Ionospheric Tomography: A Compressed Sensing Technique Based on Dictionary Learning.
IEEE Trans. Geosci. Remote. Sens.(2023)
摘要
Global Navigation Satellite System (GNSS) observation insufficiency limits the development of the voxel-based computerized ionospheric tomography (CIT) technique. Electron densities of voxels without observation cannot be accurately estimated by the commonly used algebraic reconstruction techniques. In this study, we proposed a compressed sensing technique (CST) based on dictionary learning (DL) for ionospheric tomography. Specifically, the K-singular value decomposition (K-SVD) algorithm was used for DL based on training sets that are derived from the NeQuick model, hereafter referred to as the CST_NeQuick algorithm. K-SVD uses the orthogonal matching pursuit (OMP) for sparse coding and the SVD approach for dictionary updating. Both simulations and real experiments demonstrated the feasibility and superiority of the CST algorithm when compared to the widely used multiplicative algebraic reconstruction technique (MART). It was found that the CST_NeQuick algorithm's tomographic performances were mostly superior to those of the MART algorithm in comparison with the independent slant total electron content (STEC) references. Another CST-based tomographic experiment was performed by using the MART-based solutions for DL, hereafter referred to as the CST_MART algorithm. It showed that the CST_MART algorithm can reduce the average root mean square (rms) of the CIT-derived STEC by 37.3% and 20.2%, respectively, when compared to the MART and CST_NeQuick algorithms. Besides, the CST_MART algorithm's electron density profiles (EDPs) also showed more agreement with the EDPs that were derived from radio occultation data.
更多查看译文
关键词
Compressed sensing,computerized ionospheric tomography (CIT),dictionary learning (DL),Global Navigation Satellite System (GNSS),multiplicative algebraic reconstruction technique (mart)
AI 理解论文
溯源树
样例
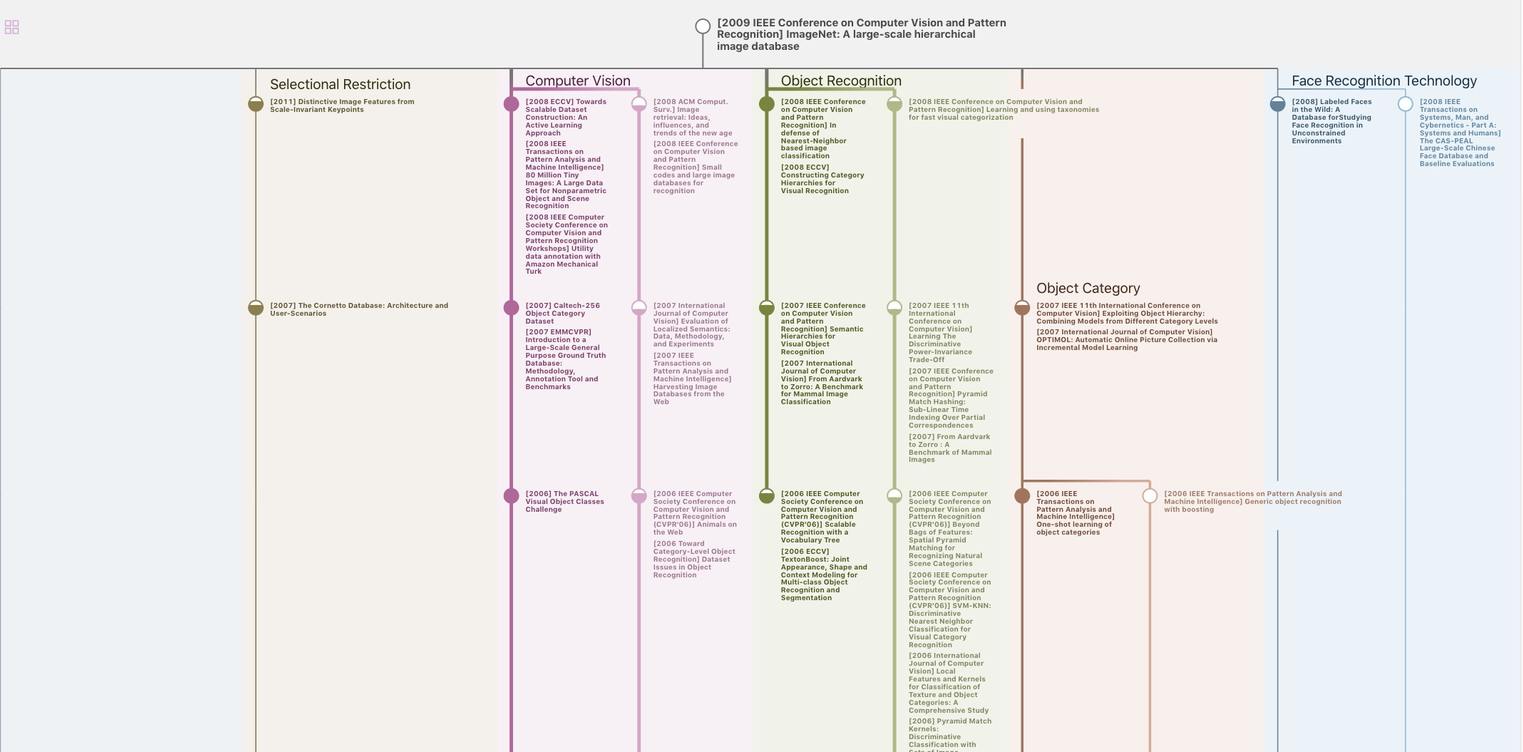
生成溯源树,研究论文发展脉络
Chat Paper
正在生成论文摘要