Penalized Whittle likelihood for spatial data.
J. Multivar. Anal.(2023)
摘要
Inference for spatial data is challenging because fitting an appropriate parametric model is often difficult. The penalized likelihood-type approach has been successfully developed for various nonparametric function estimation problems in time series analysis. However, it has not been well developed in spatial analysis. In this paper, a penalized Whittle likelihood approach is developed for nonparametric estimation of spectral density functions for regularly spaced spatial data. In particular, the estimated spectral density is the minimizer of a criterion which is developed based on the Whittle likelihood and a penalty for roughness. This approach aggregates several popular nonparametric density estimation methods into a coherent framework. Asymptotic properties of the proposed estimator are derived under mild assumptions without assuming Gaussianity. In addition, a computationally efficient method is developed to optimize the penalized likelihood function. Simulation results and real data examples are also provided to illustrate the finite sample performances of the methodology.
更多查看译文
关键词
primary,secondary
AI 理解论文
溯源树
样例
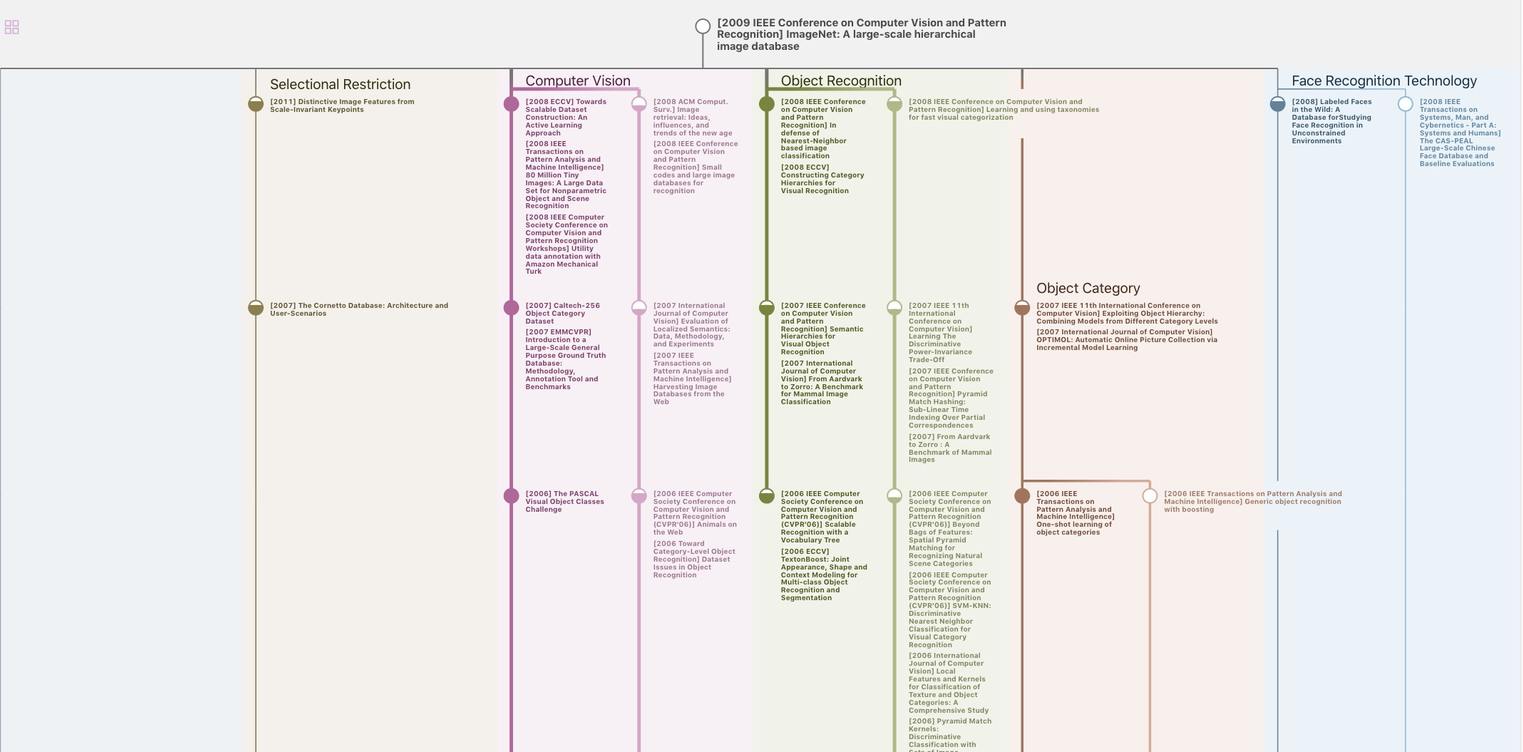
生成溯源树,研究论文发展脉络
Chat Paper
正在生成论文摘要