A Deep Q-Learning Connectivity-Aware Pheromone Mobility Model for Autonomous UAV Networks.
ICNC(2023)
摘要
Low size, weight, and power (SWaP) fixed-wing UAVs are used in many applications, including search and rescue, surveillance, and tracking. To carry out these operations efficiently, there is a need to develop scalable, decentralized autonomous UAV network architectures with high network connectivity. However, the area coverage and the network connectivity requirements exhibit a fundamental trade-off. In this paper, we design a connectivity-aware pheromone mobility model using a deep Q-learning policy (CAP-DQN). We use stigmergy-based digital pheromone maps along with distance-based local connectivity information to autonomously coordinate the UAV movements in order to improve its map coverage efficiency while maintaining high network connectivity. The DQN policy helps in tuning and improving the coverage and connectivity tradeoff. The proposed model facilitates efficient coverage and strong inter-UAV connectivity in autonomous UAV networks.
更多查看译文
关键词
Airborne network,UAV network,area coverage,network connectivity,reinforcement learning,deep Q-learning
AI 理解论文
溯源树
样例
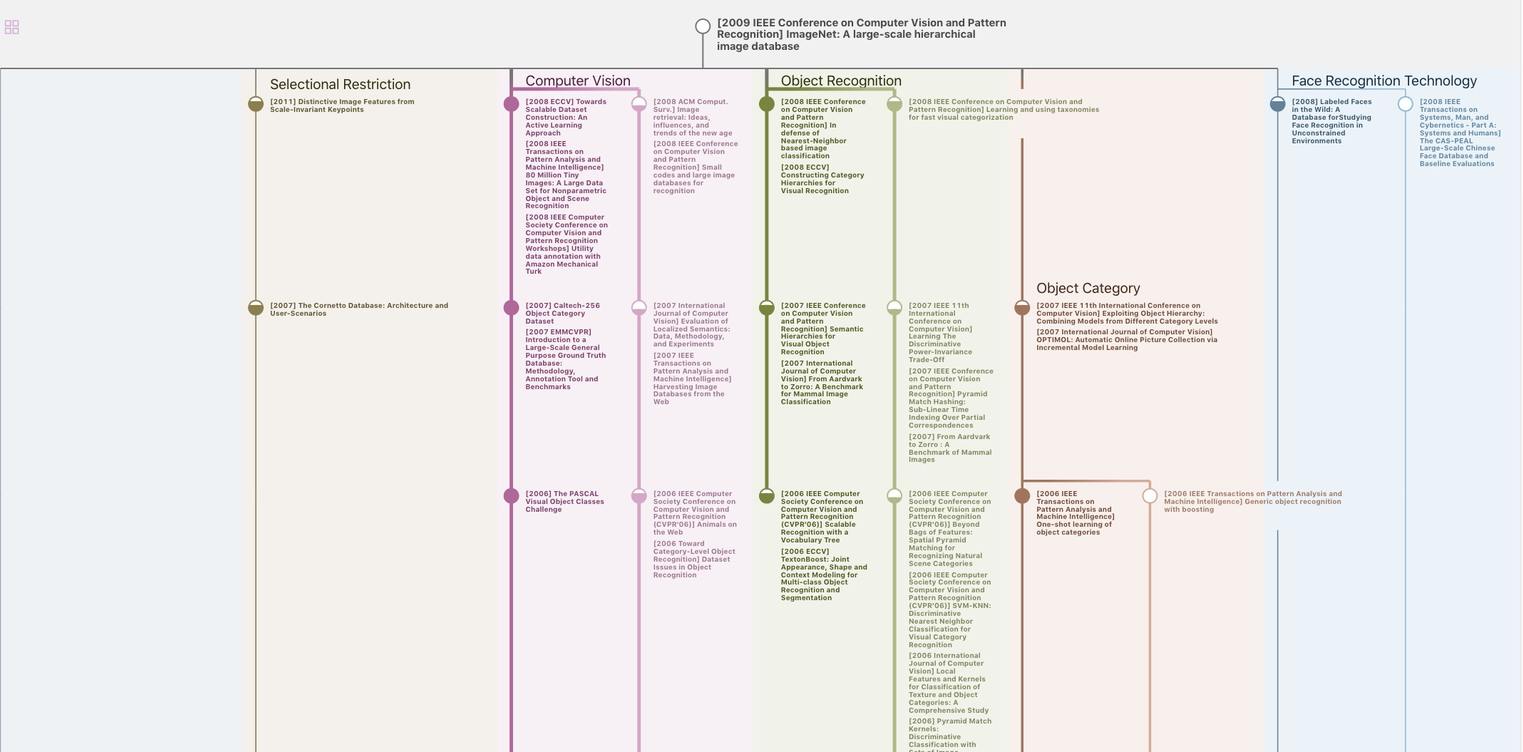
生成溯源树,研究论文发展脉络
Chat Paper
正在生成论文摘要