Regulated Subspace Projection Based Local Model Update Compression for Communication-Efficient Federated Learning.
IEEE J. Sel. Areas Commun.(2023)
摘要
Despite high utility in distributed networks, federated learning entails enormous communication overhead due to the requirement of trained model exchange at every global iteration. When the communication resources are limited, as in wireless environments, learning performance can be severely degraded by the communication overhead. On this account, communication efficiency is one of the primary concerns in federated learning. In this paper, we put forth a communication-efficient federated learning system based on the projection of local model updates. Leveraging the correlation of consecutive local model updates, we devise a novel local model update compression scheme based on the projection onto the selected subspace. Furthermore, to avoid error propagation over global iterations and thus improve learning performance, we also develop novel criteria for deciding whether to compress the local model updates or not. The convergence of the proposed algorithm is also mathematically proved by deriving an upper bound on the mean square error of the global parameter. The merits of the proposed algorithm over the state-of-the-art benchmark schemes are verified by various simulations.
更多查看译文
关键词
Federated learning,communication-efficient,compression,subspace selection,projection
AI 理解论文
溯源树
样例
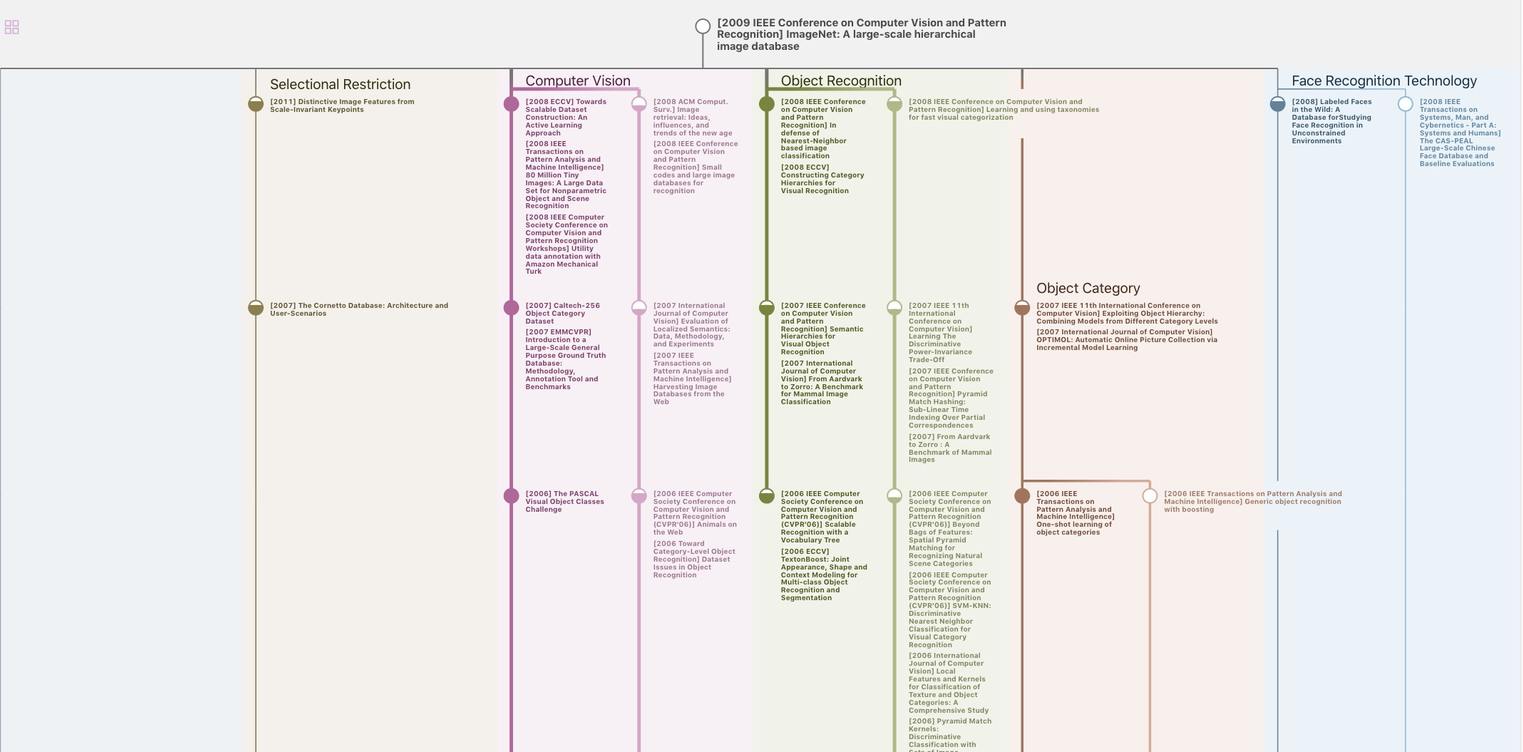
生成溯源树,研究论文发展脉络
Chat Paper
正在生成论文摘要