Risk-Informed Condition Evaluation of Solar-centered Energy Generation and Distribution Networks through Bayesian Learning and Inference.
ISGT(2023)
摘要
We develop a methodology based on Bayesian inference over Probabilistic Graphical Models (PGMs) to understand and quantify risk in solar-centered grids using targeted measurements and learned system behavior. Being non-prescriptive but, rather, able to infer system behavior and, ultimately, address risk queries from data, our machine learning-type paradigm is tailored for diverse topologies and threat scenarios often associated with distributed energy generation and photovoltaic distributed energy resources (PV-DERs) in particular. We describe algorithmic processes for: (i) learning the structure of PGMs that result from attack-prone PV-DER-proliferated distribution systems, (ii) quantifying cause-effect relationships, and (iii) evaluating risk queries based on diverse evidence. The contributions are illustrated on a residential grid subject to output impairment attacks on its PV-DER infrastructure.
更多查看译文
关键词
Photovoltaic systems,Power grids,Risk analysis,Probabilistic graphical models,Bayes methods,Machine learning
AI 理解论文
溯源树
样例
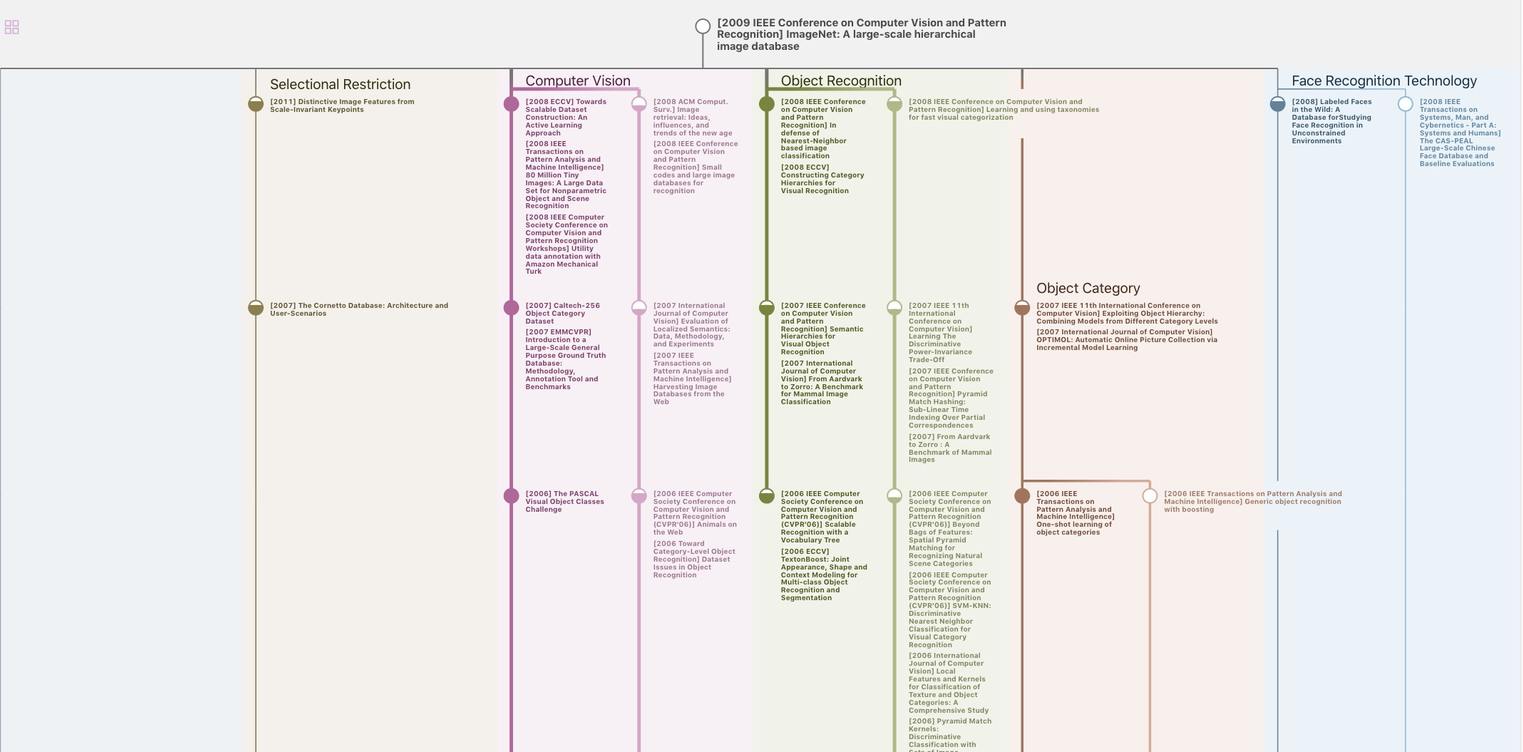
生成溯源树,研究论文发展脉络
Chat Paper
正在生成论文摘要