Mitigating robust overfitting via self-residual-calibration regularization.
Artif. Intell.(2023)
摘要
Overfitting in adversarial training has attracted the interest of researchers in the commu-nity of artificial intelligence and machine learning in recent years. To address this issue, in this paper we begin by evaluating the defense performances of several calibration methods on various robust models. Our analysis and experiments reveal two intriguing properties: 1) a well-calibrated robust model is decreasing the confidence of robust model; 2) there is a trade-off between the confidences of natural and adversarial images. These new prop-erties offer a straightforward insight into designing a simple but effective regularization, called Self-Residual-Calibration (SRC). The proposed SRC calculates the absolute residual between adversarial and natural logit features corresponding to the ground-truth labels. Furthermore, we utilize the pinball loss to minimize the quantile residual between them, resulting in more robust regularization. Extensive experiments indicate that our SRC can effectively mitigate the overfitting problem while improving the robustness of state-of-the-art models. Importantly, SRC is complementary to various regularization methods. When combined with them, we are capable of achieving the top-rank performance on the Au-toAttack benchmark leaderboard.(c) 2023 Elsevier B.V. All rights reserved.
更多查看译文
关键词
Adversarial training,Adversarial defense,Robust overfitting,Self -residual -calibration,Regularization
AI 理解论文
溯源树
样例
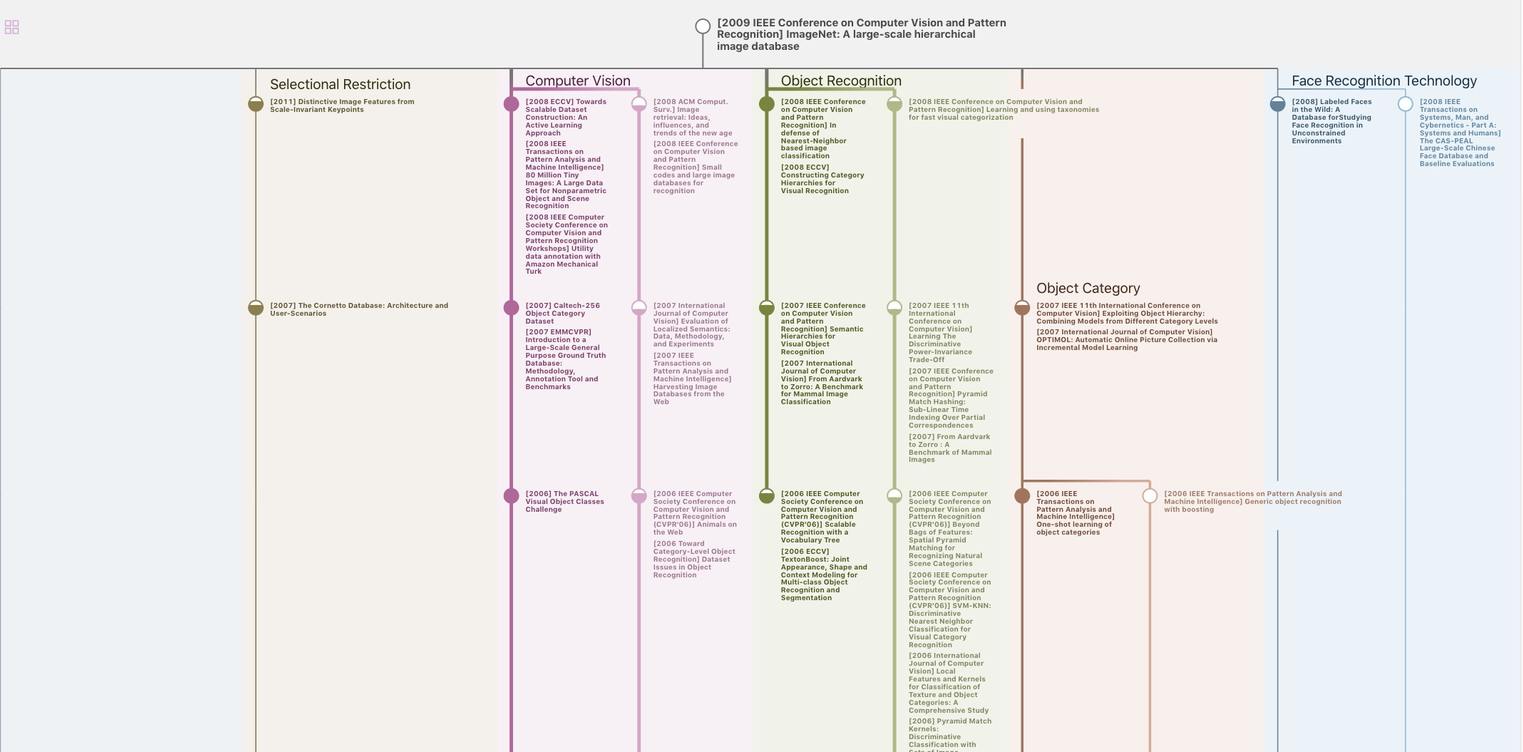
生成溯源树,研究论文发展脉络
Chat Paper
正在生成论文摘要