The Impact of a Popularity Punishing Hyperparameter on ItemKNN Recommendation Performance.
ECIR (2)(2023)
摘要
Collaborative filtering techniques have a tendency to amplify popularity biases present in the training data if no countermeasures are taken. The ItemKNN algorithm with conditional probability-inspired similarity function has a hyperparameter
$$\alpha $$
that allows one to counteract this popularity bias. In this work, we perform a deep dive into the effects of this hyperparameter in both online and offline experiments, with regard to both accuracy metrics and equality of exposure. Our experiments show that the hyperparameter can indeed counteract popularity bias in a dataset. We also find that there exists a trade-off between countering popularity bias and the quality of the recommendations: Reducing popularity bias too much results in a decrease in click-through rate, but some counteracting of popularity bias is required for optimal online performance.
更多查看译文
关键词
Recommendation systems, AB test, Nearest neighbour
AI 理解论文
溯源树
样例
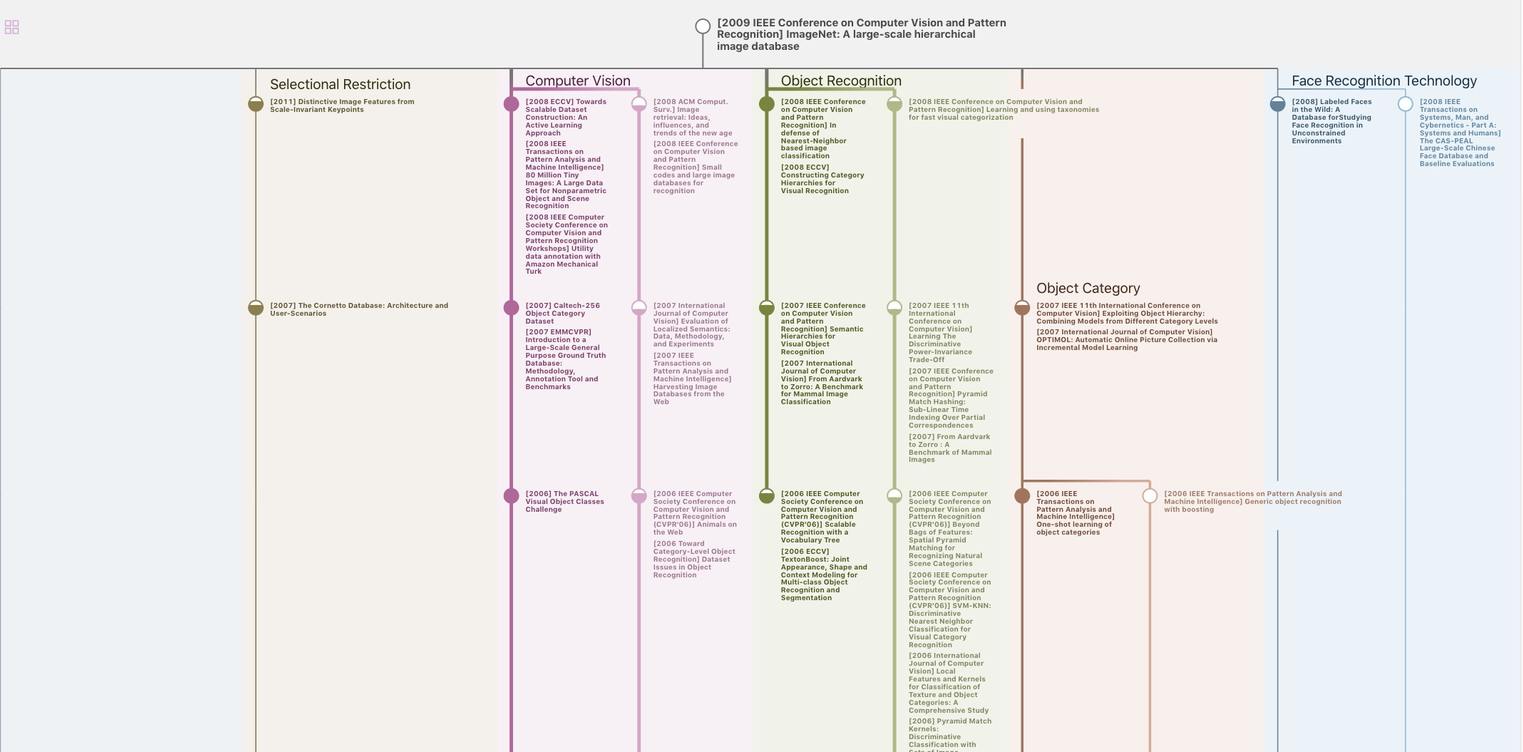
生成溯源树,研究论文发展脉络
Chat Paper
正在生成论文摘要