An Improved Online Reduction from PAC Learning to Mistake-Bounded Learning.
SOSA(2023)
摘要
A basic result in learning theory is that mistake-bounded learnability implies PAC learnability. It was shown in [Lit89] that, if a problem can be learned with M mistakes, it can be (ε, δ)-PAC-learned from samples. However, this reduction needs to store either samples or O(M) hypotheses. A different reduction, in [KLPV87], only needs to store O(1) samples and hypotheses but was only shown to work with samples.We give a refined analysis of the KLPV reduction, showing that it only uses samples with probability 1 - M-O(1). This gives the optimal sample complexity with only O(1) space overhead, for δ > M-O(1).
更多查看译文
关键词
pac learning,improved online reduction,mistake-bounded
AI 理解论文
溯源树
样例
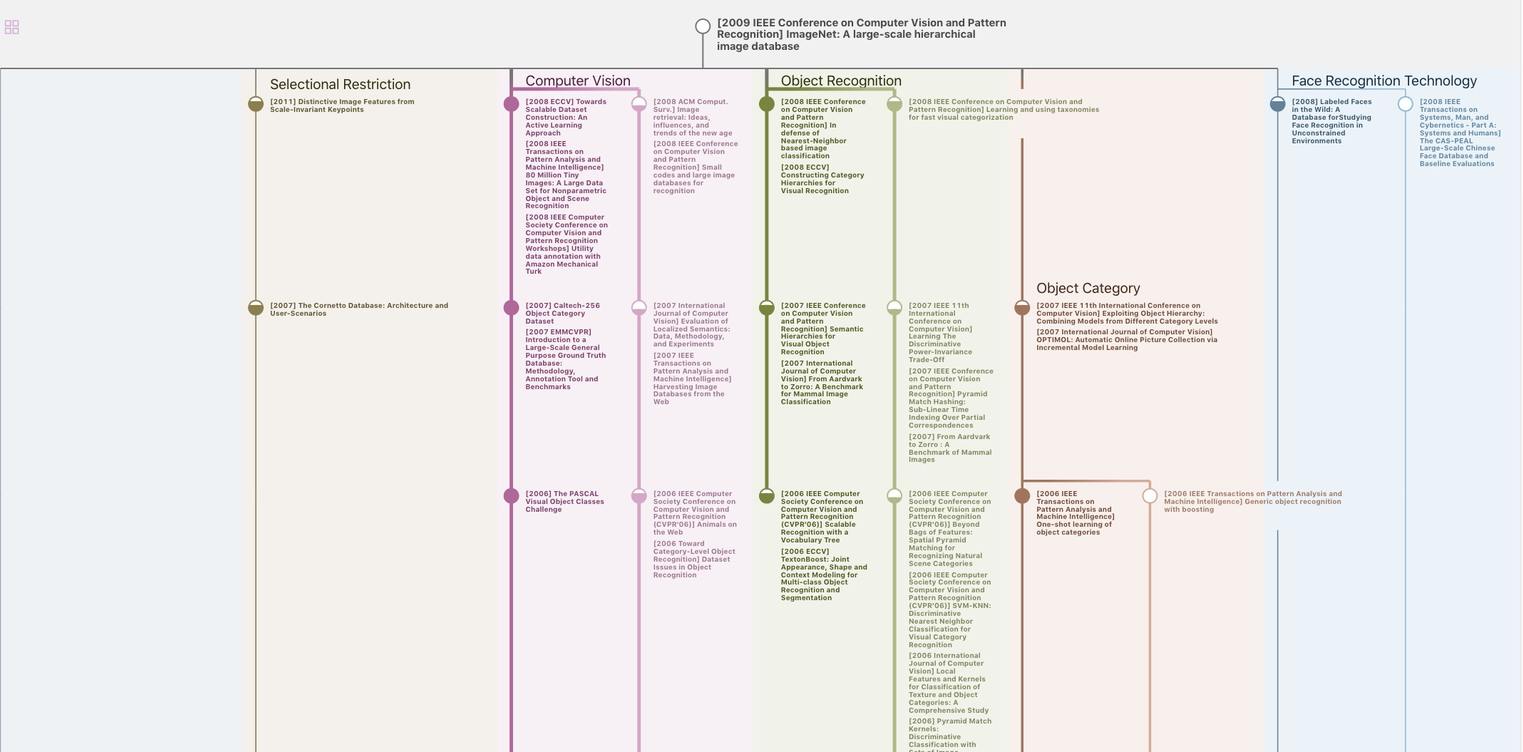
生成溯源树,研究论文发展脉络
Chat Paper
正在生成论文摘要