Deep Reinforcement Learning for Interference Management in Millimeter-Wave Networks.
IEEECONF(2022)
摘要
Interference is a long-lasting problem in cellular networks. As cell deployment becomes denser, interference becomes a major obstacle to improving network throughput. In this paper, we propose using deep reinforcement learning for interference management via joint beamforming and power control in multi-cell networks. The goal is to maximize the signal-to-interference-plus-noise ratio (SINR) in the dynamic environment of millimeter-wave multi-cell networks. The proposed algorithm works for an arbitrary number of cells to improve the network performance, measured by achievable SINR and sum-rate. Simulation results show that our algorithm performs significantly better than state-of-the-art and is close to the optimal scenario in terms of coverage and sum-rate performance.
更多查看译文
关键词
cell deployment,cellular networks,deep reinforcement learning,interference management,joint beamforming,long-lasting problem,millimeter-wave multicell networks,millimeter-wave networks,network performance,network throughput,power control,signal-to-interference-plus-noise ratio
AI 理解论文
溯源树
样例
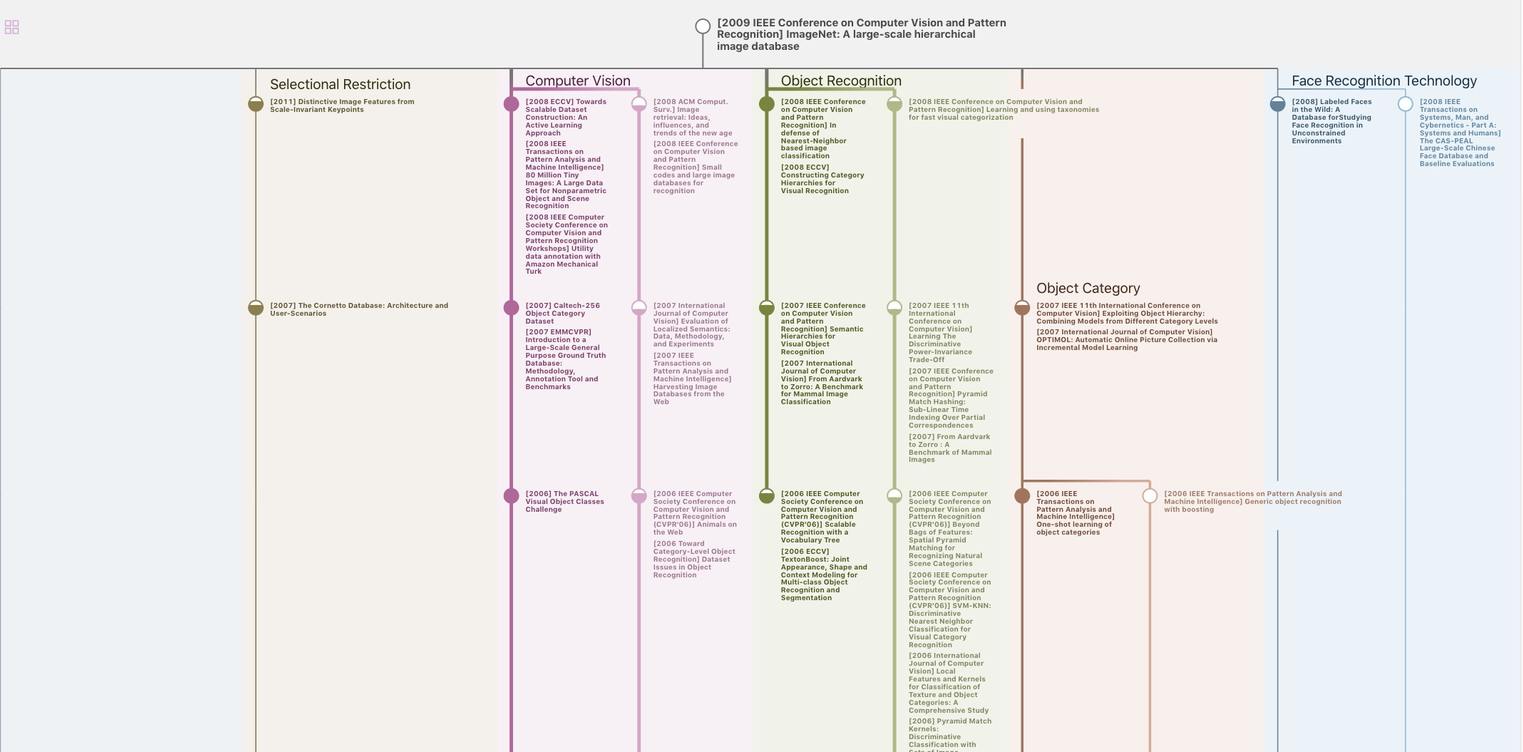
生成溯源树,研究论文发展脉络
Chat Paper
正在生成论文摘要