Contrasting Neural Click Models and Pointwise IPS Rankers.
ECIR (1)(2023)
摘要
Inverse-propensity scoring and neural click models are two popular methods for learning rankers from user clicks that are affected by position bias. Despite their prevalence, the two methodologies are rarely directly compared on equal footing. In this work, we focus on the pointwise learning setting to compare the theoretical differences of both approaches and present a thorough empirical comparison on the prevalent semi-synthetic evaluation setup in unbiased learning-to-rank. We show theoretically that neural click models, similarly to IPS rankers, optimize for the true document relevance when the position bias is known. However, our work also finds small but significant empirical differences between both approaches indicating that neural click models might be affected by position bias when learning from shared, sometimes conflicting, features instead of treating each document separately.
更多查看译文
AI 理解论文
溯源树
样例
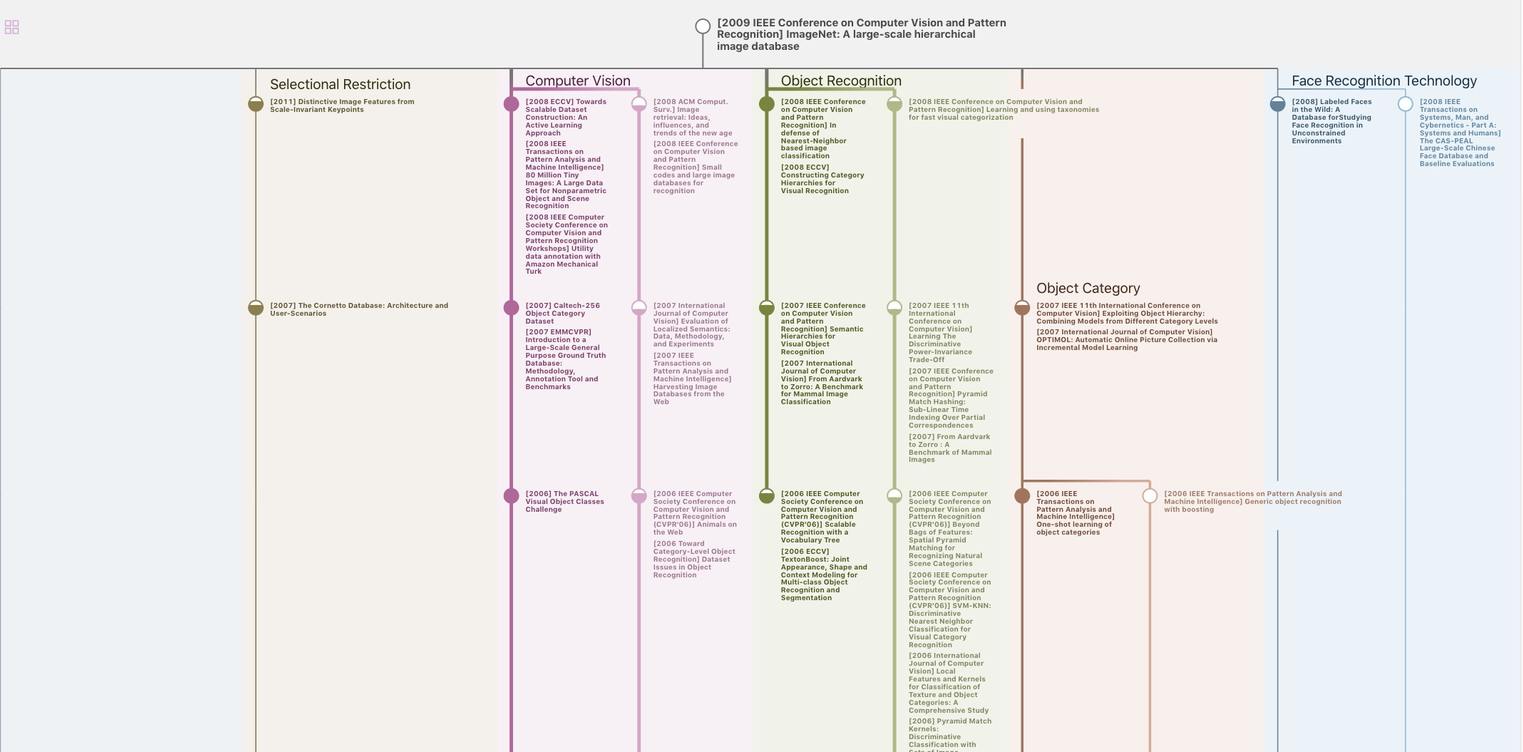
生成溯源树,研究论文发展脉络
Chat Paper
正在生成论文摘要