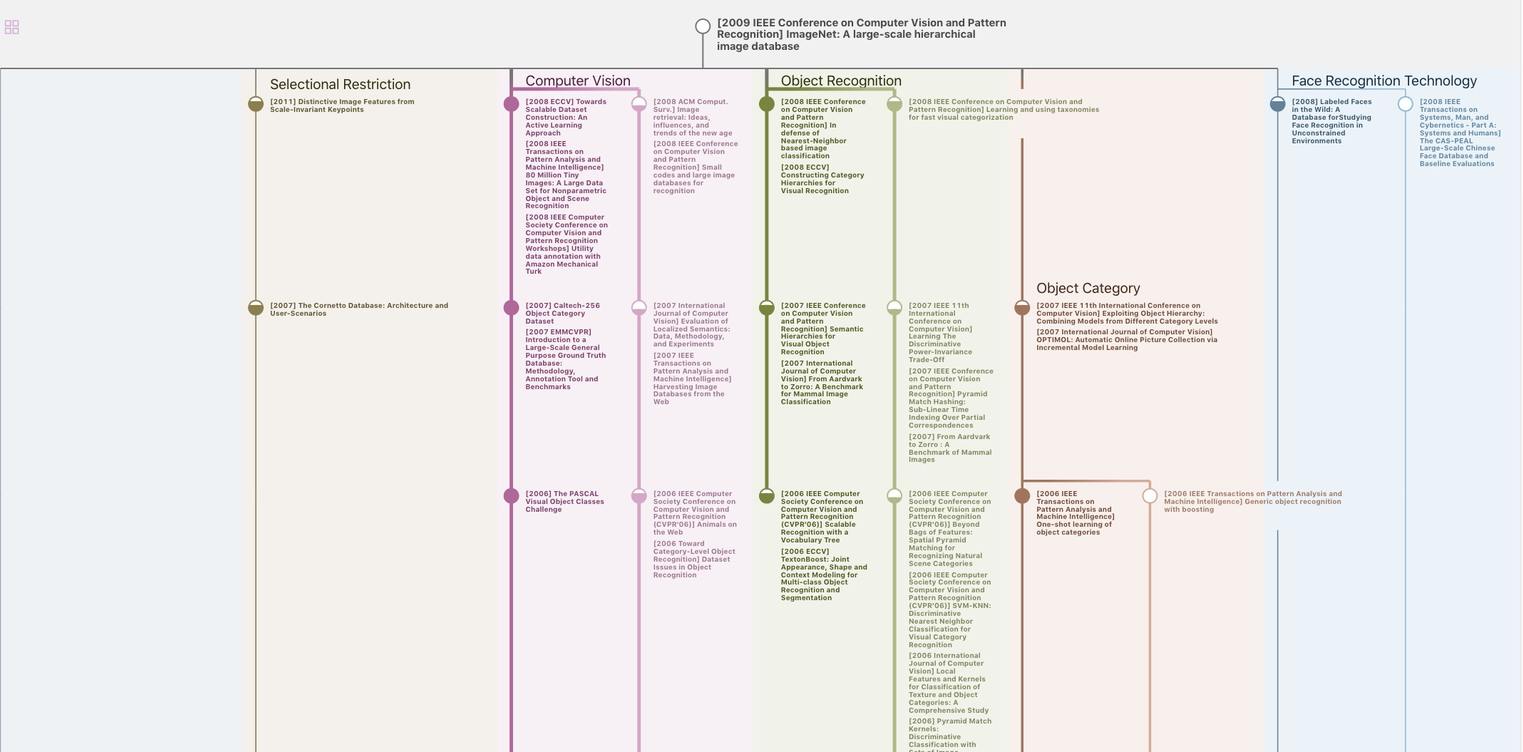
生成溯源树,研究论文发展脉络
Chat Paper
正在生成论文摘要