Robust Stochastic Principal Component Analysis via Barron Loss.
IEEECONF(2022)
摘要
Principal-Component Analysis (PCA) is a data analysis technique that models high-dimensional data by means of a lower-dimensional subspace. It has numerous applications in signal processing, machine learning, and pattern recognition. Despite its popularity, standard PCA is known to be sensitive to outliers, leading to degraded performance in the presence of corrupted data. In the era of big data, stochastic PCA algorithms are preferred due to their computational efficiency and ability to dynamically adapt to shifts in the subspace. However, most of the existing stochastic PCA algorithms are susceptible to corrupted data due to their reliance on the outlier-sensitive L2-norm. In this work, we propose a robust stochastic PCA algorithm that relies on the Barron loss. The proposed method is able to process streaming data with low computational cost, effectively perform subspace estimation, and track the subspace while also providing significant resistance to faulty/outlying data. Our numerical studies on synthetic and real-world data demonstrate the convergence and performance of the proposed method.
更多查看译文
关键词
Dynamic, Barron loss, outliers, PCA, robust, stochastic
AI 理解论文
溯源树
样例
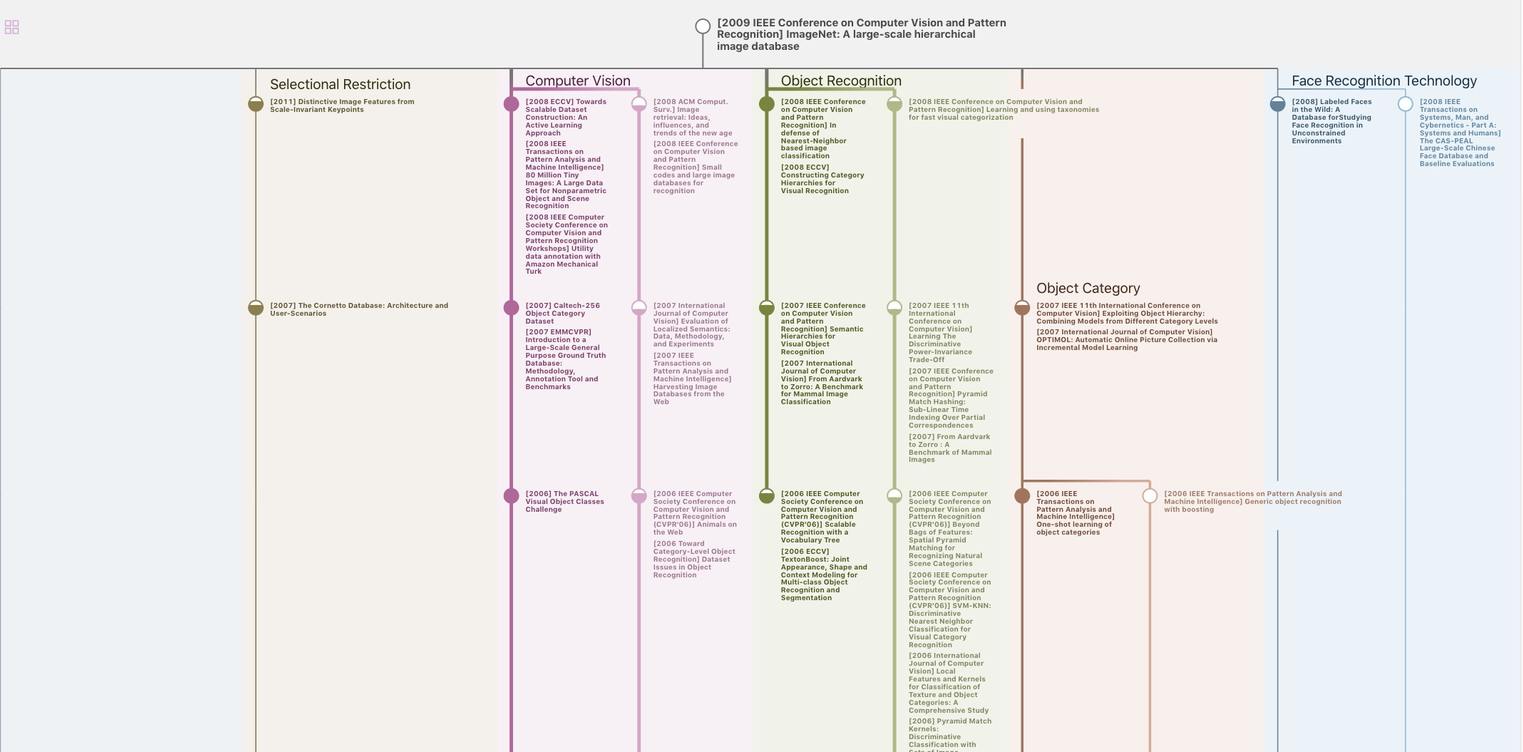
生成溯源树,研究论文发展脉络
Chat Paper
正在生成论文摘要