Outer Product Enhanced Embedding Information for Recommendation.
J. Inf. Sci. Eng.(2023)
摘要
In recommendation model, there are two critical factors that determine its performance: how to accurately extract the latent factors of users and items, and how to model their interactions. Most existing approaches represent users and items as low dimensional vectors (embeddings) and model interactions through the inner product. They ignore the paired correlation and fail to model the comprehensive correlations among latent features, making the model unable to accomplish high performance. In this paper, we propose to construct correlation matrix to enhance embedding information for recommendation. Specifically, we capture the latent factors of users and items accurately through the aggregation operation and attention network, and construct the correlation matrix between embeddings through the outer product. We propose a self-attention learning network to learn the local and global dependencies between embedded dimensions, enhance important information and eliminate noise interference. Experimental studies on four real datasets show that our method is superior to some of the most advanced methods.
更多查看译文
关键词
recommender system, matrix factorization, outer product, self-attention mechanism, correlation matrix
AI 理解论文
溯源树
样例
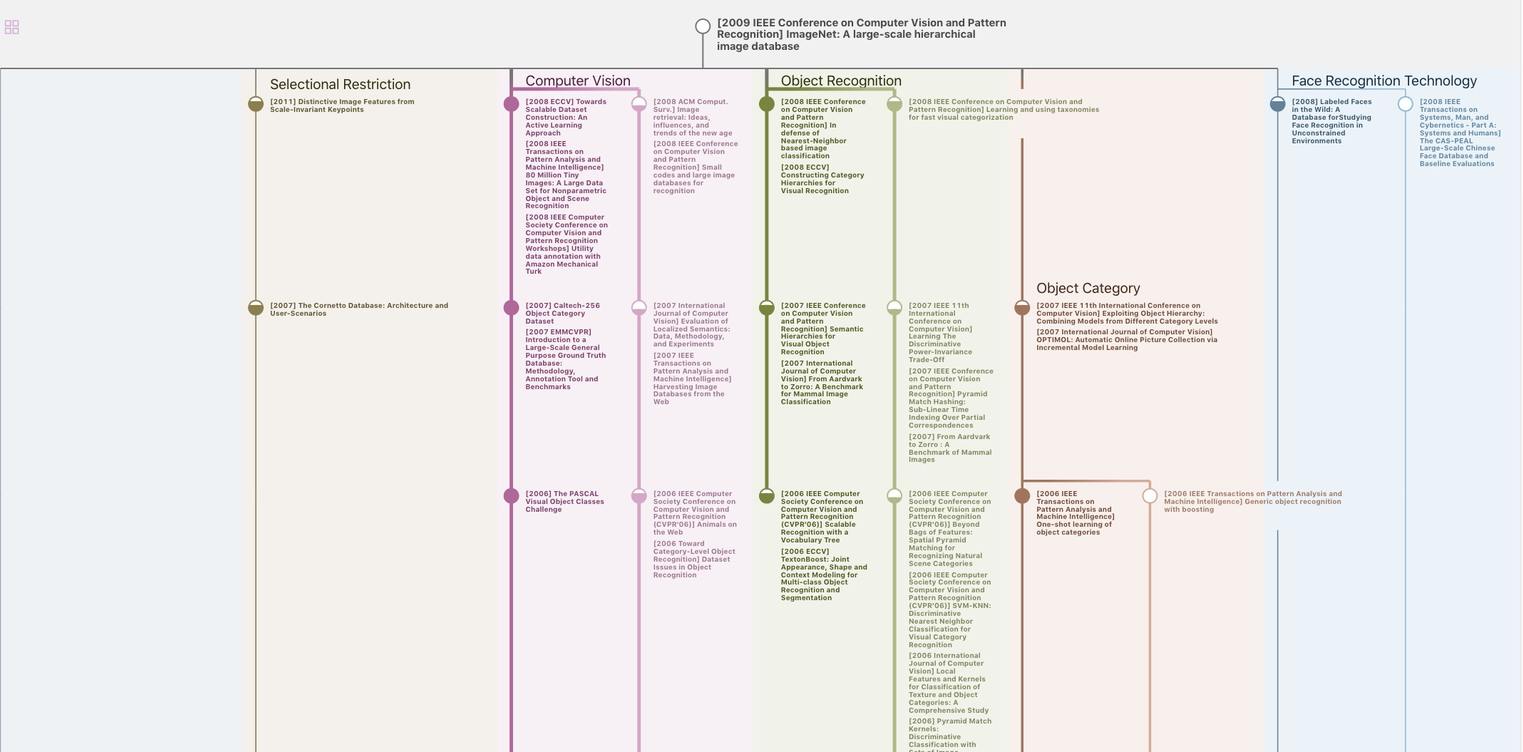
生成溯源树,研究论文发展脉络
Chat Paper
正在生成论文摘要