Deep Learning for 3-D Magnetic Inversion
IEEE Trans. Geosci. Remote. Sens.(2023)
摘要
The difficulty of 3-D magnetic inversion is to use 2-D magnetic anomaly data to obtain 3-D magnetic susceptibility structure. The contribution of the underground medium to the magnetic anomaly decreases rapidly with the increase of the depth, which leads to the rapid attenuation of the inversion resolution with the depth. In this article, artificial intelligence (AI) technology is applied to 3-D magnetic inversion to predict the susceptibility model corresponding to magnetic anomaly. The inversion network built in this article uses the method of down-sampling in the encoder to increase the receptive field and realize the feature extraction of magnetic anomaly data. In the decoder, attention fusion modules are added to fuse feature maps from different sources. Finally, we added a 3-D refiner behind the decoder. The 3-D refiner converts the 2-D feature map from the decoder into 3-D data. Based on the typical complex medium theory, this article constructs a diverse sample set of complex 3-D susceptibility models. The inversion experiment of synthetic data verifies the feasibility and versatility of the proposed network. Compared with the other methods, the distribution of susceptibility prediction obtained by our method is more accurate and more reliable in determining the magnetic body boundary. In the field example of Jinchuan Copper-nickel sulfide deposit in China, the network constructed in this article can achieve high-precision 3-D underground susceptibility imaging in this area. The susceptibility distribution is in good agreement with the borehole data and the proved deposit distribution.
更多查看译文
关键词
3-D magnetic inversion,artificial intelligence (AI),complex medium,high-precision
AI 理解论文
溯源树
样例
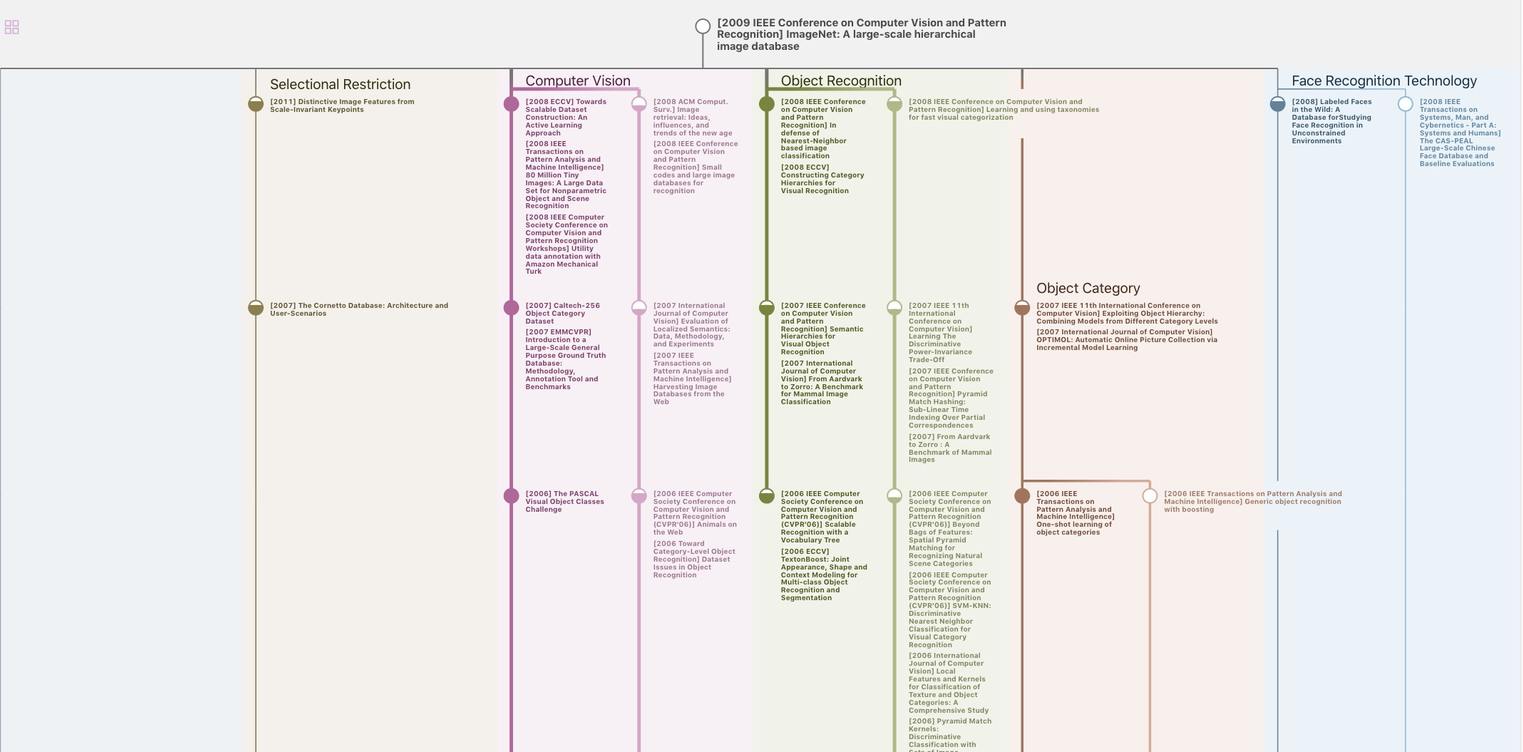
生成溯源树,研究论文发展脉络
Chat Paper
正在生成论文摘要