Hide & Seek: Traffic Matrix Completion and Inference Using Hidden Information.
CCNC(2023)
摘要
Traffic matrices are used for many network management operations, from planning to repairing. Despite years of research on the topic, their estimation and inference on the Internet are still challenging and error-prone. For example, missing values are unavoidable due to flaws in the measurement systems and possible failure in data collection systems. It is thus helpful for many network operators to recover the missing data from the partial direct measurements. Some existing matrix completion methods do not fully consider network traffic behavior and hidden traffic characteristics, showing the inability to adapt to multiple scenarios. Others instead make assumptions on the matrix structure that may be invalid or impractical, curtailing the applicability. In this paper, we propose Hide & Seek, a novel matrix completion and prediction algorithm based on a combination of generative autoencoders and Hidden Markov Models. We demonstrate with an extensive experimental evaluation on real-world datasets how our algorithm can accurately reconstruct missing values while predicting their short-term evolution.
更多查看译文
关键词
traffic matrix,machine learning,inference
AI 理解论文
溯源树
样例
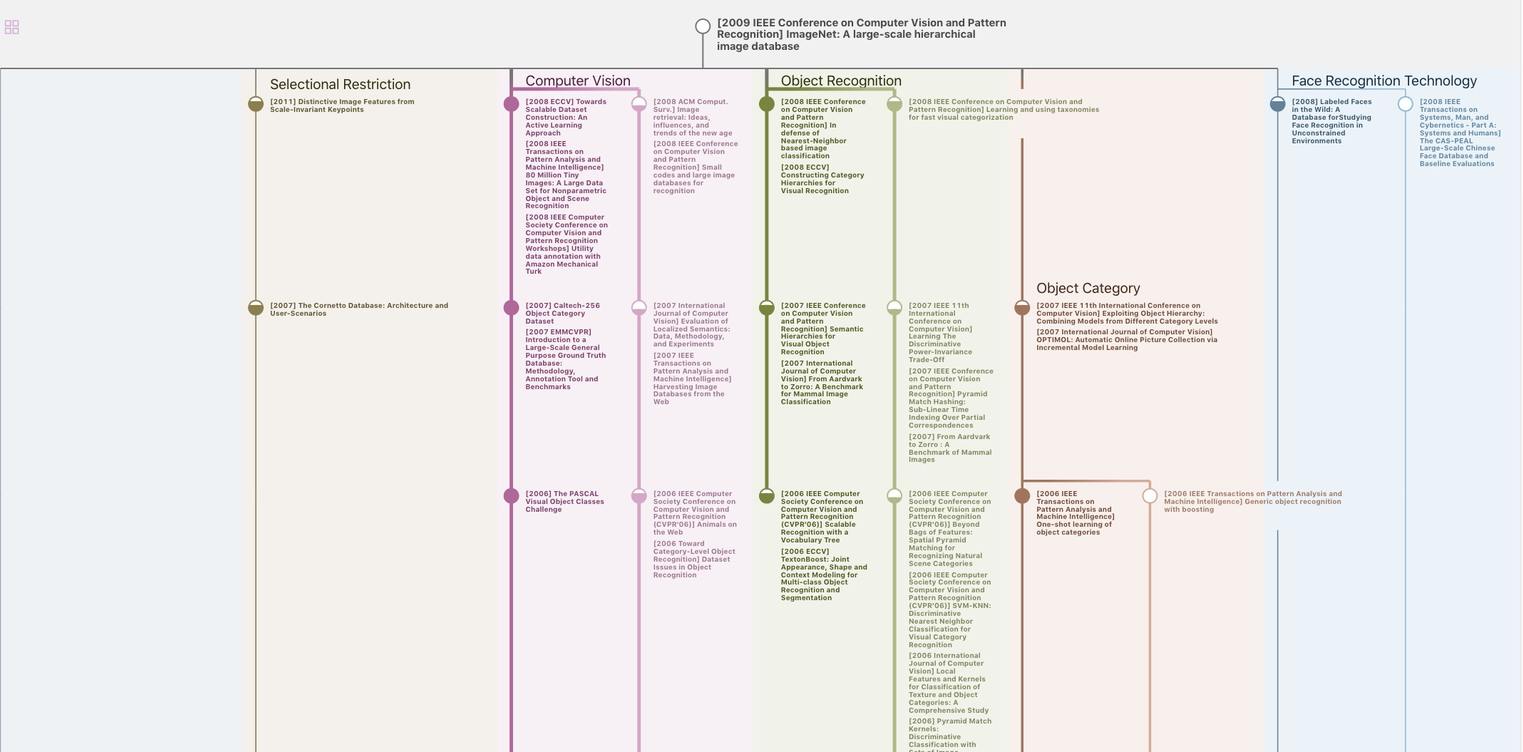
生成溯源树,研究论文发展脉络
Chat Paper
正在生成论文摘要