PigSNIPE: Scalable Neuroimaging Processing Engine for Minipig MRI.
Algorithms(2023)
摘要
Translation of basic animal research to find effective methods of diagnosing and treating human neurological disorders requires parallel analysis infrastructures. Small animals such as mice provide exploratory animal disease models. However, many interventions developed using small animal models fail to translate to human use due to physical or biological differences. Recently, large-animal minipigs have emerged in neuroscience due to both their brain similarity and economic advantages. Medical image processing is a crucial part of research, as it allows researchers to monitor their experiments and understand disease development. By pairing four reinforcement learning models and five deep learning UNet segmentation models with existing algorithms, we developed PigSNIPE, a pipeline for the automated handling, processing, and analyzing of large-scale data sets of minipig MR images. PigSNIPE allows for image registration, AC-PC alignment, detection of 19 anatomical landmarks, skull stripping, brainmask and intracranial volume segmentation (DICE 0.98), tissue segmentation (DICE 0.82), and caudate-putamen brain segmentation (DICE 0.8) in under two minutes. To the best of our knowledge, this is the first automated pipeline tool aimed at large animal images, which can significantly reduce the time and resources needed for analyzing minipig neuroimages.
更多查看译文
关键词
minipig,brain,segmentation,landmarks,image processing,deep learning,pig
AI 理解论文
溯源树
样例
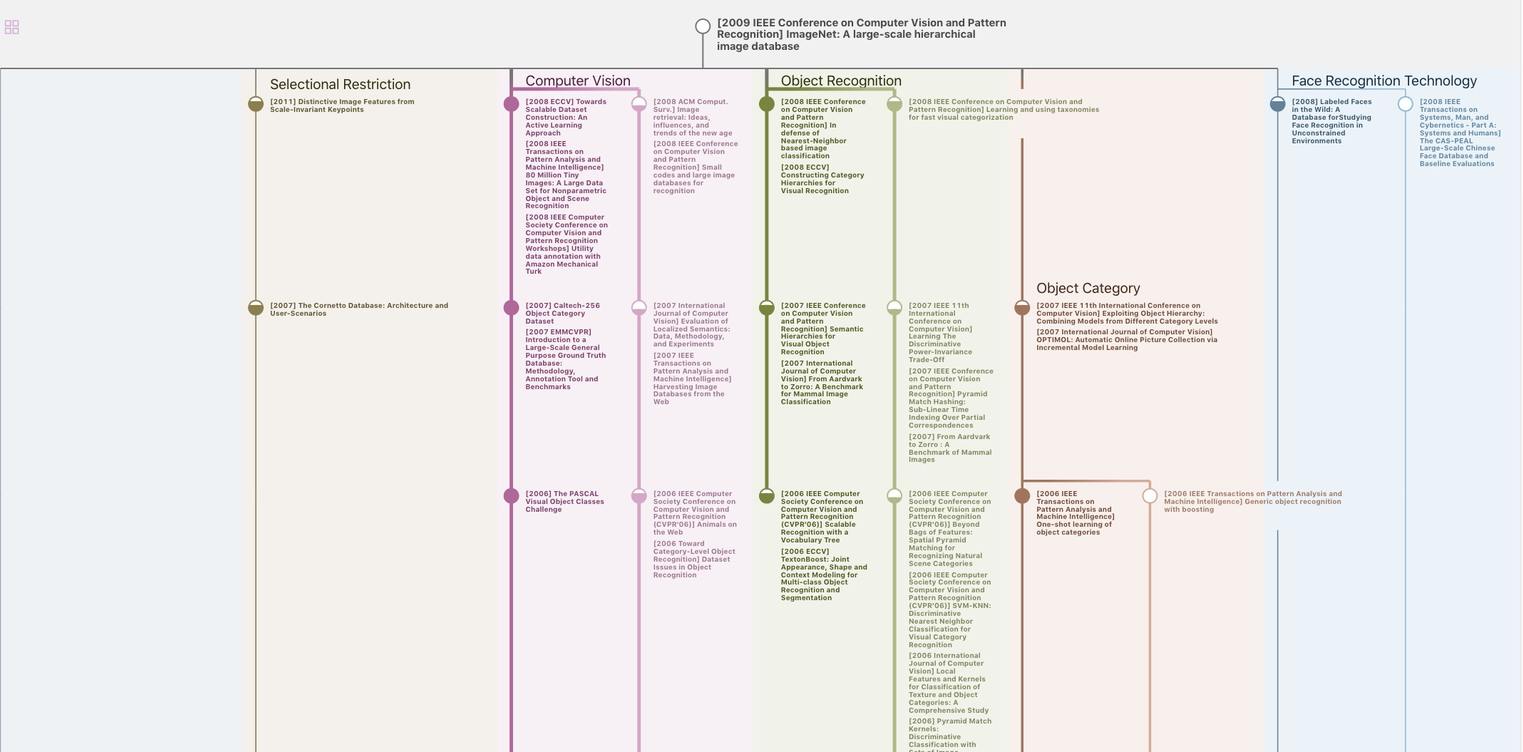
生成溯源树,研究论文发展脉络
Chat Paper
正在生成论文摘要