Deep Reinforcement Learning-based Uplink Power Control in Cell-Free Massive MIMO.
CCNC(2023)
摘要
This paper addresses the power control problem of a cell-free uplink massive Multiple-Input Multiple-Output (MIMO) system with mobile users, aiming at global sum-rate maximization under individual user Quality of Service (QoS) constraints. To solve this problem, we propose a Deep Deter-ministic Policy Gradient (DDPG)-based power control algorithm, whose design is tailored given the static and mobile user cases, respectively. In particular, different partial state space designs are investigated for each mobility use case, so as to achieve the best tradeoff between network performance and required learning complexity. Numerical results validate the effectiveness of the proposed method, which outperforms benchmark schemes both in terms of sum-rate and number of QoS satisfied users. It is shown that it can combine the advantages of traditional uniform max power control and max-min power control schemes. Furthermore, the proposed method is flexible and adapts itself well to dynamic and mobile environments.
更多查看译文
关键词
Cell-free massive MIMO,DDPG,power control
AI 理解论文
溯源树
样例
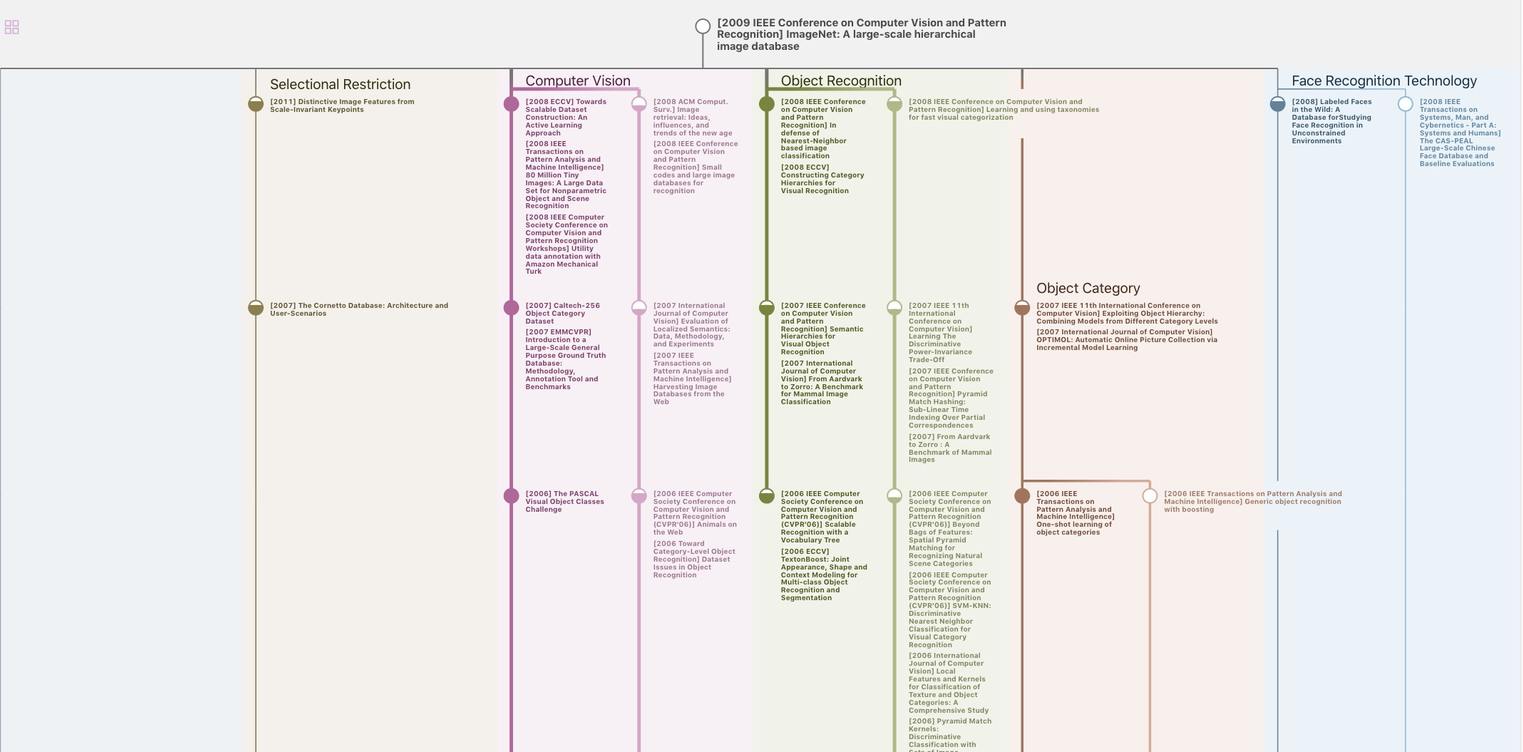
生成溯源树,研究论文发展脉络
Chat Paper
正在生成论文摘要