Feature Differentiation and Fusion for Semantic Text Matching.
ECIR (2)(2023)
摘要
Semantic Text Matching (STM for short) stands for the task of automatically determining the semantic similarity for a pair of texts. It has been widely applied in a variety of downstream tasks, e.g., information retrieval and question answering. The most recent works of STM leverage Pre-trained Language Models (abbr., PLMs) due to their remarkable capacity for representation learning. Accordingly, significant improvements have been achieved. However, our findings show that PLMs fail to capture task-specific features that signal hardly-perceptible changes in semantics. To overcome the issue, we propose a two-channel Feature Differentiation and Fusion network (FDF). It utilizes a PLM-based encoder to extract features separately from the unabridged texts and those abridged by deduplication. On this basis, gated feature fusion and interaction are conducted across the channels to expand text representations with attentive and distinguishable features. Experiments on the benchmarks QQP, MRPC and BQ show that FDF obtains substantial improvements compared to the baselines and outperforms the state-of-the-art STM models.
更多查看译文
关键词
Semantic Text Matching, Deep neural networks, Natural Language Processing
AI 理解论文
溯源树
样例
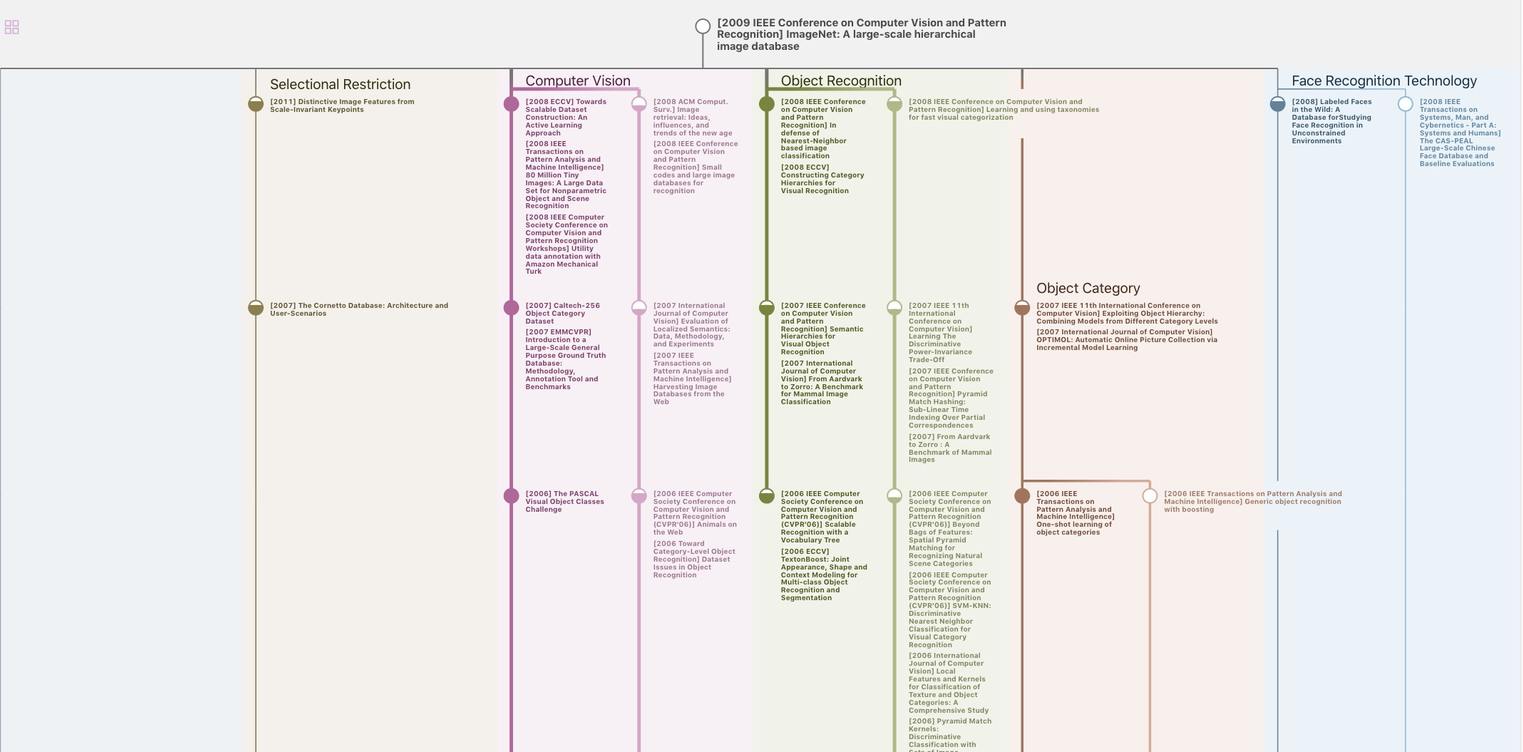
生成溯源树,研究论文发展脉络
Chat Paper
正在生成论文摘要