HGRBOL2: Human gait recognition for biometric application using Bayesian optimization and extreme learning machine
Future Gener. Comput. Syst.(2023)
摘要
The goal of gait recognition is to identify a person from a distance based on their walking style using a visual camera. However, the covariates such as a walk with carrying a bag and a change in clothes impact the recognition accuracy. This paper proposed a framework for human gait recognition based on deep learning and Bayesian optimization. The proposed framework includes both sequential and parallel steps. In the first step, optical flow-based motion regions are extracted and utilized to train the fine-tuned EfficentNet-B0 deep model. Simultaneously, we proposed a video frame enhancement technique. The same model is also separately trained on enhanced video frames. Instead of selecting static hyperparameters during the training process, Bayesian Optimization is employed in this work. Following that, two models have obtained: the motion frames model and the original frames model. Features are extracted from both models and fused using a proposed parallel approach named-Sq-Parallel Fusion (SqPF). The Tiger optimization algorithm was then improved and named Entropy controlled Tiger optimization (EVcTO). The best features are finally classified using an extreme learning machine (ELM) classifier. The experiment was carried out on two publicly available datasets, CASIA B and CASIA C, and yielded average accuracy of 92.04 and 94.97%, respectively. The proposed framework outperforms other deep learning-based networks in terms of accuracy. (c) 2023 Elsevier B.V. All rights reserved.
更多查看译文
关键词
Gait recognition,Bayesian optimization,Deep learning,Feature selection,Extreme learning machine
AI 理解论文
溯源树
样例
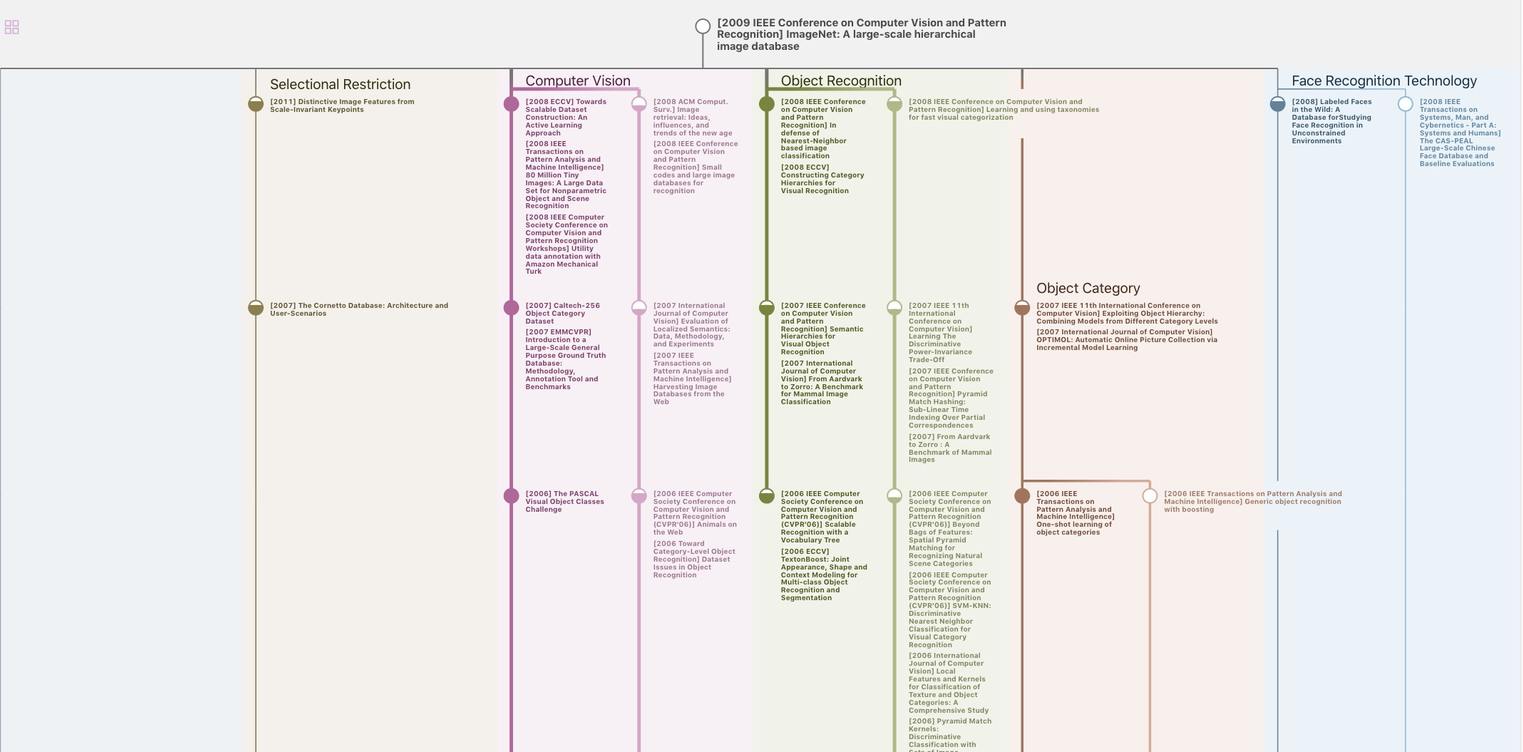
生成溯源树,研究论文发展脉络
Chat Paper
正在生成论文摘要