CLIR: Continual Cross-Lingual Transfer for Low-Resource Information Retrieval.
ECIR (2)(2023)
摘要
This paper proposes a method to train information retrieval (IR) model for a low-resource language with a small corpus and no parallel sentences. Although neural IR models based on pretrained language models (PLMs) have shown high performance in high-resource languages (HRLs), building PLM for LRLs is challenging. We propose C
$$^2$$
LIR, a method to build a high-performing neural IR model for LRL, with dictionary-based pretraining objectives for cross-lingual transfer from HRL. Experiments on the monolingual and cross-lingual IR in diverse low-resource scenarios show the effectiveness and data efficiency of C
$$^2$$
LIR.
更多查看译文
关键词
Low-resource language, Neural IR, Cross-lingual transfer
AI 理解论文
溯源树
样例
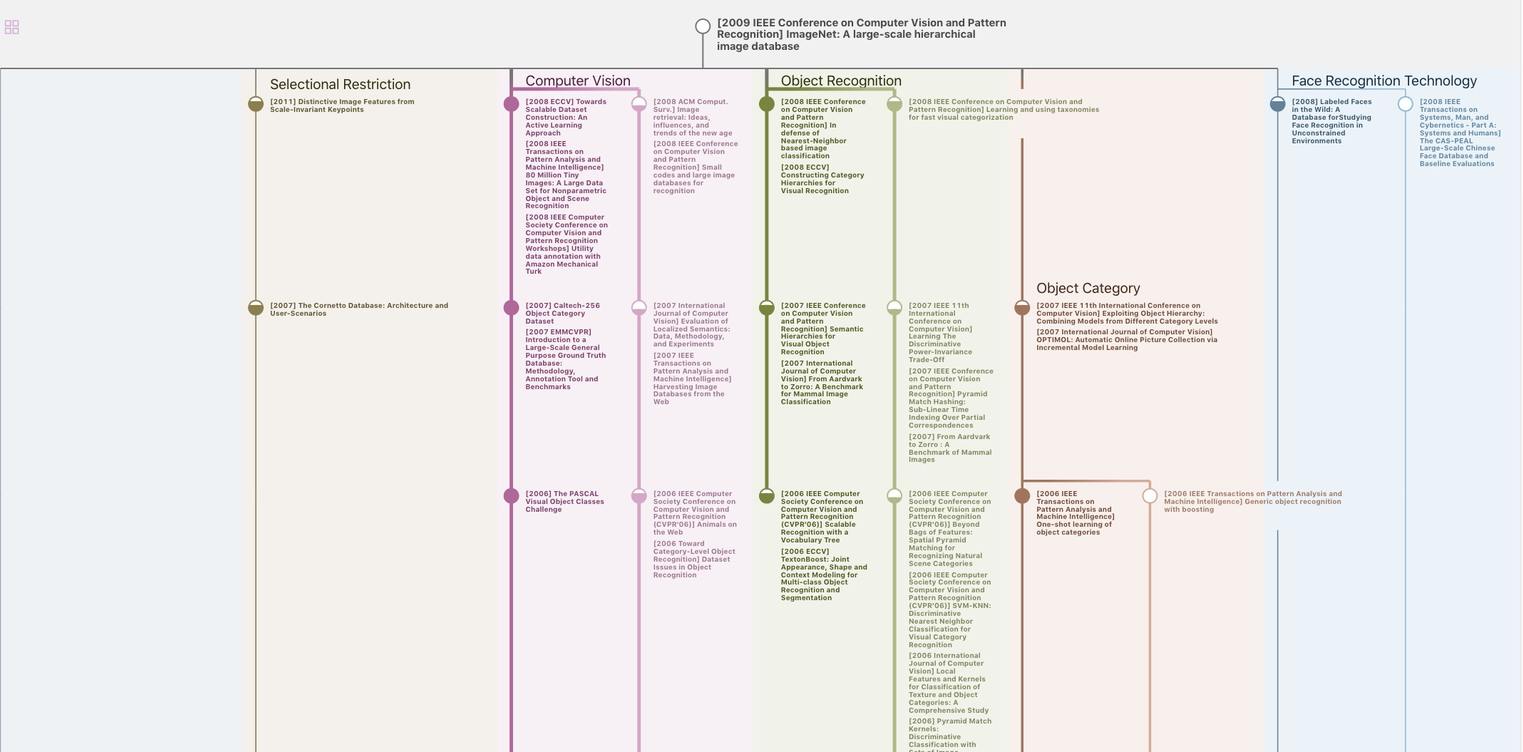
生成溯源树,研究论文发展脉络
Chat Paper
正在生成论文摘要