Adaptive Self-Supervised SAR Image Registration With Modifications of Alignment Transformation.
IEEE Trans. Geosci. Remote. Sens.(2023)
摘要
Considering that deep learning achieves the prominent performance, it has been applied to synthetic aperture radar (SAR) image registration to improve the registration accuracy. In most methods, a deep registration model is constructed to classify matched points and unmatched points, in which SAR image registration is regarded as a supervised two-classification problem. However, it is difficult to annotate massive matched points manually in practice, which limits the performance of deep networks. Besides, inevitable differences among SAR images easily cause that some training and testing samples are inconsistent, which probably brings negative effects for training a robust registration model. To address these problems, we propose an adaptive self-supervised SAR image registration method, where SAR image registration is regarded as a self-supervised task rather than the supervised two-classification task. Inspired by self-supervised learning, we consider each point on SAR images as a category-independent instance, which mitigates the requirement of manual annotations. Based on key points from images, a self-supervised model is constructed to explore the latent feature of each key point, and then, pairs of match points are sought via evaluating similarities among key points and used to calculate the alignment transformation matrix. Meanwhile, to enhance the consistency of samples, we design a new strategy that constructs multiscale samples by transforming key points from one image into another, which avoids inevitable diversities between two images effectively. In particular, the constructed samples feeding to the self-supervised model are adaptively updated with the modification of the transformation matrix in iterations. Moreover, the similarity of maximal public areas (MPAS) indicator is proposed to assist in estimating the transformation. Finally, experimental results illustrate that the proposed method achieves more accurate registrations than other compared methods.
更多查看译文
关键词
sar image registration,alignment transformation,self-supervised
AI 理解论文
溯源树
样例
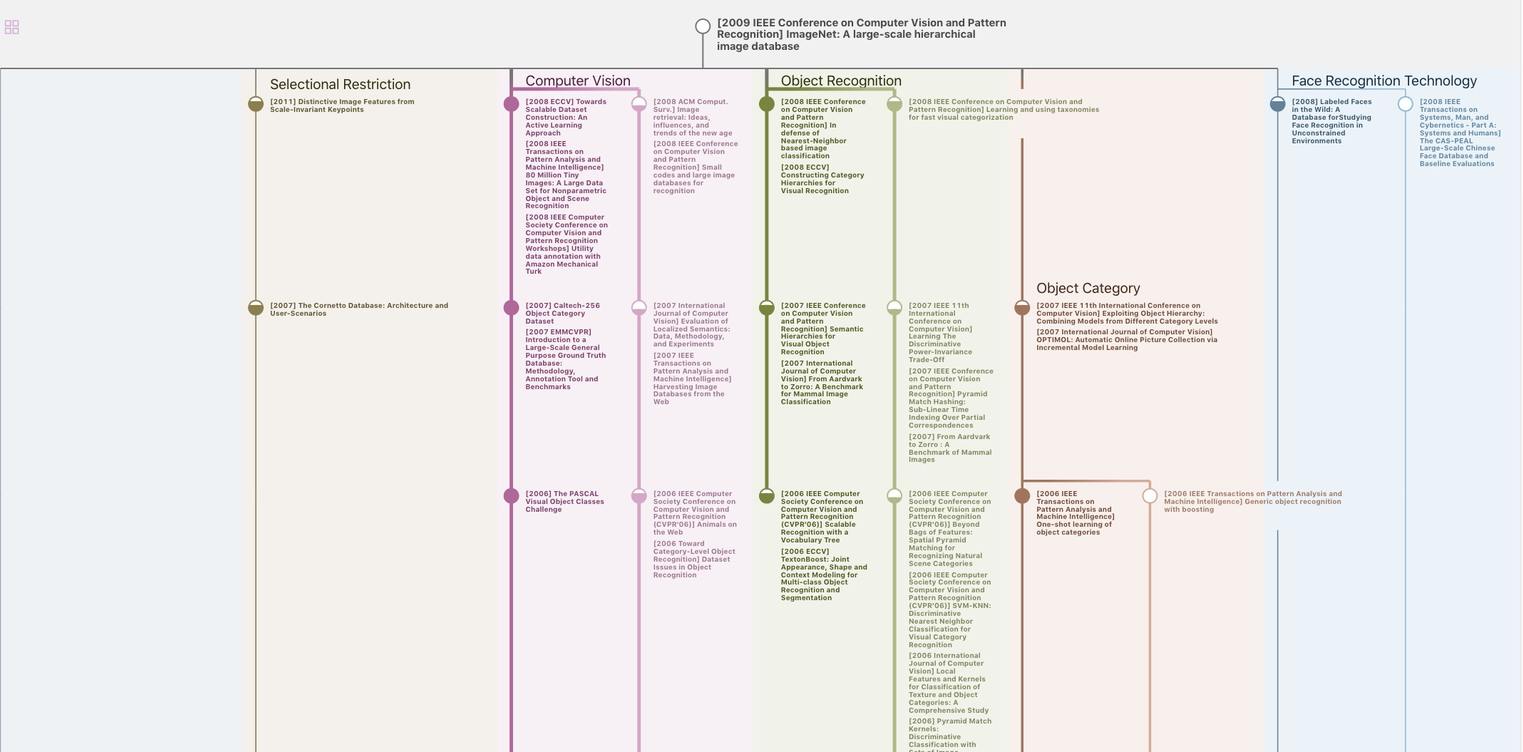
生成溯源树,研究论文发展脉络
Chat Paper
正在生成论文摘要