Unsupervised Learning via Graph Convolutional Network for Stock Trend Prediction.
AINA (1)(2023)
摘要
Stock trend prediction has received a significant amount of attention in recent years. Existing methods could not exploit the peculiar trends for prediction, which are valuable in rising-falling trend analysis for short-term or long-term investments. In this paper, we propose an integrated model that can discover peculiar trend patterns for stock trend prediction. Our proposed model is mainly divided into two parts: the clustering and prediction processes. In the clustering process, we use a Graph Convolutional Network (GCN) model to explore the trend patterns groups from a set of subsequences of time series. In the prediction process, an Long Short-term Memory (LSTM) model will be trained based on the discovered patterns for predicting future stock trends. Experimental results on real-world financial datasets demonstrate that our model yields better performance in terms of stock trend prediction, and outperforms state-of-the-art forecasting models for long-term investment.
更多查看译文
关键词
graph convolutional network,trend,prediction
AI 理解论文
溯源树
样例
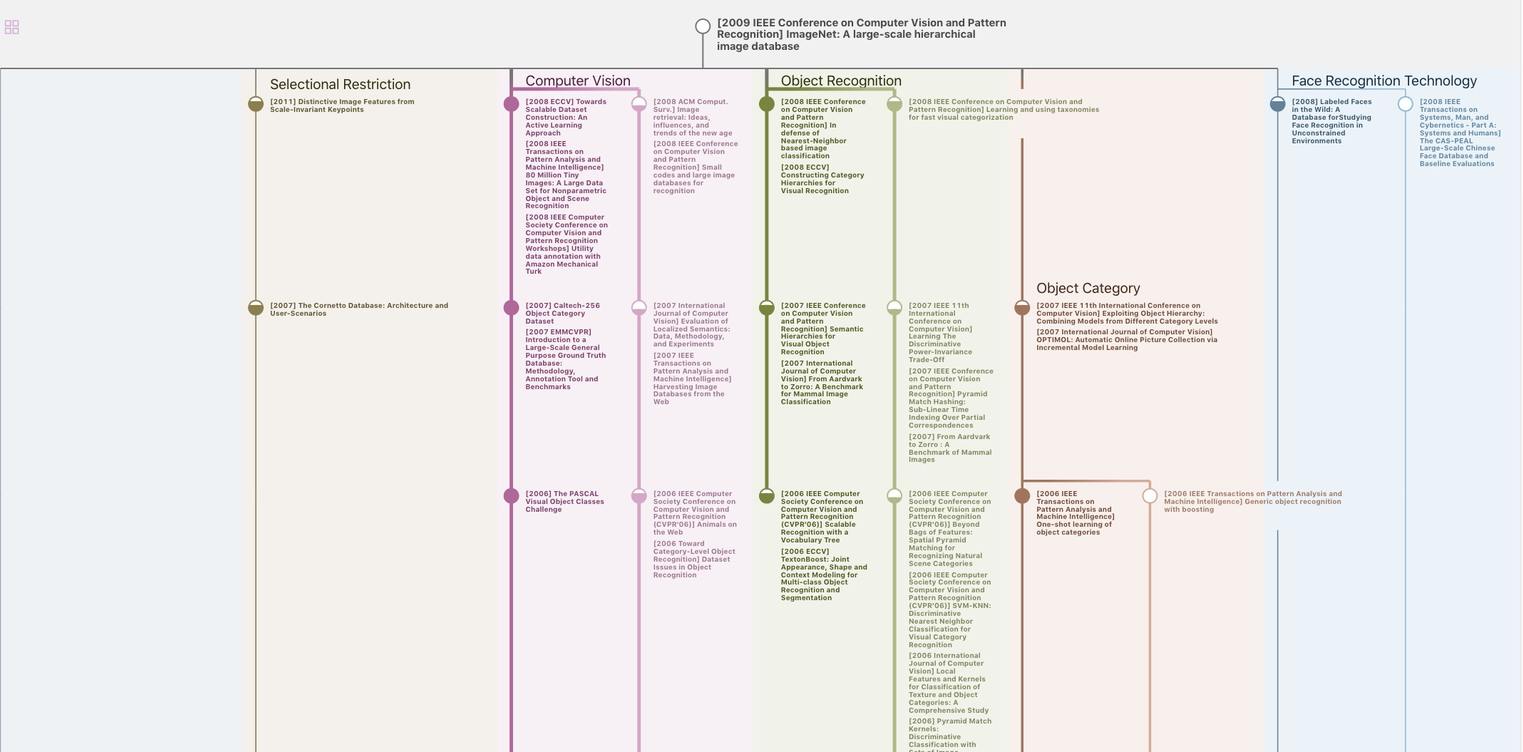
生成溯源树,研究论文发展脉络
Chat Paper
正在生成论文摘要