Implementation of Real-Time Adversarial Attacks on DNN-based Modulation Classifier.
ICNC(2023)
摘要
In this paper, we provide a hardware implementation for over-the-air (OTA) adversarial attack on a deep neural network (DNN)-based modulation classifiers. Although Automatic modulation classification (AMC) using the DNN-based method outperforms the traditional classification, it has been proven that the machine learning (ML) approaches lack robustness against adversarial attacks. Therefore, the adversarial attacks cause the loss of accuracy for the DNN-based AMC by injecting a well-designed perturbation to the wireless channels. The case study presented evaluates the adversarial attack performance and its effects on the accuracy of the DNN-classifier OTA using a universal software radio peripheral (USRP) B210. Firstly, we develop an intelligent AMC system using USRPs to classify four digitally modulated signals, namely, BPSK, QPSK, 8PSK, and 16QAM, in real-time. We consider a wireless communication system that consists of three software-defined radios (SDRs), namely, transmitter, receiver, and adversarial attack. While the Rx classifies the received signal, using a DNN-based classifier, the adversarial attack node intends to misclassify the DNN-based classifier by perturbing the input data of with an adversarial example. The developed adversarial node implements the Fast-Gradient Sign method (FGSM) to generate the needed perturbation. The results of the conducted experiment show that the DNN-based classifier achieves 97% accuracy in the absence of an adversarial node. However, after deploying the adversarial attack the classifier accuracy drops to 42%.
更多查看译文
关键词
Modulation classifications,DNN-based classifier,FSGM,USRPs,SDR.
AI 理解论文
溯源树
样例
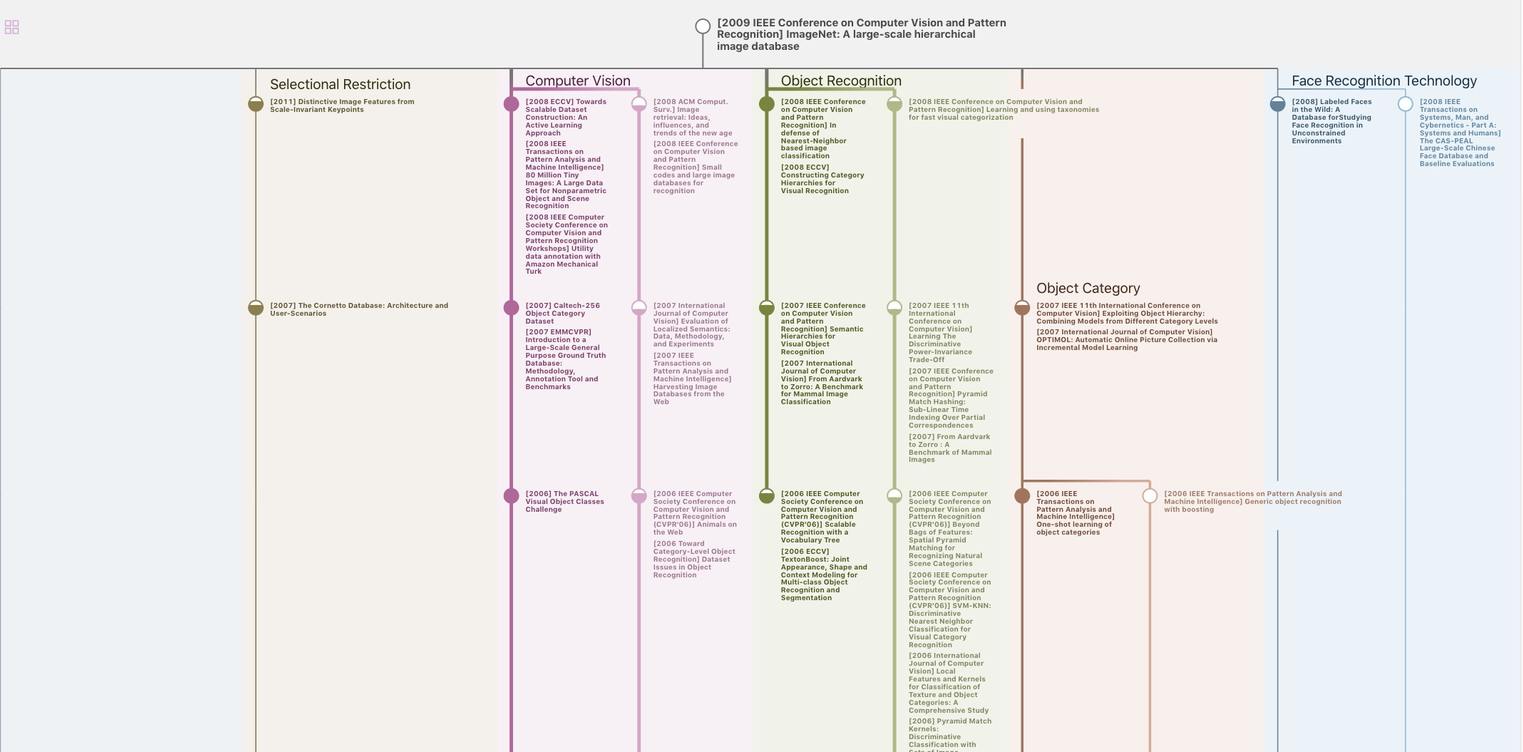
生成溯源树,研究论文发展脉络
Chat Paper
正在生成论文摘要