SECS: An effective CNN joint construction strategy for breast cancer histopathological image classification.
J. King Saud Univ. Comput. Inf. Sci.(2023)
摘要
Breast cancer is one of the most prevalent cancers in women. Reliable pathology identification can help histopathologists make accurate diagnosis of breast cancer but require specialized histopathological knowledge and a significant amount of manpower and medical resources. In this study, we fuse the coor-dinated attention mechanism to enhance the image texture analysis capability of the DenseNet, and build the CA-BreastNet model to classify microscopic histopathological images of specific types of breast can-cers in the BreakHis dataset. More crucially, convolutional decision trees based on the specialized enhanced classifying strategy(SECS) are built to increase the overall accuracy of the network by reducing the model's accuracy restriction imposed by dataset structures. The related experimental results show that our network has strong performance and the SECS offers researchers reliable and effective perfor-mance enhancement guidelines. The accuracy of the convolutional decision trees reaches 99.75% for bin-ary classification and 95.69% for eight-class classification, which means our model and strategy will be useful in the field of automatic diagnosis of breast cancer.(c) 2023 The Authors. Published by Elsevier B.V. on behalf of King Saud University. This is an open access article under the CC BY-NC-ND license (http://creativecommons.org/licenses/by-nc-nd/4.0/).
更多查看译文
关键词
Microscopy,Attention mechanism,Breast cancer,Histopathological images,Deep learning,SECS,HIs,SEC,CDT,ESC
AI 理解论文
溯源树
样例
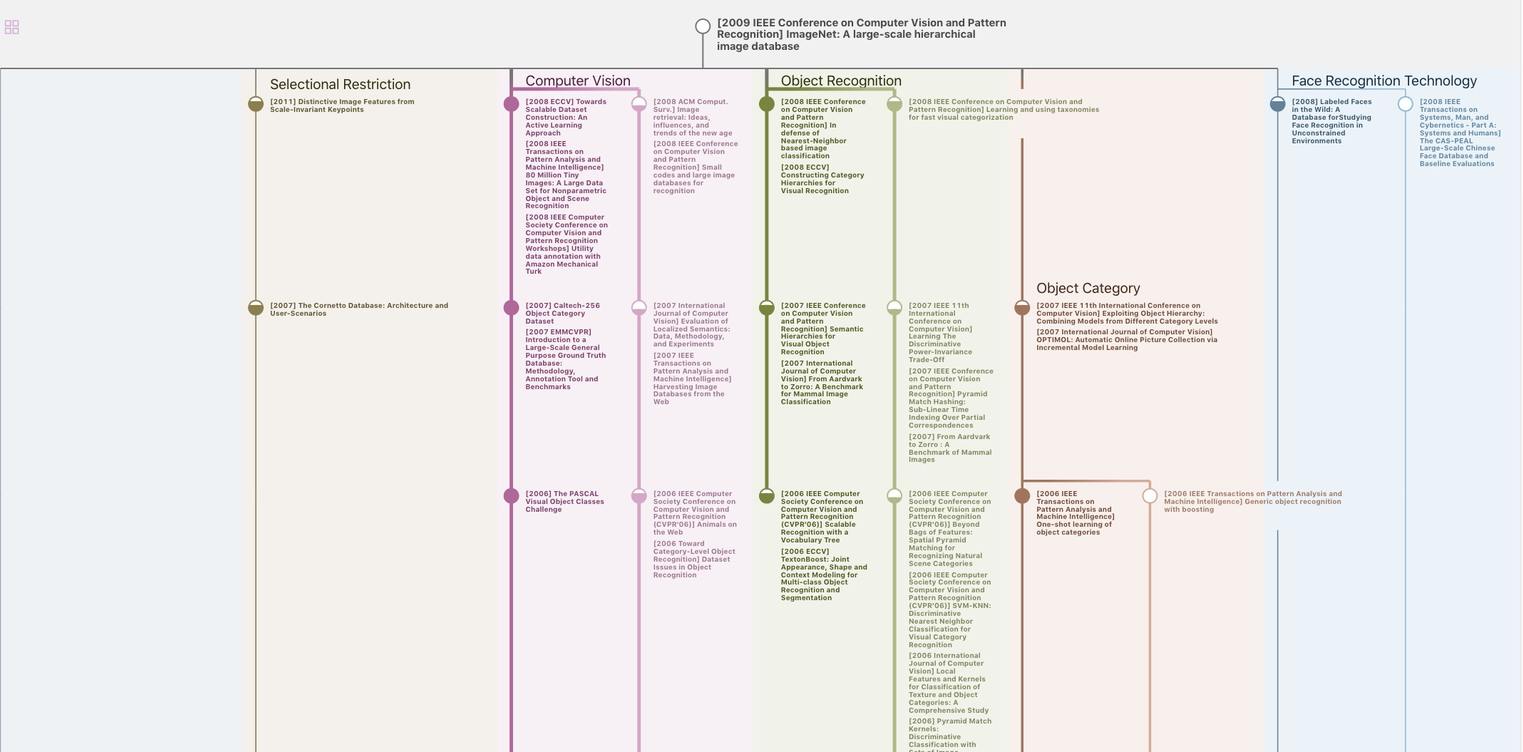
生成溯源树,研究论文发展脉络
Chat Paper
正在生成论文摘要